6 January 2025 to 28 June 2024
¶ My year in music and yours · 6 January 2025 listen/tech
I think about music in years. 2024 was the first year in a long time that I didn't have anything to do with Spotify Wrapped, but that was never why I thought about music in years, and certainly never how. I like my music data to tell me stories about music not about graphic design, and in verifiable numbers not templated snarkiness. I want to stand at the end of one year and the brink of another and think clearly about how I've been listening, and thus living, and expansively about how I might.
You don't have to want what I want, but if you want to experiment with your Spotify listening data, I've built some tools to help you. To play, you need a computer and a Spotify premium account, and you need to do two things. One is this:
- go to your Spotify Account page from the popup menu on your profile picture
- scroll down to the evasively named "Account privacy" link and click it
- scroll down the Account privacy page to the "Download your data" section
- check the box on the right under "Extended streaming history", and then the Request Data button at the bottom
While you wait for your data, which will take a couple days, do the other thing, which is to go to Curio, my web thing for collating music-data curiosity, and follow the instructions for getting a free Spotify API key. If you've already done that, just wait impatiently.
When you get your data files from Spotify, go to the History page in Curio and follow the instructions there to load your streaming history. (If the file names in the instructions don't match the files you got, you got the wrong data so go back and request the Extended streaming history like I said.)
You can ask your own questions about your data, but to get started I've already set up a bunch of views that I wanted for myself. Here is a mercilessly geeky tour of those, in which you will learn way more than you ever wanted to know about my year in music.
overview
I listen to music a lot, but never strictly as background, so not for as much time as I could physically. Apparently I went 17 whole days last year without playing any, which I admit is alarming negligence. The count under "tracks" is the strict computer version of counting in which listening to a single and then the exact same song on the album counts as 2 different things. The number under "songs" tries to account for this, although Spotify doesn't give us public access to their internal audio fingerprints, so I just do this by counting unique artist-id/song-title pairs. The "albums" number only counts the albums where I played at least two of their actual tracks (so not separate singles), although it doesn't matter whether they were played from the album page or from playlists. The "artists" number counts only the primary artists of each track. The "genres" number counts only the genres where I listened to stuff by at least 5 different artists. The "of" link at the end tells you that I had 22,423 total streams last year, and you can click your count to see yours, because that's how it should be with data.
tracks
To me a "year in music" is the music that was released in a year. I listen mostly to new music, and all the personal 2024 stats I personally want to see are about my 2024 listening to 2024 music. But there's a "new releases"/"all releases" switcher at the top of most of these History views in Curio, and a separate "old releases" option for a few of them, in case you feel differently. The ranking of tracks is done with a points calculation that you can see for yourself by going to the "see the query for this" link below the table, but my philosophical position is that playing a song on loop for a while is better evidence of affection than playing it once, but not better evidence than returning to it deliberately over the course of different days, so I give each song the sum of 4th roots of its daily playcounts. (Why 4th roots? Square roots didn't mute the looping effect enough for my taste, and chaining two square-roots together worked enough better than I didn't bother adding a cube-root fucntion to the query-language yet. (Although there's also a way to do arbitrary math, so you could raise it to the power of a third, but let's not.)) I don't tend to repeat individual songs very much, so this view isn't my favorite way to look at my own history, but even so it's useful for cross-checking the playlist view that we'll get to at the end.
albums
I mostly listen via weekly playlists of new releases, usually with only one track per artist, and in the increasingly common case of waterfall releases where most of the tracks on an album are released individually before the whole album, it's common that I have listened to most of the contents of an album without directly playing the album much or at all. All of these, though, are cases where I cared enough to break my playlist pattern and play these whole albums. "ms_played" means milliseconds, and is the standard Spotify measurement of how much clock time you spent listening to a song independent of its duration, so if you end up doing your own queries you'll quickly get familiar with this. The query here does some fun stuff with song titles and release types to try to report the album/single dynamics of my listening, but it won't surprise me much if your listening has some edge cases I didn't catch in mine.
artists
But really, my levels of attachment to music are, and have been for years, mostly artist and genre. Humans, individually and collectively. The track and album lists are, for me, steps on the way to this artist list. At the artist level, too, I am leery of overweighting isolated looping, so my artist point system sums up the square roots of the number of unique tracks by that artist played on each listening day. The "count" column here is the total number of unique new 2024 songs I played by each artist. This list is a pretty good telling of my 2024 music story to myself. I expect the chance is low that anybody other than me knows this specific combination of specific artists well enough to make sense of it. First to Eleven is a prolific pop-punk covers band who put out new songs every week or two. TERA is a strange home-demo-sounding vocaloid project that has survived me mostly rotating out of vocaloid music because their use of artificial voices is so artificial. Phyllomedusa is frog-themed grindcore. Sifaeli Mwabuka is a Swahili gospel singer from Tanzania, and I could not have told you his name and am probably as surprised as you that I spent more than four hours listening to him, but apparently he is what happens when you try to listen to both maskandi and Sepedi wedding music despite not really knowing much about either. But mainly what this tells you, as you already knew if you read my book or ever talked to me about music for longer than five minutes, is that I love Nightwish and they had a new album this year.
popularity
My favorite one-time thing I ever did for Spotify Wrapped was the Listening Personality, in 2022. It wasn't supposed to be a one-time virality-nudge, it was supposed to be the seed of a way for humans and algorithms to talk to each other about the aspects of music-preference that are independent of what kind of music you like. Spotify, sadly, was not as interested as I was in what people actually want, nor about using algorithms to amplify curiosity instead of, say, maximizing marginal revenue, so I never got to do more with the Listening Personality codes beyond showing them to people once, but at least I obstinately insisted on Wrapped displaying the four-letter Myers-Briggs-esque codes I devised for each listener, over the graphics team's predictable attempt to reduce the 16 combinations to unexplained tarot cards. (But don't worry, they got their tarot cards the next year.)
I can't exactly reproduce the Listening Personality with just our own listening histories, because the calibration of the polarities of each axis was done holistically across the whole Spotify population. But if we lose collective breadth, we gain individual depth. Instead of a single letter-pair encoding a single score representing how popular the music you tend to listen to tends to be, here we have a heatmap in which the X-axis is your level of artist attachment (scaled to 100 for your most-played artist) and the Y-axis is those artists' global popularities (scaled to 100 for Taylor). What my heatmap tells us is that I listen very broadly to a lot of artists who are not very popular but usually are also not entirely unknown. And I also do like Taylor. If you only listen to Taylor, your heatmap will be a single red cell in the top left with a number of which I assume you will be very proud.
diversity
Two of the other three dimensions of the Listening Personality had to do with how your listening is distributed or concentrated across artists and across songs. I am a fairly extreme outlier in the direction of variety in both, which we see here as a small explosion centered in the bottom (more artists) right (more songs). If you spent the year cycling through Taylor's Versions of everything, you would end up in the top left; if just covers of "Anti-Hero", a red line down the right edge. The axes here are not normalized, because I no longer have access to everybody else's data to figure out a generalized granularity, so your own version of this view may be comically small or awkwardly large.
genres
The Wrapped story I worked on every year (although one year they substituted something else without having the guts to tell me they were going to) was the list of your Top Genres. I have taken some shit over the years for occasionally making up genre names, but if you want to know what the point of that was, there's a whole chapter about it in my book, and after seeing the 2024 Wrapped in which Spotify ditched the whole concept of genres as human musical community and generated idiotic random phrases instead, I feel 100% comfortable with what I did. Every year as we started making plans for Wrapped I said the same three things: 1) I will do the genre analysis so it's right, because these are real communities of artists and listeners in the real world and they deserve to see themselves. 2) Let's show people all their genres instead of just five. 3) And let's also let people see which artists (both in general and that you like) go with each genre, so the whole thing isn't mysterious. And every year the "creative" team said something like "Or what if we show the same unexplained list of five, but as a spinning hamburger made of radioactive sludge?"
Curio's version is obdurately sparkleless but sludgeless: all your genres, ranked with artisanally hand-tuned scoring, and clickable in your version to see exactly which of your artists make up each list.
words
My favorite story I proposed for Wrapped, knowing full-well that it would never get approved, ironically did involve generating random phrases for people, but the way I did it was from the words in the titles of the songs you played, so even this totally frivolous thing was explainable and there was a sense in which it could be correct. The key to getting a short list of pertinent words is having the whole population's listening to work with, so you can give each person the few words that most distinctively characterize their listening. Without that global data I am forced to give you lots of words instead of a few, in a weighted shuffle to avoid everybody's saying love death soundtrack day, but hopefully yours, like mine, will including something true and vulgar that will make you smile and would make lawyers panic.
durations
People talk about pop songs and attention spans both getting shorter, and we have all those milliseconds, so this one is a view of your listening (by month, for fun, across the top) distributed by how long you listened to each track (in minutes, down the left). I listen to a lot of three-minute songs. I listen to a few seconds of a lot of songs and then skip. I listen to some long songs. Apparently in October I was working on getting the track-scan timings right in Curio.
daymap
Everything you play on a streaming service is timestamped. In Spotify's case the timestamps are in UTC, so I included a timeshift option to allow you to bump them into your local time, or adjust your listening day some other way if you prefer. I'm not going to include my daymap, here, because it shows you every timestamp from the entire year and I realize looking at it that immediately reveals exactly when I traveled to a different time zone this year, and maybe that's more sharing than I need.
weekmap
Musically speaking, though, the view by hour and weekday is more relevant. Spotify spent a lot of energy, over the years, trying to believe that most people's listening is heavily determined by day and time, and if yours is, now you have a playlist feature for that in Spotify. Mine isn't. I make a playlist of new songs I want to hear every Friday, and then I cycle through it, gradually deleting songs I don't end up enjoying, for the rest of the week. Thus there is absolutely no sense in which a have different music I prefer on Tuesday afternoon vs Thursday morning. What I do have, as you see here, is a very distinct pattern of doing a lot of rapid new-song scanning while eating my lunch on Fridays. Also, hopefully I do not have any important online accounts for which the security question is "At what hour are you most likely to be asleep?"
release years
The fourth dimension in the Listening Personality was newness. I know, from data, that I am, or at least for a few years provably was, one of the handful of people in the world whose listening is most intently focused on new music. Curio thus has two different ways of looking at this particular quirk. This first one shows release years by listening months, so you can see how I listen to songs from the previous year for at least the first week of January, and how in May last year I tried and ultimately failed to find or remember one particular Ray Charles song.
song ages
My listening pattern is even clearer in this view by song age, with ascending resolution. Friday is new-release day, but I am often distracted from listening over the weekends, so Monday through Thursday I am most heavily playing the songs from the previous Friday.
poster
As you may already realize, my taste in visual design runs to the Tuftean. Don't make me justify the horizonal rule in this poster view to ET, but the order and sizes and colors here are all data-driven, and yet it's still faintly reminiscent of a concert-poster design.
10x10 grid
5x5 grid
banner
If I want a visual summary of my year in music, it should definitely be made out of the visual art that is already attached to the music, not something else.
playlist
open this playlist in Spotify
The playlist, at least for me, is the authoritative final summary. It's my year in music, so it better be made of music, and it better make sense to me when I play it.
Spotify makes you a playlist, too, called Your Top Songs. It's fine, in that so far they haven't decided to screw it up with any kind of machine learning. It's never what I want, personally, because it ignores release dates and it has mutiple songs per artist and it doesn't sort the way I want.
What I want, and thus built, is not a Top Songs playlist, but an attempt at a playlist that represents a listening year. To do this it starts from the artist list, not song list, and then tries to pick your most-played new song from each of those artists.
Here's what that looks like in Dactal query syntax, as you can see if you click the "see the query for this" link at the bottom of the playlist table, except I've added a little color-coding:
The red parts are the artist query, which goes like this:
- get all your 2024 tracks (from a previous query)
- group these by each track's first artist, song name and date
- assign the square root of the number of times you played that artist/song that day as songdatepoints
- group those artist/song/date groups by artist and song
- total the songdatepoints from all dates per artist/song
- also total the amount of time you played this artist/song
- sort and rank the artist/song groups by songpoints and then songtime (in both cases from larger to smaller)
- group those artist/song groups by artist
- assign each artist a total artistpoints value that is the sum of songpoints-.5 (to reduce the weight of lots of songs you only played once)
- total up the amount of time you listened to that artist
The playlist query inserts one more line (an annotation suboperation) to calculate each artist's topsong for the playlist by taking the artist's highest-ranking artist/song group and getting an album track if one is available from the potentially multiple tracks representing that song, and the one you streamed first otherwise.
The artist query already sorts the artists by artistpoints and then listening time, which is the same order I wanted for the playlist. The :@<=100 picks the first 100, and the final block in parentheses just constructs some nicer columns for the final view. Curio watches for query results with uri columns, and offers to make them into a playlist for you, as you'll see.
The other thing I always wanted in Wrapped and never got, however, is the ability to remove things. Sometimes it's technically true that you played a song a lot, but not emotionally true that you liked it. Or, in my case, it's technically true that the reissue of Ultravox's Lament is a 2024 release, but I don't consider new repackagings of old recordings of "Dancing With Tears in My Eyes" to be new songs, so I prefer to remove that one. The chaining nature of Dactal makes this easy. That final sorting/filtering line just becomes
with the limit filter still at the end so we get 100 tracks instead of 99 despite dropping one. It would also be possible to filter Ultravox out at the artist level, or Lament out at the album level, but it doesn't make any difference to the results.
That's all what Curio gives you, including deleting tracks:
But this still isn't quite what I want, and I am stubborn. I make playlists every week, during the year, and these sometimes represent my decisions, after a week of listening to more than one track from an album, which one to keep. And it's not always the one I played the most times. Curio already has this playlist data, or can have it, if you provide an indexing pattern at the bottom of the Playlists page. This can be as simple as * to index everything, but I have lots of playlists that I made for reasons other than my taste, so I only index these two particular sets:
(End partial matches with asterisks, and separate multiple patterns with a space, so the commas here are part of the matching patterns.)
So my own personal variation adds one more wrinkle to the artist/song-group sorting:
The songkey line computes an artist/song key-string, which the s2pa line then uses to navigate into another dataset, itself produced by a query, that indexes playlist appearances by those same artist/song keys. I could use this dataset join to sort tracks by playlist counts or dates or anything, but all I really want to do is prefer tracks that I put on a playlist to ones I didn't, if there's a choice. So the .(1) part results in a 1 for songkeys that are in the songkey to playlist apperances dataset, and nothing for songkeys that aren't, and Dactal always sorts something before nothing.
There is absolutely no way that you could possibly tell the difference between me doing this and not, nor any reason you should care, but I care. What you choose to care about, in your own data and life, is your business, but the moral function of software is to let us express our care, and thus to encourage us to realize we have it. Your data is yours. The stories it tells are your stories. You should want them to be right, and nobody but you should get to tell you what that right is.
You don't have to care, but I want you to. The more we all care about everything, the less we will tolerate it any of it being bad.
You don't have to want what I want, but if you want to experiment with your Spotify listening data, I've built some tools to help you. To play, you need a computer and a Spotify premium account, and you need to do two things. One is this:
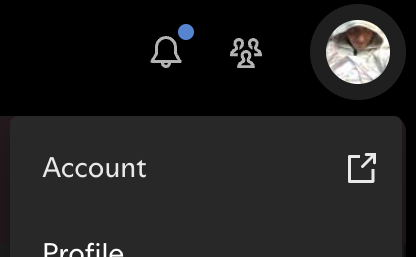
- scroll down to the evasively named "Account privacy" link and click it
- scroll down the Account privacy page to the "Download your data" section
- check the box on the right under "Extended streaming history", and then the Request Data button at the bottom
While you wait for your data, which will take a couple days, do the other thing, which is to go to Curio, my web thing for collating music-data curiosity, and follow the instructions for getting a free Spotify API key. If you've already done that, just wait impatiently.
When you get your data files from Spotify, go to the History page in Curio and follow the instructions there to load your streaming history. (If the file names in the instructions don't match the files you got, you got the wrong data so go back and request the Extended streaming history like I said.)
You can ask your own questions about your data, but to get started I've already set up a bunch of views that I wanted for myself. Here is a mercilessly geeky tour of those, in which you will learn way more than you ever wanted to know about my year in music.
overview

I listen to music a lot, but never strictly as background, so not for as much time as I could physically. Apparently I went 17 whole days last year without playing any, which I admit is alarming negligence. The count under "tracks" is the strict computer version of counting in which listening to a single and then the exact same song on the album counts as 2 different things. The number under "songs" tries to account for this, although Spotify doesn't give us public access to their internal audio fingerprints, so I just do this by counting unique artist-id/song-title pairs. The "albums" number only counts the albums where I played at least two of their actual tracks (so not separate singles), although it doesn't matter whether they were played from the album page or from playlists. The "artists" number counts only the primary artists of each track. The "genres" number counts only the genres where I listened to stuff by at least 5 different artists. The "of" link at the end tells you that I had 22,423 total streams last year, and you can click your count to see yours, because that's how it should be with data.
tracks
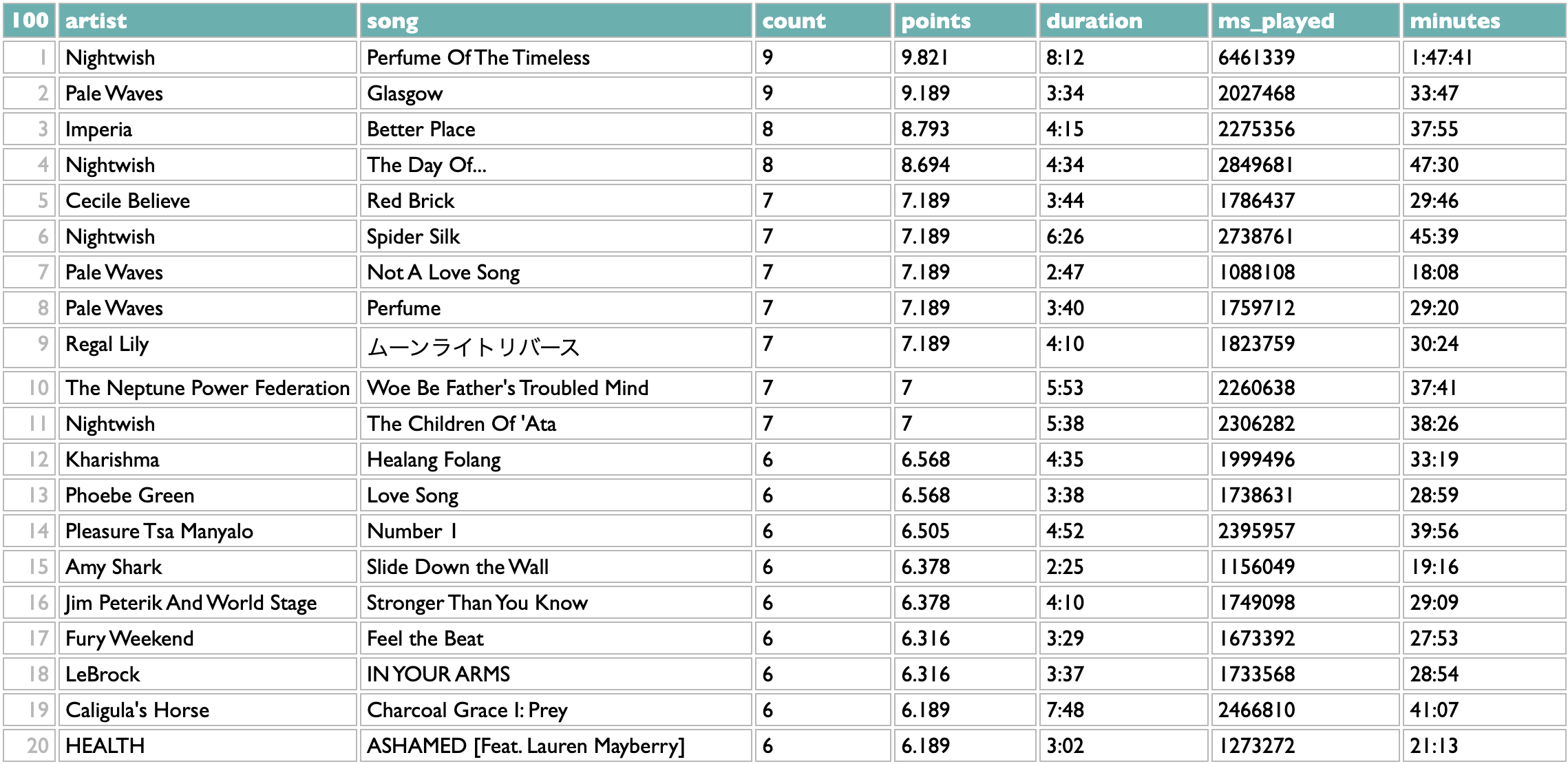
To me a "year in music" is the music that was released in a year. I listen mostly to new music, and all the personal 2024 stats I personally want to see are about my 2024 listening to 2024 music. But there's a "new releases"/"all releases" switcher at the top of most of these History views in Curio, and a separate "old releases" option for a few of them, in case you feel differently. The ranking of tracks is done with a points calculation that you can see for yourself by going to the "see the query for this" link below the table, but my philosophical position is that playing a song on loop for a while is better evidence of affection than playing it once, but not better evidence than returning to it deliberately over the course of different days, so I give each song the sum of 4th roots of its daily playcounts. (Why 4th roots? Square roots didn't mute the looping effect enough for my taste, and chaining two square-roots together worked enough better than I didn't bother adding a cube-root fucntion to the query-language yet. (Although there's also a way to do arbitrary math, so you could raise it to the power of a third, but let's not.)) I don't tend to repeat individual songs very much, so this view isn't my favorite way to look at my own history, but even so it's useful for cross-checking the playlist view that we'll get to at the end.
albums
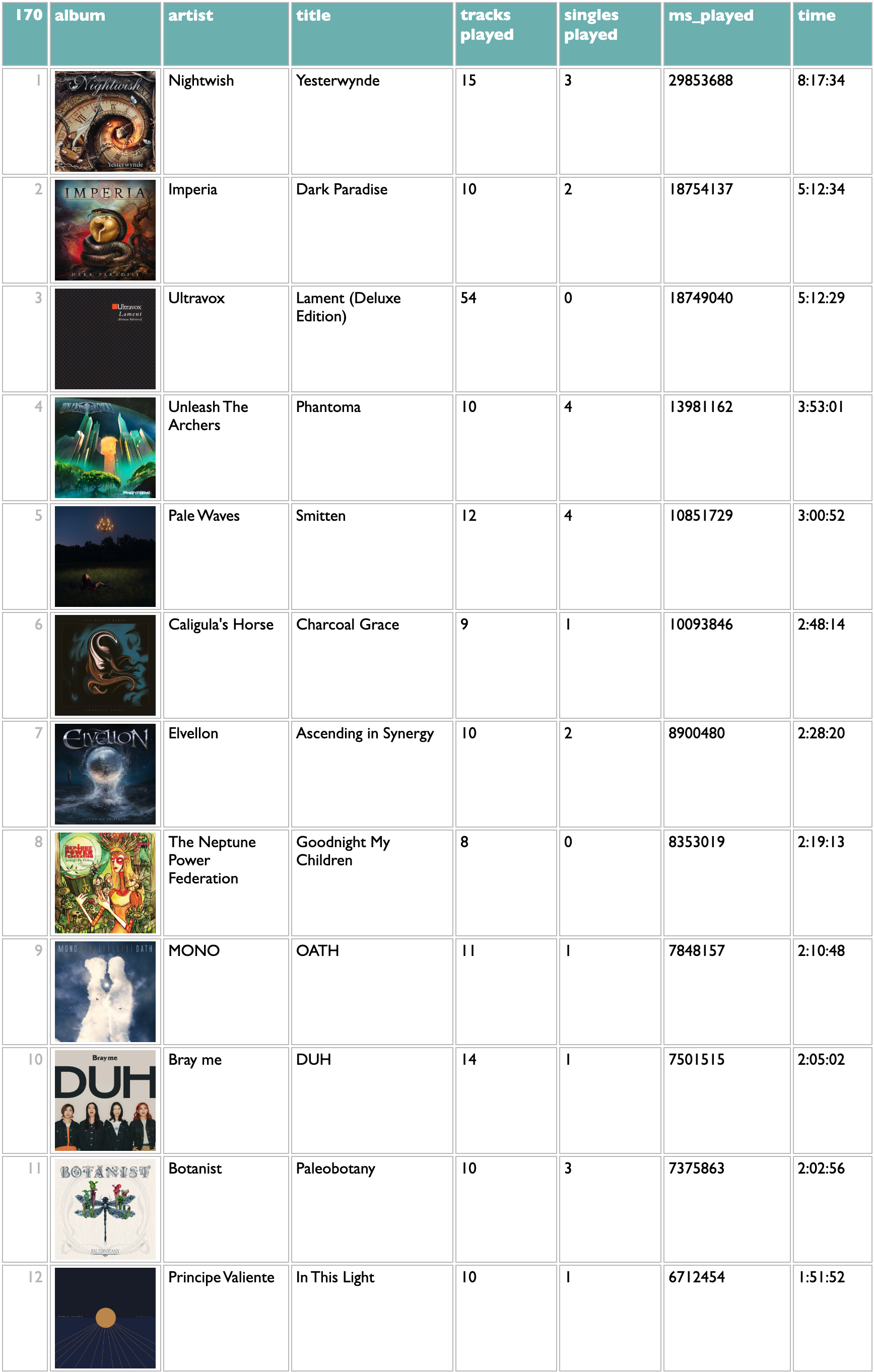
I mostly listen via weekly playlists of new releases, usually with only one track per artist, and in the increasingly common case of waterfall releases where most of the tracks on an album are released individually before the whole album, it's common that I have listened to most of the contents of an album without directly playing the album much or at all. All of these, though, are cases where I cared enough to break my playlist pattern and play these whole albums. "ms_played" means milliseconds, and is the standard Spotify measurement of how much clock time you spent listening to a song independent of its duration, so if you end up doing your own queries you'll quickly get familiar with this. The query here does some fun stuff with song titles and release types to try to report the album/single dynamics of my listening, but it won't surprise me much if your listening has some edge cases I didn't catch in mine.
artists
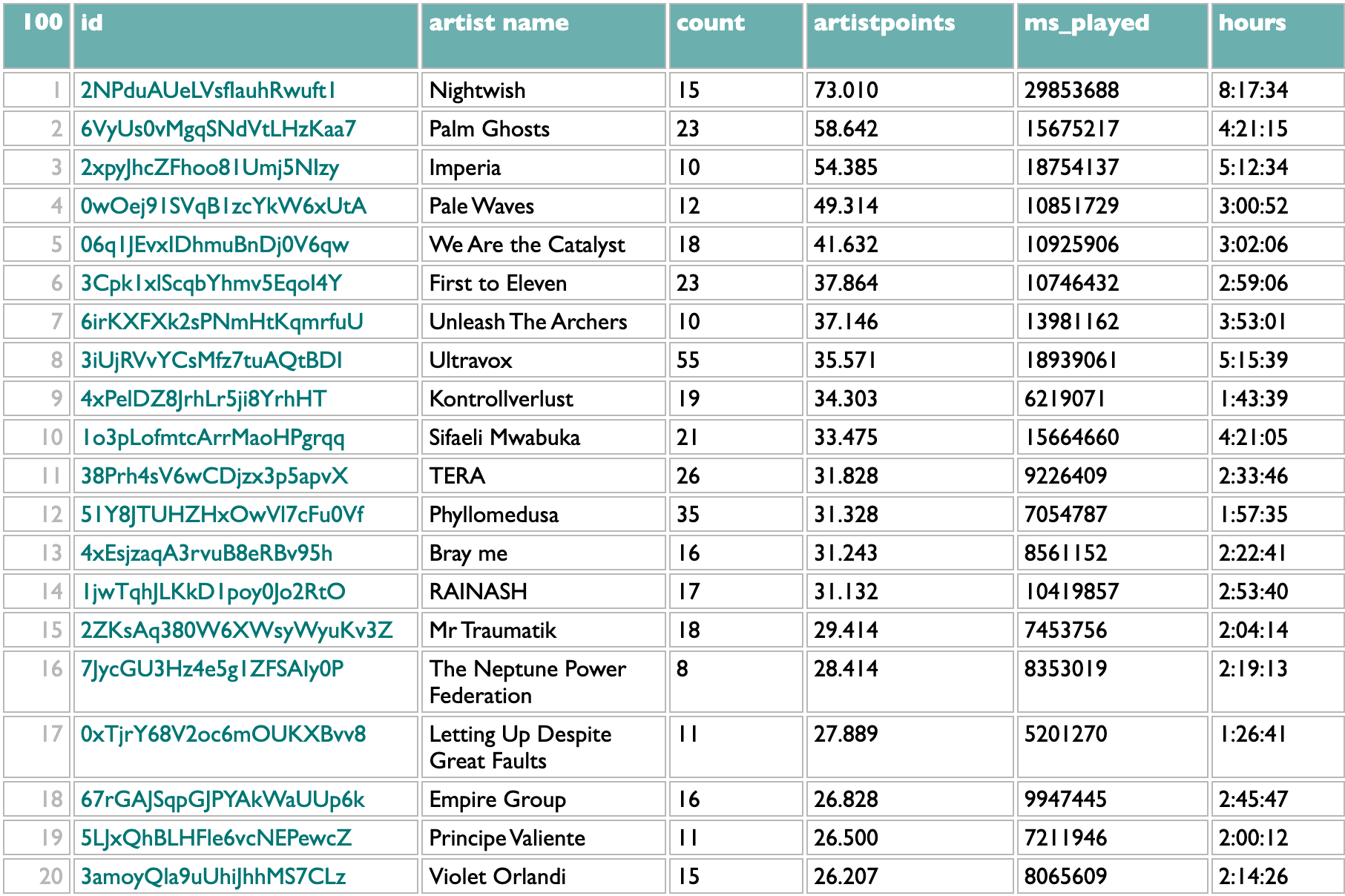
But really, my levels of attachment to music are, and have been for years, mostly artist and genre. Humans, individually and collectively. The track and album lists are, for me, steps on the way to this artist list. At the artist level, too, I am leery of overweighting isolated looping, so my artist point system sums up the square roots of the number of unique tracks by that artist played on each listening day. The "count" column here is the total number of unique new 2024 songs I played by each artist. This list is a pretty good telling of my 2024 music story to myself. I expect the chance is low that anybody other than me knows this specific combination of specific artists well enough to make sense of it. First to Eleven is a prolific pop-punk covers band who put out new songs every week or two. TERA is a strange home-demo-sounding vocaloid project that has survived me mostly rotating out of vocaloid music because their use of artificial voices is so artificial. Phyllomedusa is frog-themed grindcore. Sifaeli Mwabuka is a Swahili gospel singer from Tanzania, and I could not have told you his name and am probably as surprised as you that I spent more than four hours listening to him, but apparently he is what happens when you try to listen to both maskandi and Sepedi wedding music despite not really knowing much about either. But mainly what this tells you, as you already knew if you read my book or ever talked to me about music for longer than five minutes, is that I love Nightwish and they had a new album this year.
popularity
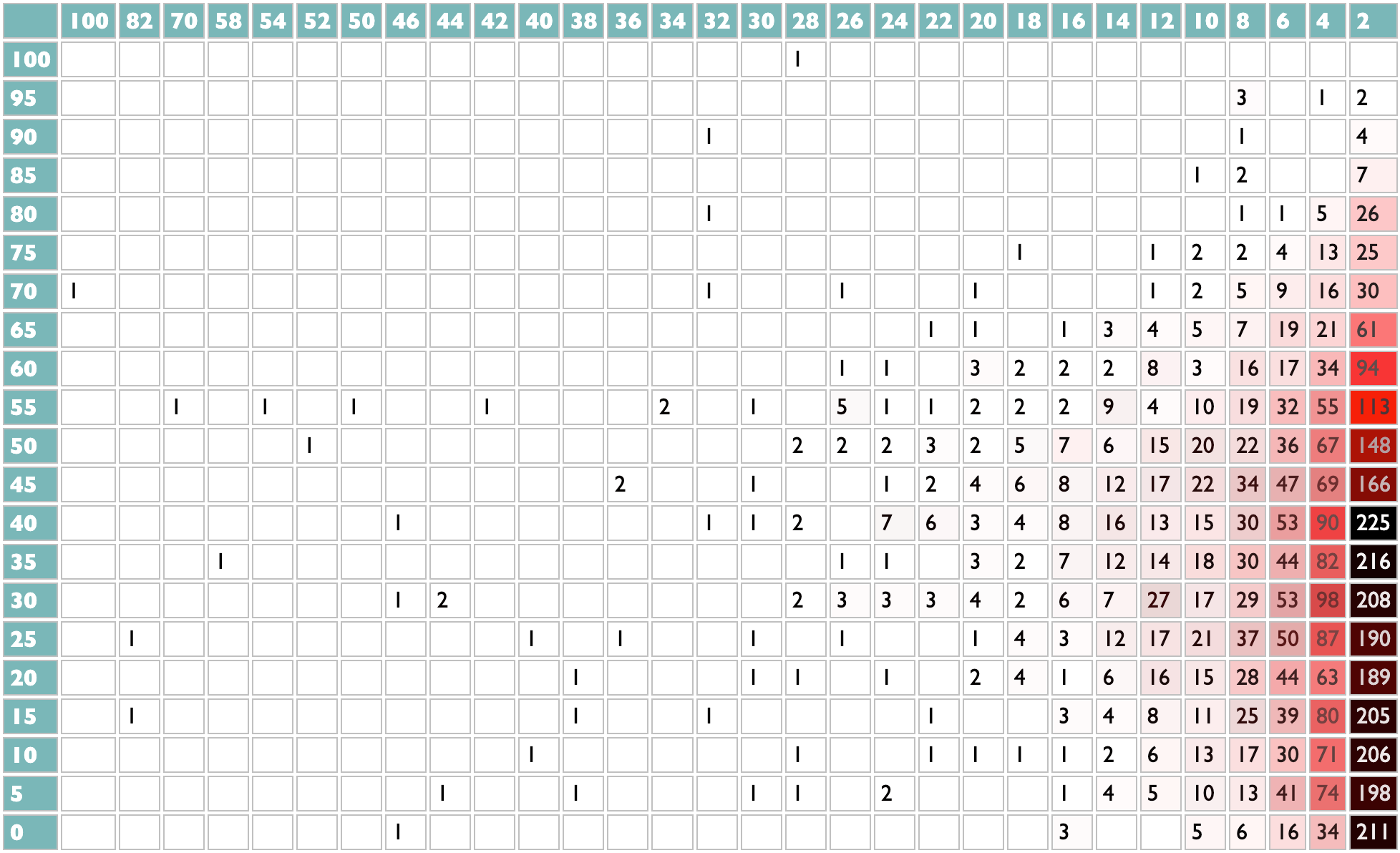
My favorite one-time thing I ever did for Spotify Wrapped was the Listening Personality, in 2022. It wasn't supposed to be a one-time virality-nudge, it was supposed to be the seed of a way for humans and algorithms to talk to each other about the aspects of music-preference that are independent of what kind of music you like. Spotify, sadly, was not as interested as I was in what people actually want, nor about using algorithms to amplify curiosity instead of, say, maximizing marginal revenue, so I never got to do more with the Listening Personality codes beyond showing them to people once, but at least I obstinately insisted on Wrapped displaying the four-letter Myers-Briggs-esque codes I devised for each listener, over the graphics team's predictable attempt to reduce the 16 combinations to unexplained tarot cards. (But don't worry, they got their tarot cards the next year.)
I can't exactly reproduce the Listening Personality with just our own listening histories, because the calibration of the polarities of each axis was done holistically across the whole Spotify population. But if we lose collective breadth, we gain individual depth. Instead of a single letter-pair encoding a single score representing how popular the music you tend to listen to tends to be, here we have a heatmap in which the X-axis is your level of artist attachment (scaled to 100 for your most-played artist) and the Y-axis is those artists' global popularities (scaled to 100 for Taylor). What my heatmap tells us is that I listen very broadly to a lot of artists who are not very popular but usually are also not entirely unknown. And I also do like Taylor. If you only listen to Taylor, your heatmap will be a single red cell in the top left with a number of which I assume you will be very proud.
diversity
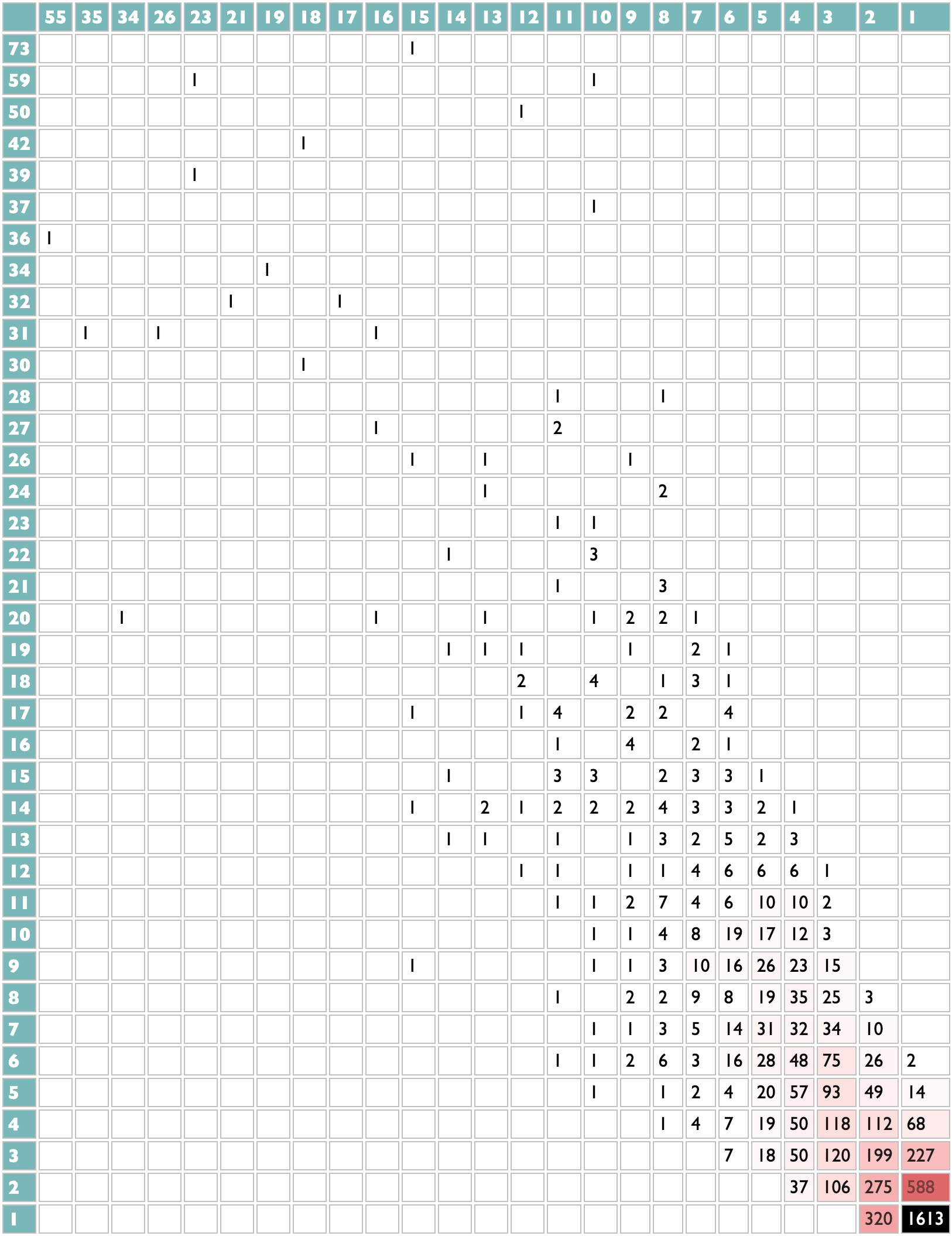
Two of the other three dimensions of the Listening Personality had to do with how your listening is distributed or concentrated across artists and across songs. I am a fairly extreme outlier in the direction of variety in both, which we see here as a small explosion centered in the bottom (more artists) right (more songs). If you spent the year cycling through Taylor's Versions of everything, you would end up in the top left; if just covers of "Anti-Hero", a red line down the right edge. The axes here are not normalized, because I no longer have access to everybody else's data to figure out a generalized granularity, so your own version of this view may be comically small or awkwardly large.
genres
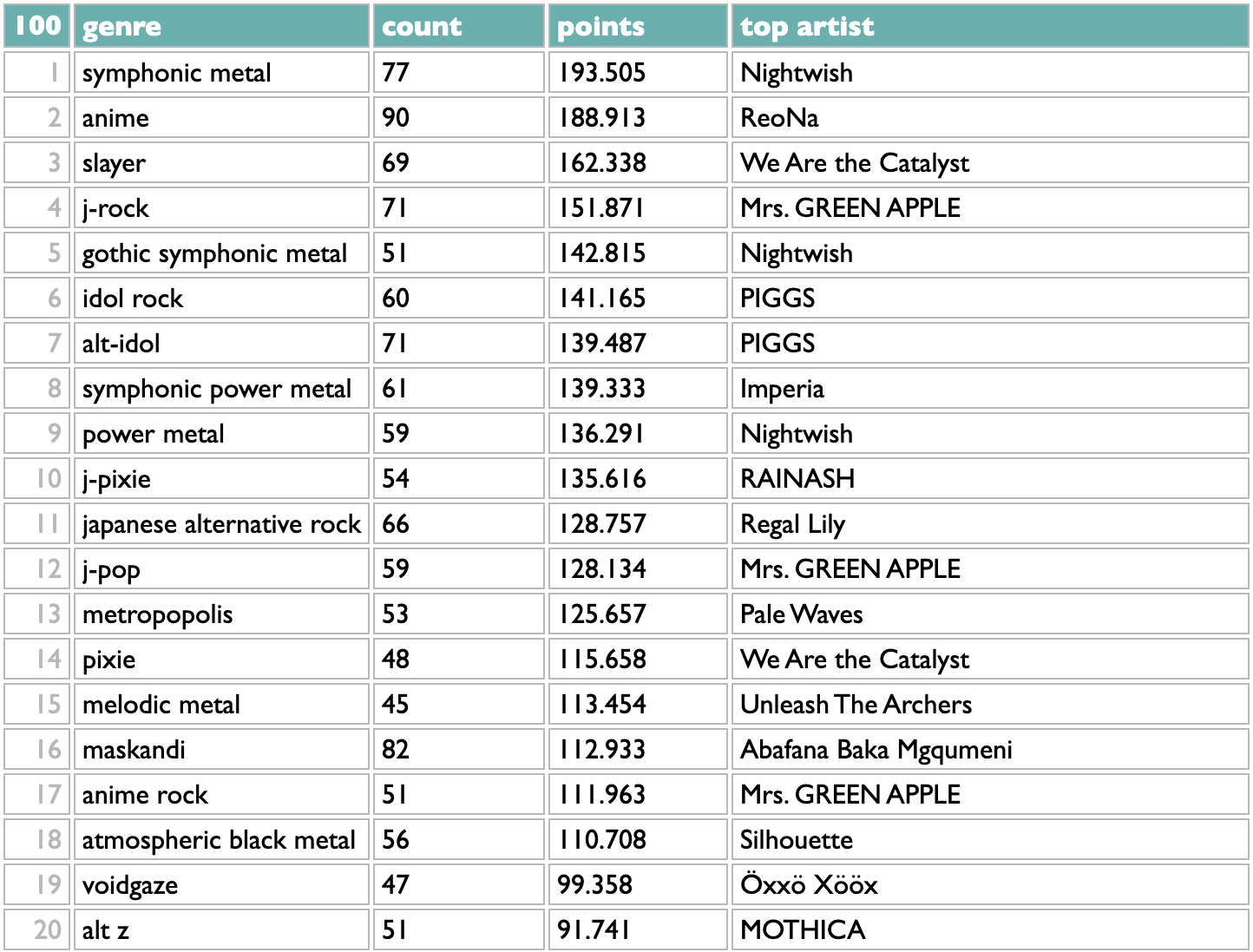
The Wrapped story I worked on every year (although one year they substituted something else without having the guts to tell me they were going to) was the list of your Top Genres. I have taken some shit over the years for occasionally making up genre names, but if you want to know what the point of that was, there's a whole chapter about it in my book, and after seeing the 2024 Wrapped in which Spotify ditched the whole concept of genres as human musical community and generated idiotic random phrases instead, I feel 100% comfortable with what I did. Every year as we started making plans for Wrapped I said the same three things: 1) I will do the genre analysis so it's right, because these are real communities of artists and listeners in the real world and they deserve to see themselves. 2) Let's show people all their genres instead of just five. 3) And let's also let people see which artists (both in general and that you like) go with each genre, so the whole thing isn't mysterious. And every year the "creative" team said something like "Or what if we show the same unexplained list of five, but as a spinning hamburger made of radioactive sludge?"
Curio's version is obdurately sparkleless but sludgeless: all your genres, ranked with artisanally hand-tuned scoring, and clickable in your version to see exactly which of your artists make up each list.
words

My favorite story I proposed for Wrapped, knowing full-well that it would never get approved, ironically did involve generating random phrases for people, but the way I did it was from the words in the titles of the songs you played, so even this totally frivolous thing was explainable and there was a sense in which it could be correct. The key to getting a short list of pertinent words is having the whole population's listening to work with, so you can give each person the few words that most distinctively characterize their listening. Without that global data I am forced to give you lots of words instead of a few, in a weighted shuffle to avoid everybody's saying love death soundtrack day, but hopefully yours, like mine, will including something true and vulgar that will make you smile and would make lawyers panic.
durations
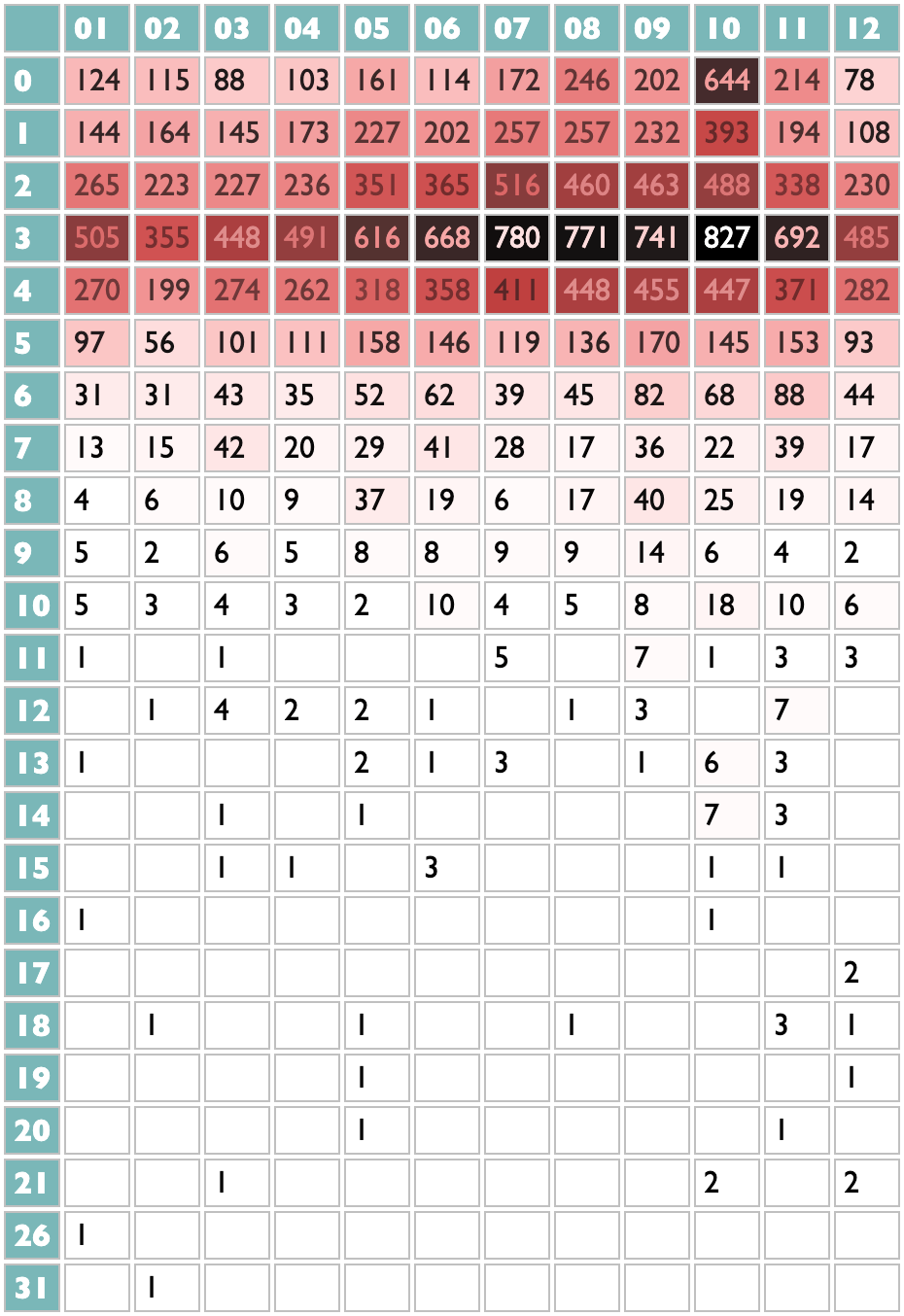
People talk about pop songs and attention spans both getting shorter, and we have all those milliseconds, so this one is a view of your listening (by month, for fun, across the top) distributed by how long you listened to each track (in minutes, down the left). I listen to a lot of three-minute songs. I listen to a few seconds of a lot of songs and then skip. I listen to some long songs. Apparently in October I was working on getting the track-scan timings right in Curio.
daymap
Everything you play on a streaming service is timestamped. In Spotify's case the timestamps are in UTC, so I included a timeshift option to allow you to bump them into your local time, or adjust your listening day some other way if you prefer. I'm not going to include my daymap, here, because it shows you every timestamp from the entire year and I realize looking at it that immediately reveals exactly when I traveled to a different time zone this year, and maybe that's more sharing than I need.
weekmap
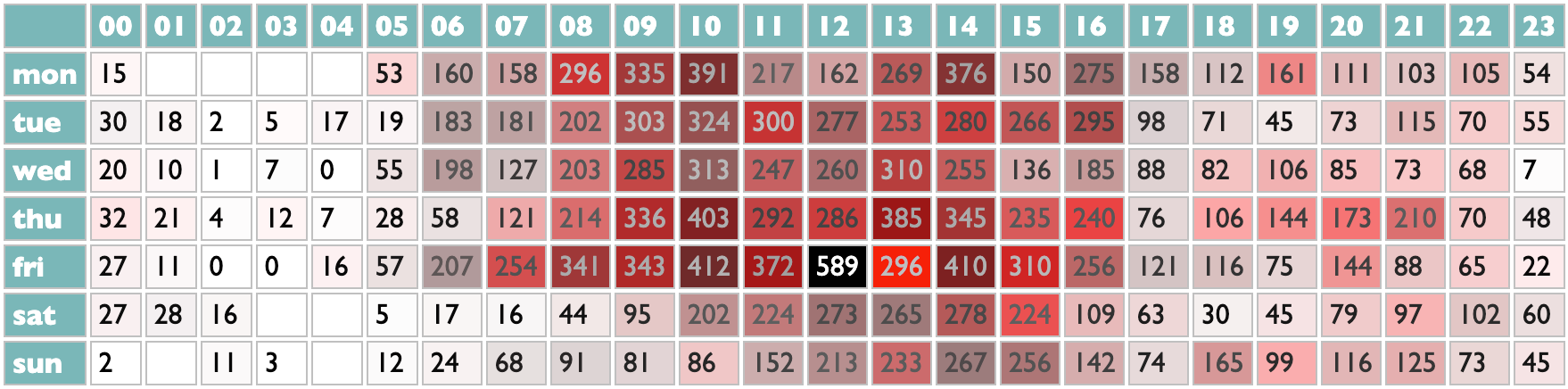
Musically speaking, though, the view by hour and weekday is more relevant. Spotify spent a lot of energy, over the years, trying to believe that most people's listening is heavily determined by day and time, and if yours is, now you have a playlist feature for that in Spotify. Mine isn't. I make a playlist of new songs I want to hear every Friday, and then I cycle through it, gradually deleting songs I don't end up enjoying, for the rest of the week. Thus there is absolutely no sense in which a have different music I prefer on Tuesday afternoon vs Thursday morning. What I do have, as you see here, is a very distinct pattern of doing a lot of rapid new-song scanning while eating my lunch on Fridays. Also, hopefully I do not have any important online accounts for which the security question is "At what hour are you most likely to be asleep?"
release years
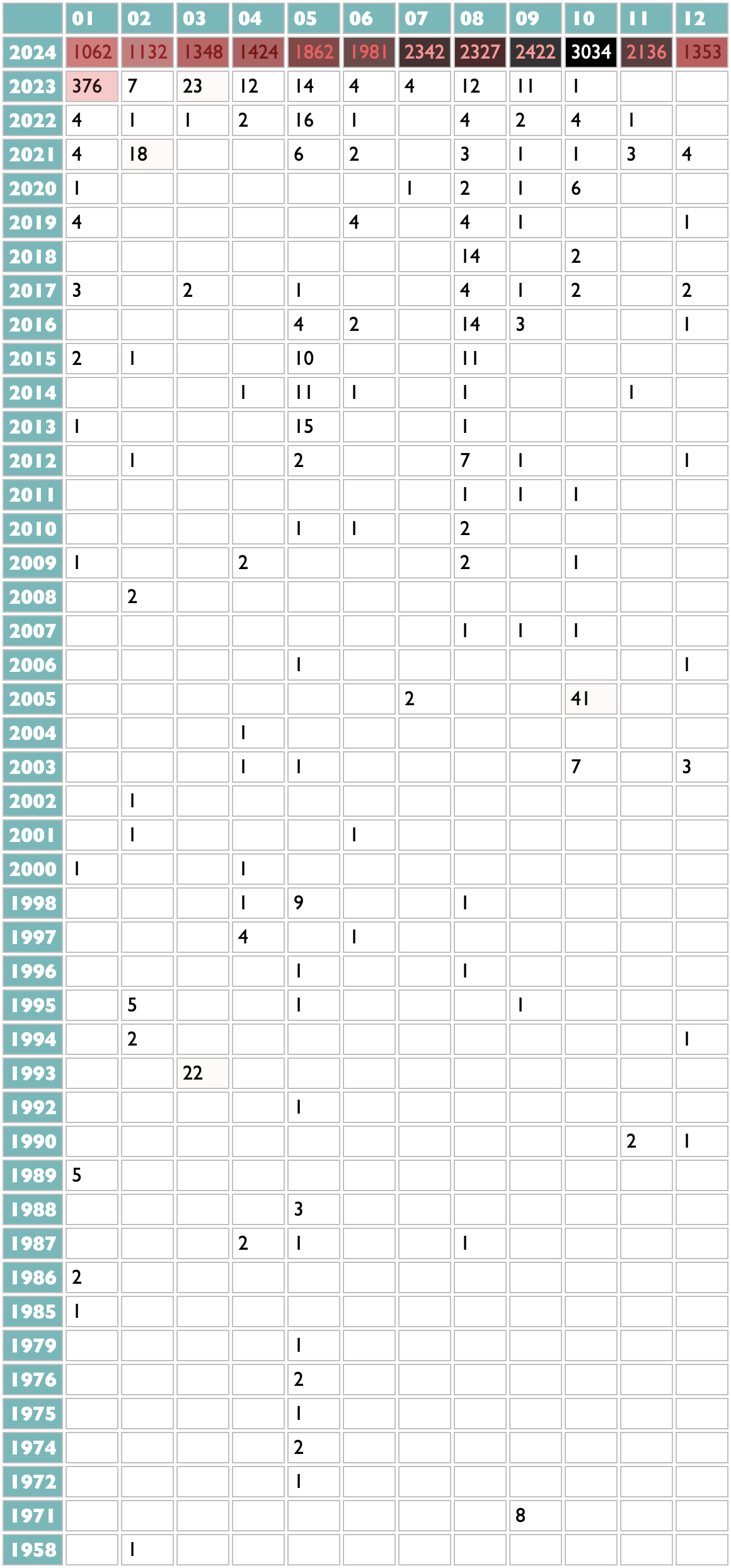
The fourth dimension in the Listening Personality was newness. I know, from data, that I am, or at least for a few years provably was, one of the handful of people in the world whose listening is most intently focused on new music. Curio thus has two different ways of looking at this particular quirk. This first one shows release years by listening months, so you can see how I listen to songs from the previous year for at least the first week of January, and how in May last year I tried and ultimately failed to find or remember one particular Ray Charles song.
song ages
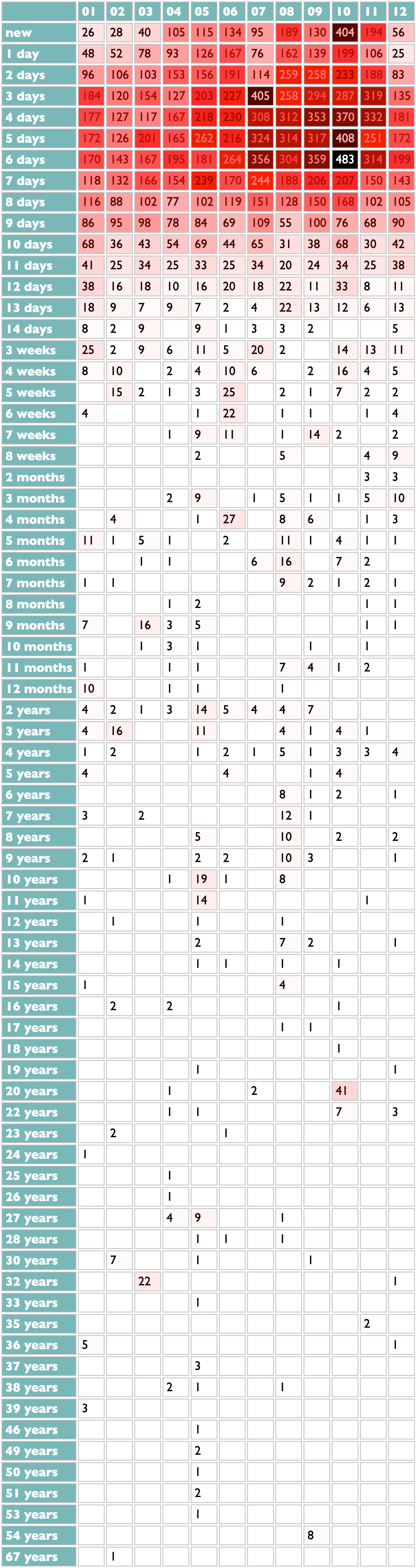
My listening pattern is even clearer in this view by song age, with ascending resolution. Friday is new-release day, but I am often distracted from listening over the weekends, so Monday through Thursday I am most heavily playing the songs from the previous Friday.
poster
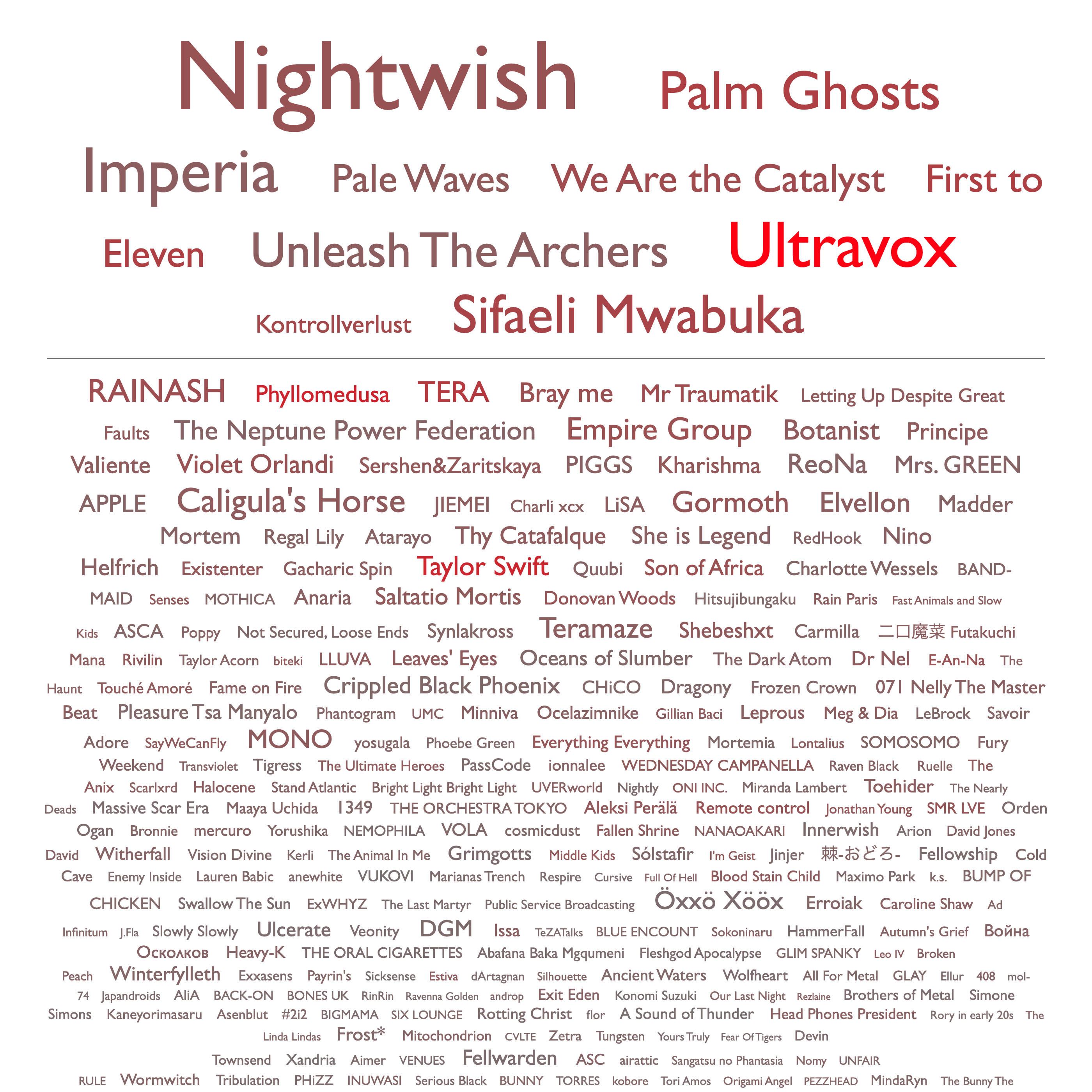
As you may already realize, my taste in visual design runs to the Tuftean. Don't make me justify the horizonal rule in this poster view to ET, but the order and sizes and colors here are all data-driven, and yet it's still faintly reminiscent of a concert-poster design.
10x10 grid
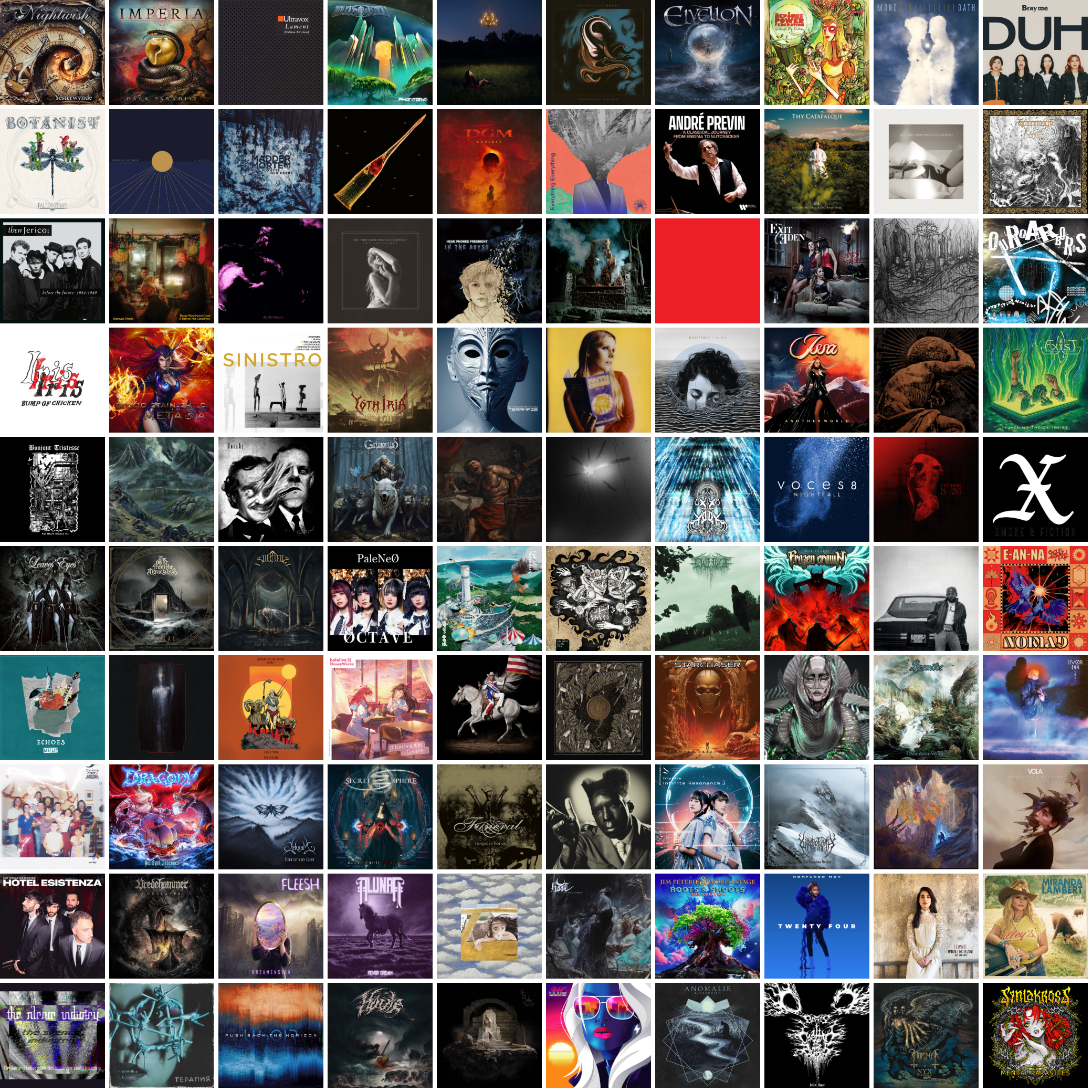
5x5 grid
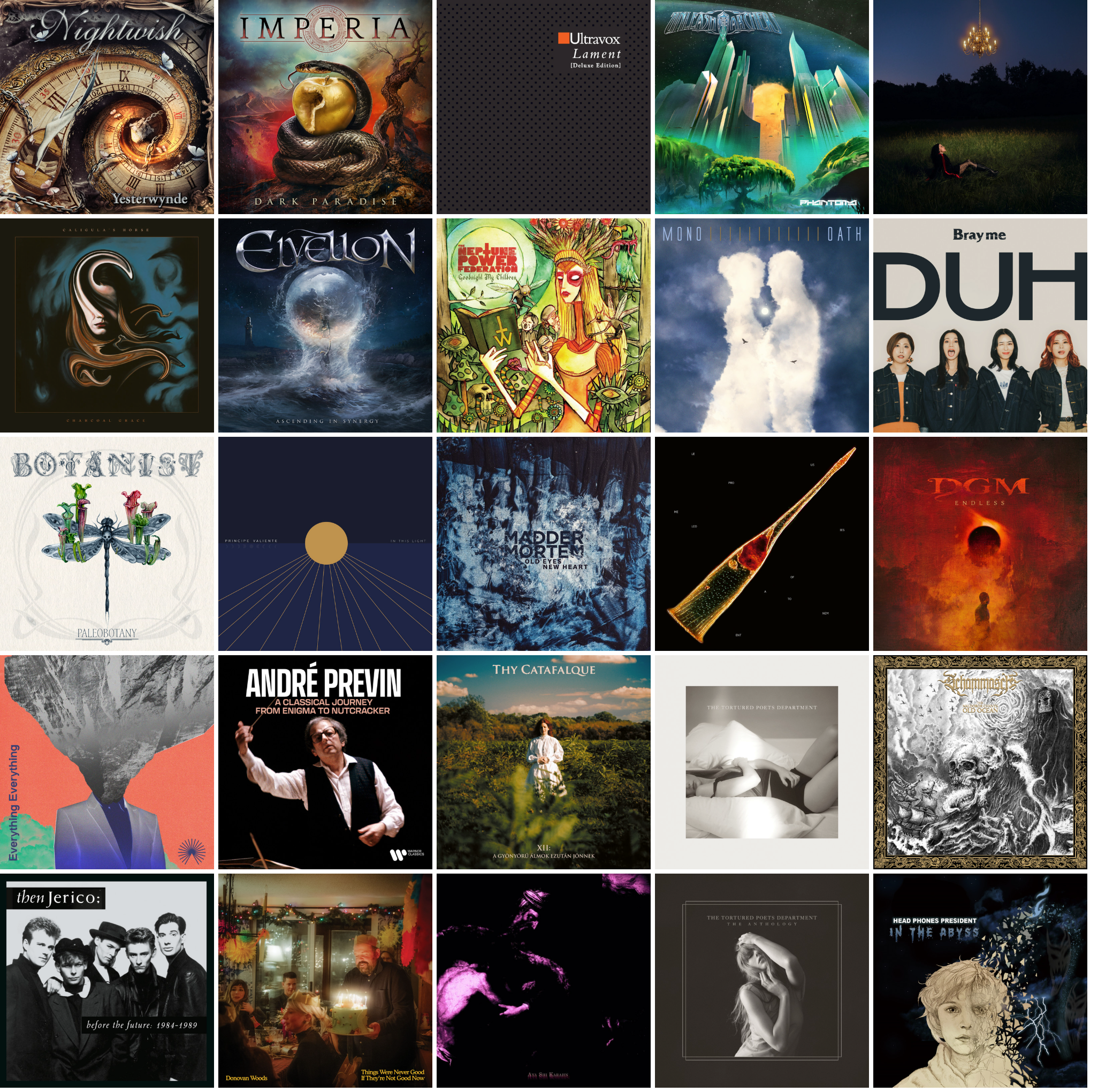
banner
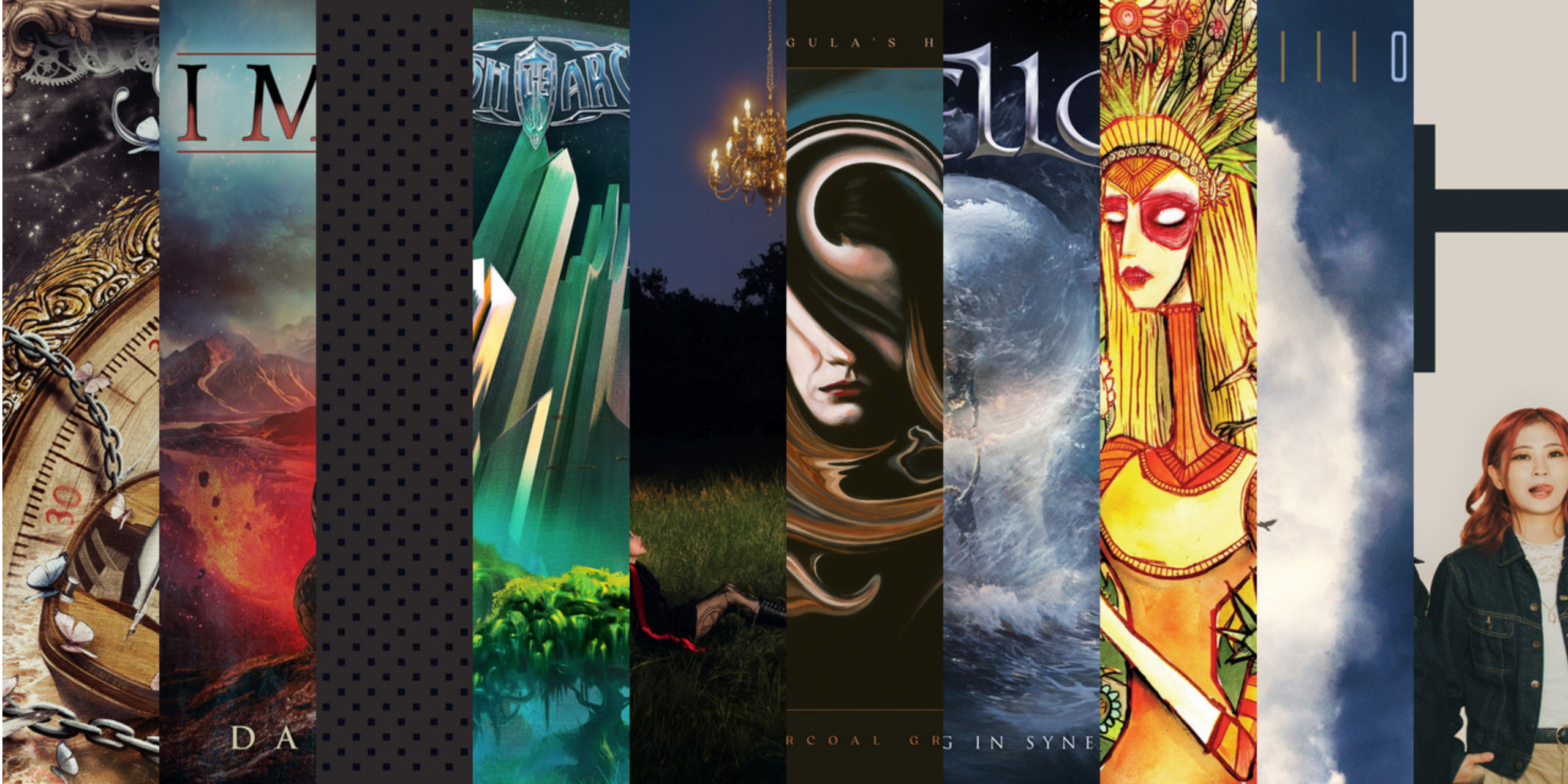
If I want a visual summary of my year in music, it should definitely be made out of the visual art that is already attached to the music, not something else.
playlist
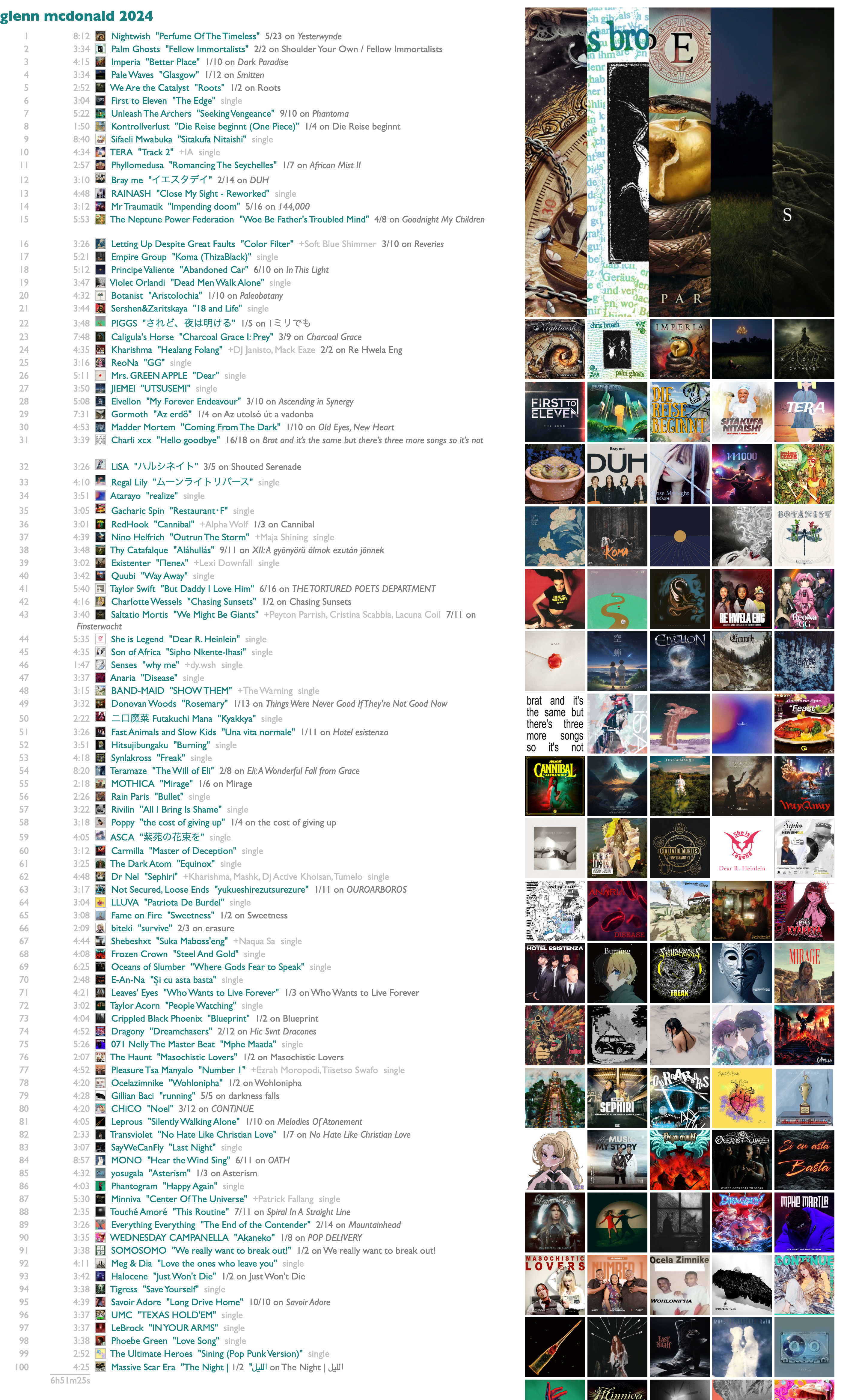
open this playlist in Spotify
The playlist, at least for me, is the authoritative final summary. It's my year in music, so it better be made of music, and it better make sense to me when I play it.
Spotify makes you a playlist, too, called Your Top Songs. It's fine, in that so far they haven't decided to screw it up with any kind of machine learning. It's never what I want, personally, because it ignores release dates and it has mutiple songs per artist and it doesn't sort the way I want.
What I want, and thus built, is not a Top Songs playlist, but an attempt at a playlist that represents a listening year. To do this it starts from the artist list, not song list, and then tries to pick your most-played new song from each of those artists.
Here's what that looks like in Dactal query syntax, as you can see if you click the "see the query for this" link at the bottom of the playlist table, except I've added a little color-coding:
2024 tracks full
/artist=(.track info.artists:@1),song=(.track info.name),date
|songdatepoints=(....count,sqrt)
/artist,song
||songpoints=(..of...songdatepoints,total),
songtime=(..of..of....ms_played,total)
#rank=songpoints,songtime
/artist
|artistpoints=(.of..songpoints..(...._,(0.5),difference)....total),
ms_played=(..of..songtime,total),
topsong=(.of:@1.of#(.of.track info.album:album_type=album),(.of.ts):@1.of.track info)
#artistpoints,ms_played:@<=100
.(...artistpoints=artistpoints,
duration=(.topsong....duration_ms,mmss),
artist=(.topsong.artists:@1.name),
track=(.topsong.name),
uri=(.topsong.uri))
/artist=(.track info.artists:@1),song=(.track info.name),date
|songdatepoints=(....count,sqrt)
/artist,song
||songpoints=(..of...songdatepoints,total),
songtime=(..of..of....ms_played,total)
#rank=songpoints,songtime
/artist
|artistpoints=(.of..songpoints..(...._,(0.5),difference)....total),
ms_played=(..of..songtime,total),
topsong=(.of:@1.of#(.of.track info.album:album_type=album),(.of.ts):@1.of.track info)
#artistpoints,ms_played:@<=100
.(...artistpoints=artistpoints,
duration=(.topsong....duration_ms,mmss),
artist=(.topsong.artists:@1.name),
track=(.topsong.name),
uri=(.topsong.uri))
The red parts are the artist query, which goes like this:
- get all your 2024 tracks (from a previous query)
- group these by each track's first artist, song name and date
- assign the square root of the number of times you played that artist/song that day as songdatepoints
- group those artist/song/date groups by artist and song
- total the songdatepoints from all dates per artist/song
- also total the amount of time you played this artist/song
- sort and rank the artist/song groups by songpoints and then songtime (in both cases from larger to smaller)
- group those artist/song groups by artist
- assign each artist a total artistpoints value that is the sum of songpoints-.5 (to reduce the weight of lots of songs you only played once)
- total up the amount of time you listened to that artist
The playlist query inserts one more line (an annotation suboperation) to calculate each artist's topsong for the playlist by taking the artist's highest-ranking artist/song group and getting an album track if one is available from the potentially multiple tracks representing that song, and the one you streamed first otherwise.
The artist query already sorts the artists by artistpoints and then listening time, which is the same order I wanted for the playlist. The :@<=100 picks the first 100, and the final block in parentheses just constructs some nicer columns for the final view. Curio watches for query results with uri columns, and offers to make them into a playlist for you, as you'll see.
The other thing I always wanted in Wrapped and never got, however, is the ability to remove things. Sometimes it's technically true that you played a song a lot, but not emotionally true that you liked it. Or, in my case, it's technically true that the reissue of Ultravox's Lament is a 2024 release, but I don't consider new repackagings of old recordings of "Dancing With Tears in My Eyes" to be new songs, so I prefer to remove that one. The chaining nature of Dactal makes this easy. That final sorting/filtering line just becomes
#artistpoints,ms_played:uri!=[spotify:track:3sJYcbQ3CQCpCajduB9UK2]:@<=100
with the limit filter still at the end so we get 100 tracks instead of 99 despite dropping one. It would also be possible to filter Ultravox out at the artist level, or Lament out at the album level, but it doesn't make any difference to the results.
That's all what Curio gives you, including deleting tracks:

But this still isn't quite what I want, and I am stubborn. I make playlists every week, during the year, and these sometimes represent my decisions, after a week of listening to more than one track from an album, which one to keep. And it's not always the one I played the most times. Curio already has this playlist data, or can have it, if you provide an indexing pattern at the bottom of the Playlists page. This can be as simple as * to index everything, but I have lots of playlists that I made for reasons other than my taste, so I only index these two particular sets:

(End partial matches with asterisks, and separate multiple patterns with a space, so the commas here are part of the matching patterns.)
So my own personal variation adds one more wrinkle to the artist/song-group sorting:
/artist,song<
||songpoints=(..of...songdatepoints,total),
songtime=(..of..of....ms_played,total),
songkey=(....(.artist.id),song,concatenate),
s2pa=(.songkey.songkey to playlist appearances.(1))
#rank=s2pa,songpoints,songtime
||songpoints=(..of...songdatepoints,total),
songtime=(..of..of....ms_played,total),
songkey=(....(.artist.id),song,concatenate),
s2pa=(.songkey.songkey to playlist appearances.(1))
#rank=s2pa,songpoints,songtime
The songkey line computes an artist/song key-string, which the s2pa line then uses to navigate into another dataset, itself produced by a query, that indexes playlist appearances by those same artist/song keys. I could use this dataset join to sort tracks by playlist counts or dates or anything, but all I really want to do is prefer tracks that I put on a playlist to ones I didn't, if there's a choice. So the .(1) part results in a 1 for songkeys that are in the songkey to playlist apperances dataset, and nothing for songkeys that aren't, and Dactal always sorts something before nothing.
There is absolutely no way that you could possibly tell the difference between me doing this and not, nor any reason you should care, but I care. What you choose to care about, in your own data and life, is your business, but the moral function of software is to let us express our care, and thus to encourage us to realize we have it. Your data is yours. The stories it tells are your stories. You should want them to be right, and nobody but you should get to tell you what that right is.
You don't have to care, but I want you to. The more we all care about everything, the less we will tolerate it any of it being bad.
¶ Data Rights · 22 December 2024 essay/tech
Your data is yours. Data derived from your actions, your tastes, your active and passive online presences, is all your data. Your public life generates public data, which contributes to collective knowledge, but in addition to personal knowledge, not in place of it.
You are entitled to both your public and private data. Your public data can be used by the public without your consent, but not without your awareness and their accountability. You are entitled to an intelligible and verifiable explanation of how it has been used. You are entitled to be able to double-check the sorting of your Spotify Wrapped just as you can double-check the math for the interest payments from your savings account.
You may choose to share your private data with other people, or applications, or corporations, in order to let them do something for you, or to help you do something for other people. For this your informed consent is necessary, and thus you are entitled to an intelligible and verifiable explanation of how your data would be used if you permit. You are entitled to know what Spotify would do with your Wrapped before you decide whether to join.
This is the world we have now:
you < corporations > software > your data
This is the world we want:
you > your data > software > corporations
The actors are the same, but the roles and the power are not. Today most computational power is structurally centralized and hoarded, and thus its potential for conversion into human energy is constrained and reduced. Most software is made by corporations, formulated for their corporate goals, and sealed against any other access or experimentation. Recent developments like LLM AIs seem inertially on a path towards even more centralized power-control and thus individual and social powerlessness.
We want a future, instead, in which creative power is widely distributed and human energy is bountifully amplified. We want software creation to be democratized so that our sources of imagination can be more broadly recruited. We want people and groups to have the power to pursue their own goals, not just for our own narrow sakes, but for our collective potential.
For this world to exist, we must figure out how, both logistically and politically, to move the data layer on which most meaningful software acts into the computational and conversational open. We need not just data portability -- the right to chose between evils -- but a shared language for talking about algorithms and data logic like we use math to discuss numbers. We need to be able to talk about what we want, and test what we might have and how.
This is how the AT Protocol, on which the social microblogging platform Bluesky runs, is designed. Its schemas are public, its public information is public. Bluesky, the application, makes use of this protocol and your data to construct a social experience for you and with you, producing feeds and following and public conversations and personal data ownership. The Bluesky software is open source, and most of the data relationships that constitute the social network are derivable from accessible data in tractable ways. But the Bluesky application still conceals the data layer more than it exposes it, so I made a ruthlessly basic Bluesky query interface called SkyQ to try to invert this. You can see the data directly, and wander through it both curiously and computationally. You can build data tools for yourself, or for everyone, that everyone can share.
Current music streaming services, like Spotify, are not built this way at all. Your Spotify listening data is yours, morally, but so inaccessible to you that Spotify can make a yearly spectacle out of briefly sharing the most superficial and unverifiable analyses of it with you. And the collective knowledge that we, 600 million of us, amass through our listening, is so inaccessible to us that Spotify can passively deprive us of its insights just by not caring.
Curio, thus, my web thing for collating music curiosity, is both an experiment in making a music interface that does music things the way I personally want them done, but also a meta-experiment in making a data experience that uses your data with respect for your data rights. Every Curio page has data link at the bottom. Every bit of data Curio stores is also visible directly, on a query page where you can explore it however you like. I made a bunch of Spotify-Wrapped-like tools with which you can analyze your listening, but they do so with queries you can see, check, change or build upon, so if your goals diverge from mine, you are free to pursue them. The more paths we can follow, the more we will learn about how to reach anywhere.
There is a lot more to the human future of Data Rights than just microblogging and listening-history heatmaps, obviously. We are not yet near it, and we probably won't reach it with just our web browsers and a query language and a manifesto. Maybe no tendrils of these specific current dreams of mine will end up swirling in whatever collective dreams we eventually create by agreeing to share. I claim no certainty about the details. Certainty is not my goal. Possibility? Less resignation, more hope. I'm totally sure of almost nothing.
But I'm pretty sure we only get dreamier futures by dreaming.
You are entitled to both your public and private data. Your public data can be used by the public without your consent, but not without your awareness and their accountability. You are entitled to an intelligible and verifiable explanation of how it has been used. You are entitled to be able to double-check the sorting of your Spotify Wrapped just as you can double-check the math for the interest payments from your savings account.
You may choose to share your private data with other people, or applications, or corporations, in order to let them do something for you, or to help you do something for other people. For this your informed consent is necessary, and thus you are entitled to an intelligible and verifiable explanation of how your data would be used if you permit. You are entitled to know what Spotify would do with your Wrapped before you decide whether to join.
This is the world we have now:
you < corporations > software > your data
This is the world we want:
you > your data > software > corporations
The actors are the same, but the roles and the power are not. Today most computational power is structurally centralized and hoarded, and thus its potential for conversion into human energy is constrained and reduced. Most software is made by corporations, formulated for their corporate goals, and sealed against any other access or experimentation. Recent developments like LLM AIs seem inertially on a path towards even more centralized power-control and thus individual and social powerlessness.
We want a future, instead, in which creative power is widely distributed and human energy is bountifully amplified. We want software creation to be democratized so that our sources of imagination can be more broadly recruited. We want people and groups to have the power to pursue their own goals, not just for our own narrow sakes, but for our collective potential.
For this world to exist, we must figure out how, both logistically and politically, to move the data layer on which most meaningful software acts into the computational and conversational open. We need not just data portability -- the right to chose between evils -- but a shared language for talking about algorithms and data logic like we use math to discuss numbers. We need to be able to talk about what we want, and test what we might have and how.
This is how the AT Protocol, on which the social microblogging platform Bluesky runs, is designed. Its schemas are public, its public information is public. Bluesky, the application, makes use of this protocol and your data to construct a social experience for you and with you, producing feeds and following and public conversations and personal data ownership. The Bluesky software is open source, and most of the data relationships that constitute the social network are derivable from accessible data in tractable ways. But the Bluesky application still conceals the data layer more than it exposes it, so I made a ruthlessly basic Bluesky query interface called SkyQ to try to invert this. You can see the data directly, and wander through it both curiously and computationally. You can build data tools for yourself, or for everyone, that everyone can share.
Current music streaming services, like Spotify, are not built this way at all. Your Spotify listening data is yours, morally, but so inaccessible to you that Spotify can make a yearly spectacle out of briefly sharing the most superficial and unverifiable analyses of it with you. And the collective knowledge that we, 600 million of us, amass through our listening, is so inaccessible to us that Spotify can passively deprive us of its insights just by not caring.
Curio, thus, my web thing for collating music curiosity, is both an experiment in making a music interface that does music things the way I personally want them done, but also a meta-experiment in making a data experience that uses your data with respect for your data rights. Every Curio page has data link at the bottom. Every bit of data Curio stores is also visible directly, on a query page where you can explore it however you like. I made a bunch of Spotify-Wrapped-like tools with which you can analyze your listening, but they do so with queries you can see, check, change or build upon, so if your goals diverge from mine, you are free to pursue them. The more paths we can follow, the more we will learn about how to reach anywhere.
There is a lot more to the human future of Data Rights than just microblogging and listening-history heatmaps, obviously. We are not yet near it, and we probably won't reach it with just our web browsers and a query language and a manifesto. Maybe no tendrils of these specific current dreams of mine will end up swirling in whatever collective dreams we eventually create by agreeing to share. I claim no certainty about the details. Certainty is not my goal. Possibility? Less resignation, more hope. I'm totally sure of almost nothing.
But I'm pretty sure we only get dreamier futures by dreaming.
¶ Subgenres, subcontinents · 9 December 2024 essay/listen/tech
¶ Know Yourself (For Free) · 30 November 2024 listen/tech
It's that time of year when companies begin pretending that a) the year is already over, and b) you should be grateful to them for giving you a tiny yearly glimpse of your own data.
But your data is yours. You shouldn't have to elaborately ask for it, but that tends to be the state at the moment. You can get your streaming history from Spotify by going to Account > Security and privacy > Account privacy (of course). They're hoping you don't, because they really want you to eagerly wait for them to tell you what you listened to this year (not counting the last few weeks of it) in parsimoniously abbreviated detail and laboriously garish graphic design.
But if you do, because you can, and you get a Spotify API key, which you can, then you can play with Curio, my experimental app for organizing music curiosity. Curio does a potentially puzzling assortment of things that I like to do, but it also has a query language, so that neither of us is limited by what I already think I want.
And so, among other things, you can make your own Wrapped.
Request your data. When you get it, it'll be a zip file. Unzip it and you'll get a folder full of files. Go to the Curio query page. Hit the "Load more data" button and select all the downloaded files in that folder whose names start with "Streaming_History_Audio_". Curio will stitch them all back together for you. Now you have your listening history.
Of course, to make sense of your listening history, you would need a bit more data. But you could run this query:
listening history
:ts>=2024
:ms_played>=30000
|id=(...spotify_track_uri,([:]),split.split:@3)
|track info=(.id.other tracks)
:(.track info.album.release_date:>2024)
|date=(...ts,(T),split.split:@1)
Translated, that says:
- start with your whole listening history
- filter that to just the plays from 2024
- filter that to just the songs you played at least 30 seconds of
- perform some arcane shenanigans to extract the ID from the Spotify track URI
- use that ID to get the full track info from the Spotify API
- filter the list to just the tracks that were released in 2024, because to a music person that's what "year in music" means
- extract the date from the Spotify timestamp
Hit Enter to run that, and then type "2024 tracks full" in the query-name box to the right, and hit Enter there too.
Now you have the right data to start doing some analysis.
You could now, for example, run this query:
2024 tracks full
/artist=(.track info.artist:@1),song=(.track info.name),date
|points=(....count,sqrt....sqrt)
/artist,song
|points=(.of....points,total)
#rank=points
- take the 2024 tracks from the first query
- group them by artist, song name and date
- give each artist-song-date triplet the quad root of the number of times you played it that day (because I don't trust looping very much)
- group the artist-song-date triplets into artist-song pairs
- score the pairs by totaling their triplets
- sort and rank the pairs by points
You could save that one as "2024 songs scored". Notice that it doesn't end after 5, or even after 100.
You could also do a very similar query for artists, just skipping the song part:
2024 tracks full
/artist=(.track info.artist),date
|points=(....count,sqrt)
/artist
|points=(.of....points,total)
#rank=points
And if you save that one as "2024 artists scored", then you could also use it for:
2024 artists scored
/genre=(.artist.artist genres.genres)
|points=(....count,sqrt....total),top artist=(.of:@1.name)#rank=points
and now you have a top genre list that isn't limited to 5, shows you artist counts and your top artist for each, and because this is all data, even lets you see which artists belong to every genre (click the "of[...]" link).
But those are just questions I asked. You can ask your own.
You should always be able to ask your own questions about yourself. You should demand to be.
But your data is yours. You shouldn't have to elaborately ask for it, but that tends to be the state at the moment. You can get your streaming history from Spotify by going to Account > Security and privacy > Account privacy (of course). They're hoping you don't, because they really want you to eagerly wait for them to tell you what you listened to this year (not counting the last few weeks of it) in parsimoniously abbreviated detail and laboriously garish graphic design.
But if you do, because you can, and you get a Spotify API key, which you can, then you can play with Curio, my experimental app for organizing music curiosity. Curio does a potentially puzzling assortment of things that I like to do, but it also has a query language, so that neither of us is limited by what I already think I want.
And so, among other things, you can make your own Wrapped.
Request your data. When you get it, it'll be a zip file. Unzip it and you'll get a folder full of files. Go to the Curio query page. Hit the "Load more data" button and select all the downloaded files in that folder whose names start with "Streaming_History_Audio_". Curio will stitch them all back together for you. Now you have your listening history.
Of course, to make sense of your listening history, you would need a bit more data. But you could run this query:
listening history
:ts>=2024
:ms_played>=30000
|id=(...spotify_track_uri,([:]),split.split:@3)
|track info=(.id.other tracks)
:(.track info.album.release_date:>2024)
|date=(...ts,(T),split.split:@1)
Translated, that says:
- start with your whole listening history
- filter that to just the plays from 2024
- filter that to just the songs you played at least 30 seconds of
- perform some arcane shenanigans to extract the ID from the Spotify track URI
- use that ID to get the full track info from the Spotify API
- filter the list to just the tracks that were released in 2024, because to a music person that's what "year in music" means
- extract the date from the Spotify timestamp
Hit Enter to run that, and then type "2024 tracks full" in the query-name box to the right, and hit Enter there too.

Now you have the right data to start doing some analysis.
You could now, for example, run this query:
2024 tracks full
/artist=(.track info.artist:@1),song=(.track info.name),date
|points=(....count,sqrt....sqrt)
/artist,song
|points=(.of....points,total)
#rank=points
- take the 2024 tracks from the first query
- group them by artist, song name and date
- give each artist-song-date triplet the quad root of the number of times you played it that day (because I don't trust looping very much)
- group the artist-song-date triplets into artist-song pairs
- score the pairs by totaling their triplets
- sort and rank the pairs by points
You could save that one as "2024 songs scored". Notice that it doesn't end after 5, or even after 100.
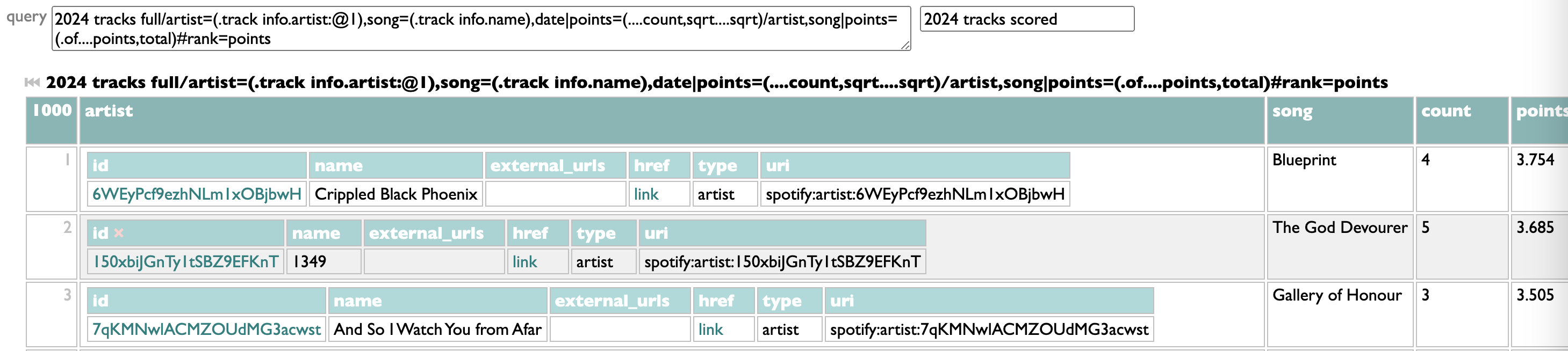
You could also do a very similar query for artists, just skipping the song part:
2024 tracks full
/artist=(.track info.artist),date
|points=(....count,sqrt)
/artist
|points=(.of....points,total)
#rank=points
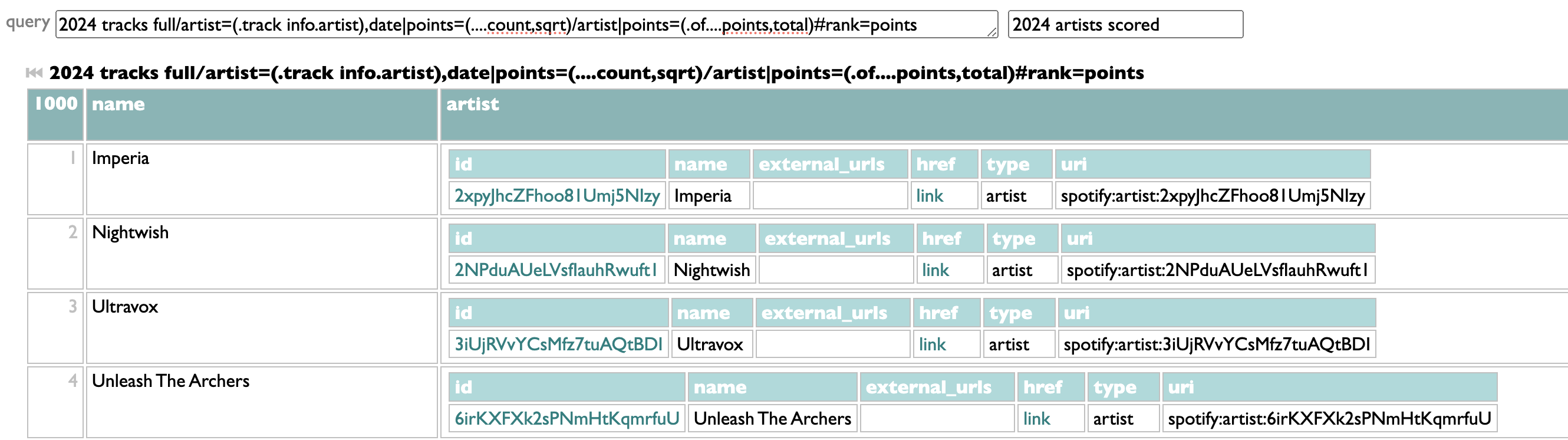
And if you save that one as "2024 artists scored", then you could also use it for:
2024 artists scored
/genre=(.artist.artist genres.genres)
|points=(....count,sqrt....total),top artist=(.of:@1.name)#rank=points
and now you have a top genre list that isn't limited to 5, shows you artist counts and your top artist for each, and because this is all data, even lets you see which artists belong to every genre (click the "of[...]" link).
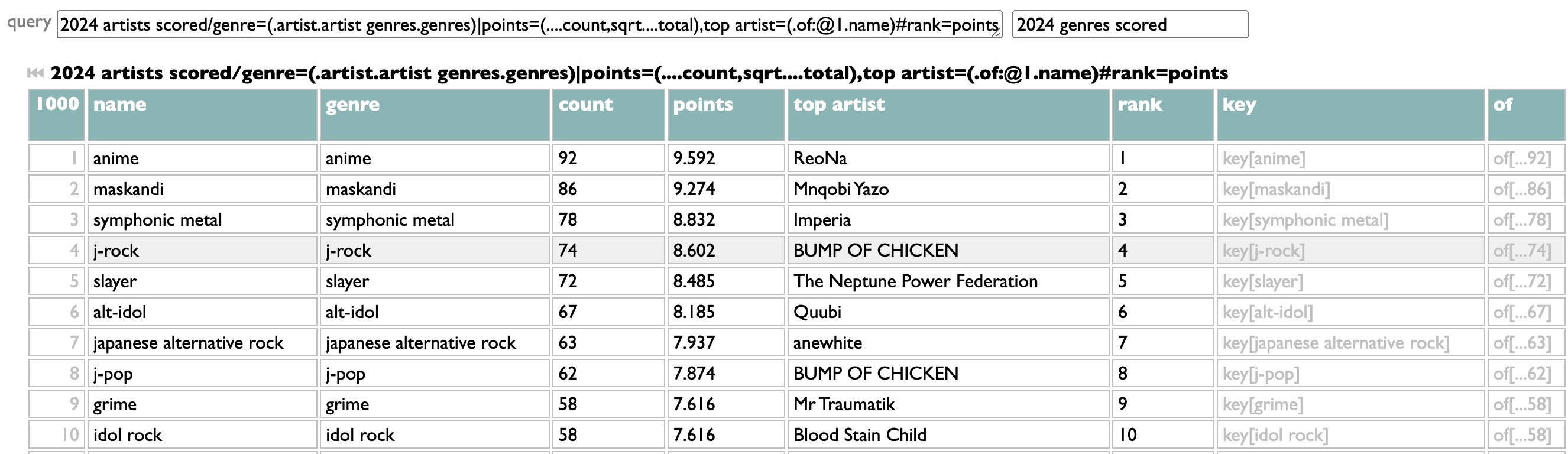
But those are just questions I asked. You can ask your own.
You should always be able to ask your own questions about yourself. You should demand to be.
¶ 6 November 2024
The next democracy we build will have to be better than this one.
¶ You've had 200 years to figure out how to search for this. · 30 September 2024 listen/tech
Streaming is a great way to listen to classical music. You have to ignore essentially all algorithmic recommendation features, which are generally oriented around tracks and playlists, but this isn't a terrible general policy anyway. Find the music you want to hear, and listen to it. If there are tools that help you find stuff, that's great.
If there are tools that are supposed to help you find stuff, but don't, that's less great. I started thinking about this again after seeing a plaintive Reddit post from somebody trying, unsuccessfully, to find a classical recording composed by (Joseph) Haydn, conducted by (Eugen) Jochum and performed by (Staatskapelle) Dresden. They made it worse for themselves by trying to do it in the Spotify mobile app, but it's not a lot better in the desktop version:
The "Top result" is Haydn and Jochum, but not Dresden. The "Songs" are Haydn without Jochum or Dresden, and the Artists start with, obtusely, Jospeh Haydn's less-famous younger brother. And you see that 3 of 5 classical titles here get truncated before the most important part, despite word-wrap technology having existed for only slightly less of history than Haydn's symphonies. The page does scroll, but that's only helpful if you're looking for "Haydn Radio", which you shouldn't be, or a hot podcast about Haydn, which may or may not exist but I don't care which.
After trying a few other search variations, I was almost ready to conclude that the Haydn recordings in question were hard to find because they weren't actually on Spotify, but finally I looked them up elsewhere, and then I was able to find them on Spotify after all. Part of the problem is that album credits are usually less complete than track credits. This is an industry metadata issue, not a Spotify-specific problem, and there's no reason you should have to care about that difference any more than the Haydns do, but it means none of us are ever going to find the two albums of Haydn / Jochum / Dresden music on Spotify, because they are credited only to Haydn, whose artist page has 1465 releases.
Apple Music, of course, has a whole dedicated classical-music sub-service called Idagio, so we might expect the difference to be like night and day, and indeed it is in terms of color palettes:
Results-wise, however, a "Haydn Jochum Dresden" search here still finds only one of the four pieces it should, and a lot of irrelevance instead of the other three, and really we shouldn't need a whole bespoke streaming service just to upgrade our search success from 0/4 to 1/4.
We only need a little extra syntax to say what we want, and a tiny bit of extra post-processing to get it. Both of which I've now added to my existing research tools on everynoise.com.
The main addition is that you can append // to your track-name search (which here is blank because we're trying to find these without remembering what they're called) to filter by artist. Doing =Haydn looks for "Haydn" only in the name of the first artist, which in the case of classical releases is always the composer. +Dresden would specify matching "Dresden" in an artist name other than the first, but there's nobody named "Haydn Dresden" yet, so I didn't bother. Partial matches are fine in all cases, so we don't need to know whether Haydn is listed as Joseph or Franz Joseph, nor how to spell Eugen or Staatskapelle.
The extra bonus feature I added is the /// album bit, which says to find only tracks that appear on proper albums (as opposed to dodgy mood compilations), and then to group and sort the results by those albums. I also took the time to factor common prefixes out of the titles, which spares us only the repeated word "Symphony" in the case of the two omnibus Haydn packages above, but produces much more readable track lists in some more-common cases. Take, e.g., this:
Ah yes, Beethoven's immortal symphony "...", popularly known as "Ellipsica". All eight lines in the "Songs" section are truncated, and trust me that you don't need to know what the "artist" called "Beethoven Symphony No. 9" sounds like, unless you are currently less than six months old and suffering from colic. Why are there spaces before the commas in the "Top result" artist list, and why does every artist in the boldly labeled Artists section say "Artist" again under it?
With a little care, filtering and sorting, we can get this:
Real albums. Readable movements. No recommendations, no moods except for your own.
And none of this was even specific to classical music. You can use these same tools to find that Bowie/Jagger song about dancing:
To be fair, you could find that, plus some bonus Björk, with normal searching:
But my version has a wildcard filter so we can find all of Bowie's collaborations:
And even deduplicate them by song:
Or all the Ghetts tracks with no guests:
Or all the non-CHVRCHES songs featuring CHVRCHES:
It's not complicated. We don't need AI for this.
(Which is good, because the AI is, maybe, not quite ready...)
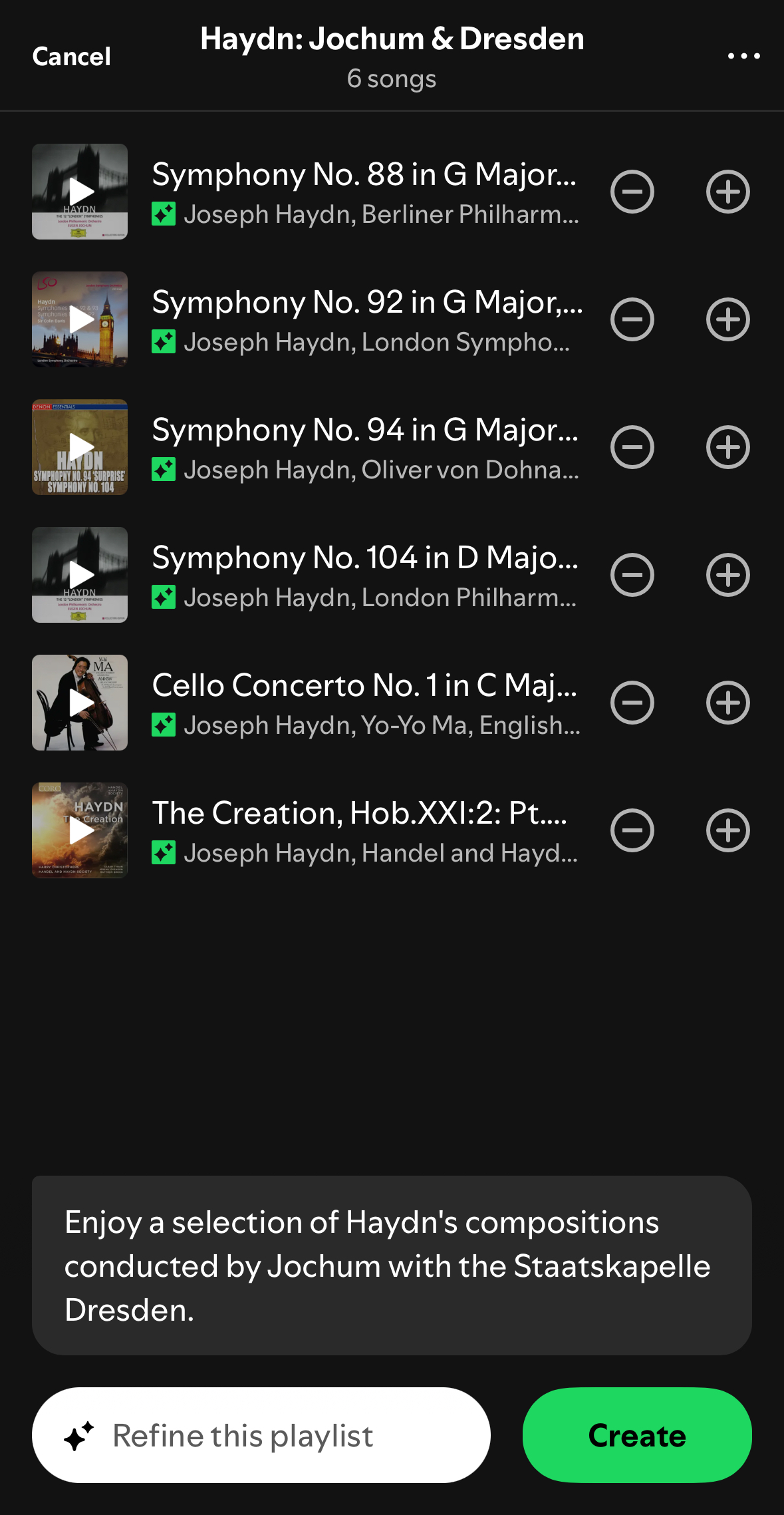
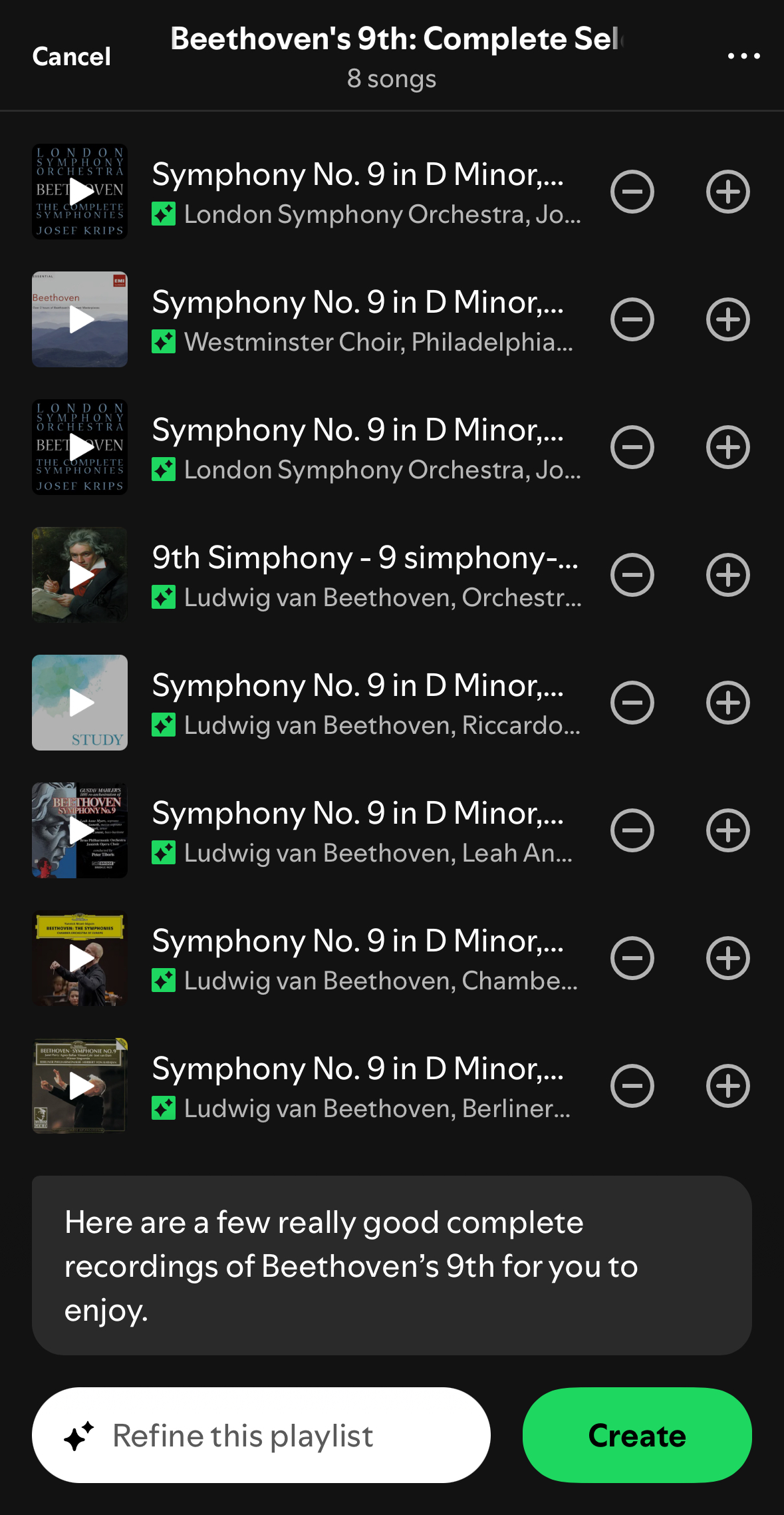
If there are tools that are supposed to help you find stuff, but don't, that's less great. I started thinking about this again after seeing a plaintive Reddit post from somebody trying, unsuccessfully, to find a classical recording composed by (Joseph) Haydn, conducted by (Eugen) Jochum and performed by (Staatskapelle) Dresden. They made it worse for themselves by trying to do it in the Spotify mobile app, but it's not a lot better in the desktop version:
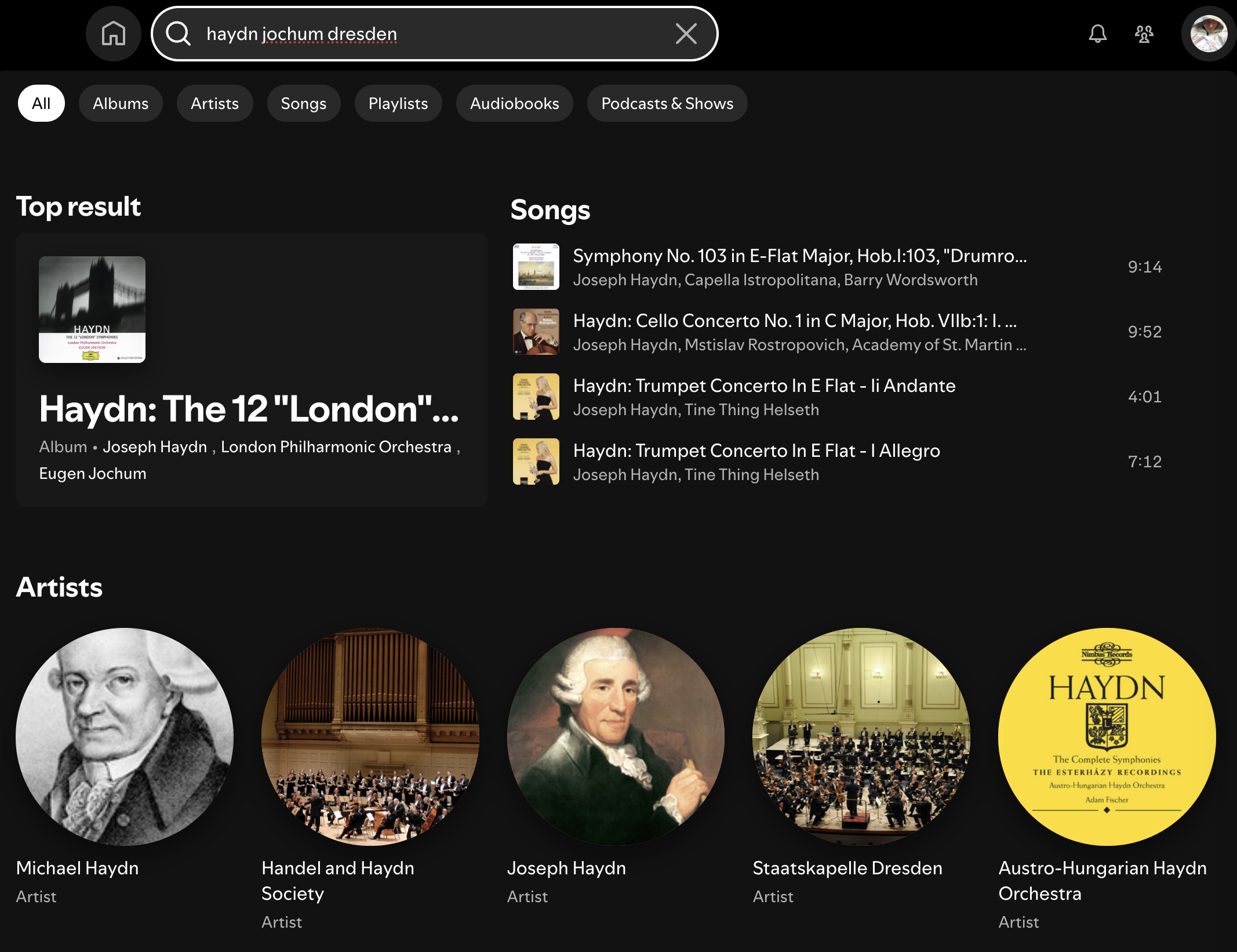
The "Top result" is Haydn and Jochum, but not Dresden. The "Songs" are Haydn without Jochum or Dresden, and the Artists start with, obtusely, Jospeh Haydn's less-famous younger brother. And you see that 3 of 5 classical titles here get truncated before the most important part, despite word-wrap technology having existed for only slightly less of history than Haydn's symphonies. The page does scroll, but that's only helpful if you're looking for "Haydn Radio", which you shouldn't be, or a hot podcast about Haydn, which may or may not exist but I don't care which.
After trying a few other search variations, I was almost ready to conclude that the Haydn recordings in question were hard to find because they weren't actually on Spotify, but finally I looked them up elsewhere, and then I was able to find them on Spotify after all. Part of the problem is that album credits are usually less complete than track credits. This is an industry metadata issue, not a Spotify-specific problem, and there's no reason you should have to care about that difference any more than the Haydns do, but it means none of us are ever going to find the two albums of Haydn / Jochum / Dresden music on Spotify, because they are credited only to Haydn, whose artist page has 1465 releases.
Apple Music, of course, has a whole dedicated classical-music sub-service called Idagio, so we might expect the difference to be like night and day, and indeed it is in terms of color palettes:
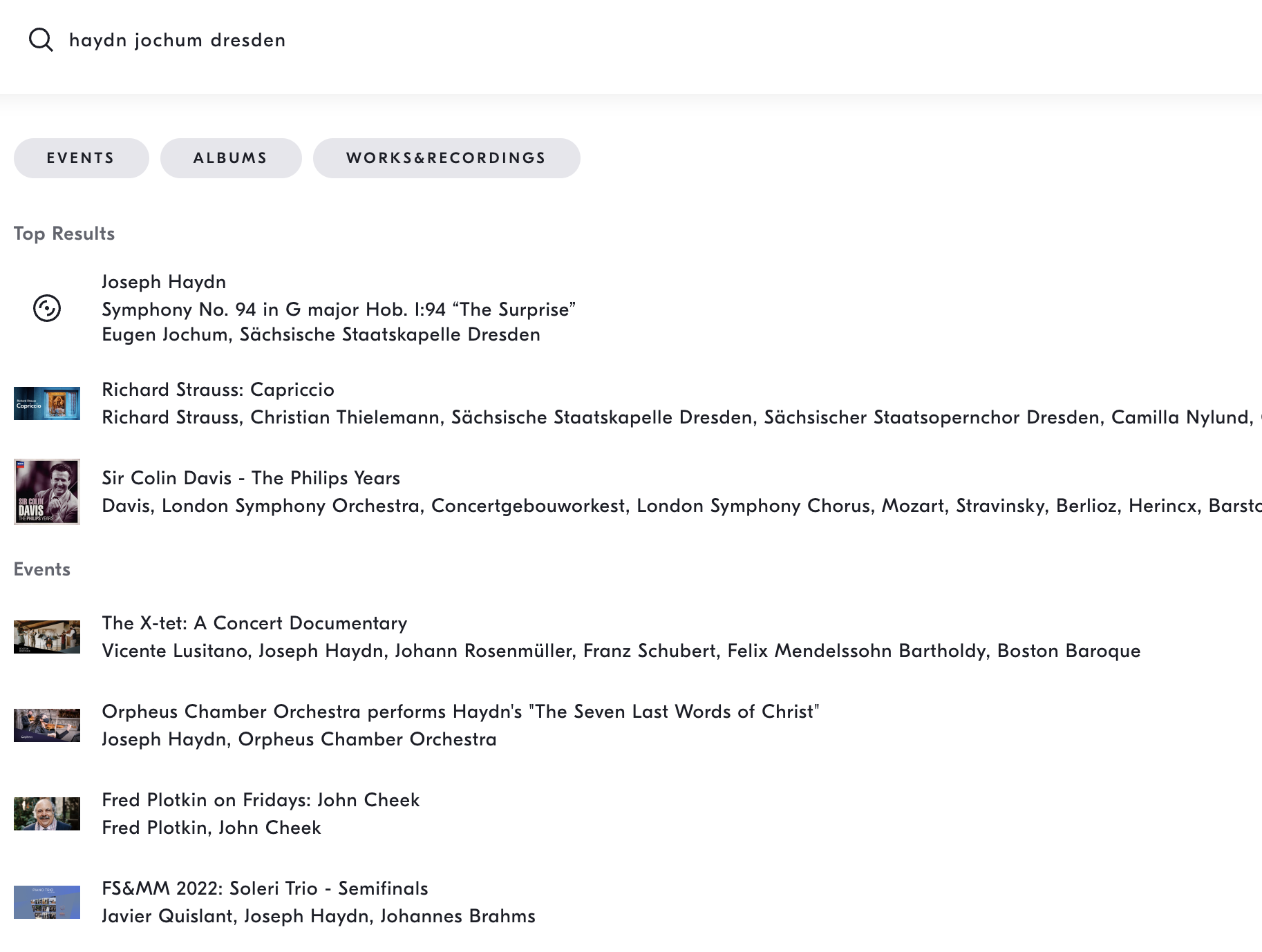
Results-wise, however, a "Haydn Jochum Dresden" search here still finds only one of the four pieces it should, and a lot of irrelevance instead of the other three, and really we shouldn't need a whole bespoke streaming service just to upgrade our search success from 0/4 to 1/4.
We only need a little extra syntax to say what we want, and a tiny bit of extra post-processing to get it. Both of which I've now added to my existing research tools on everynoise.com.
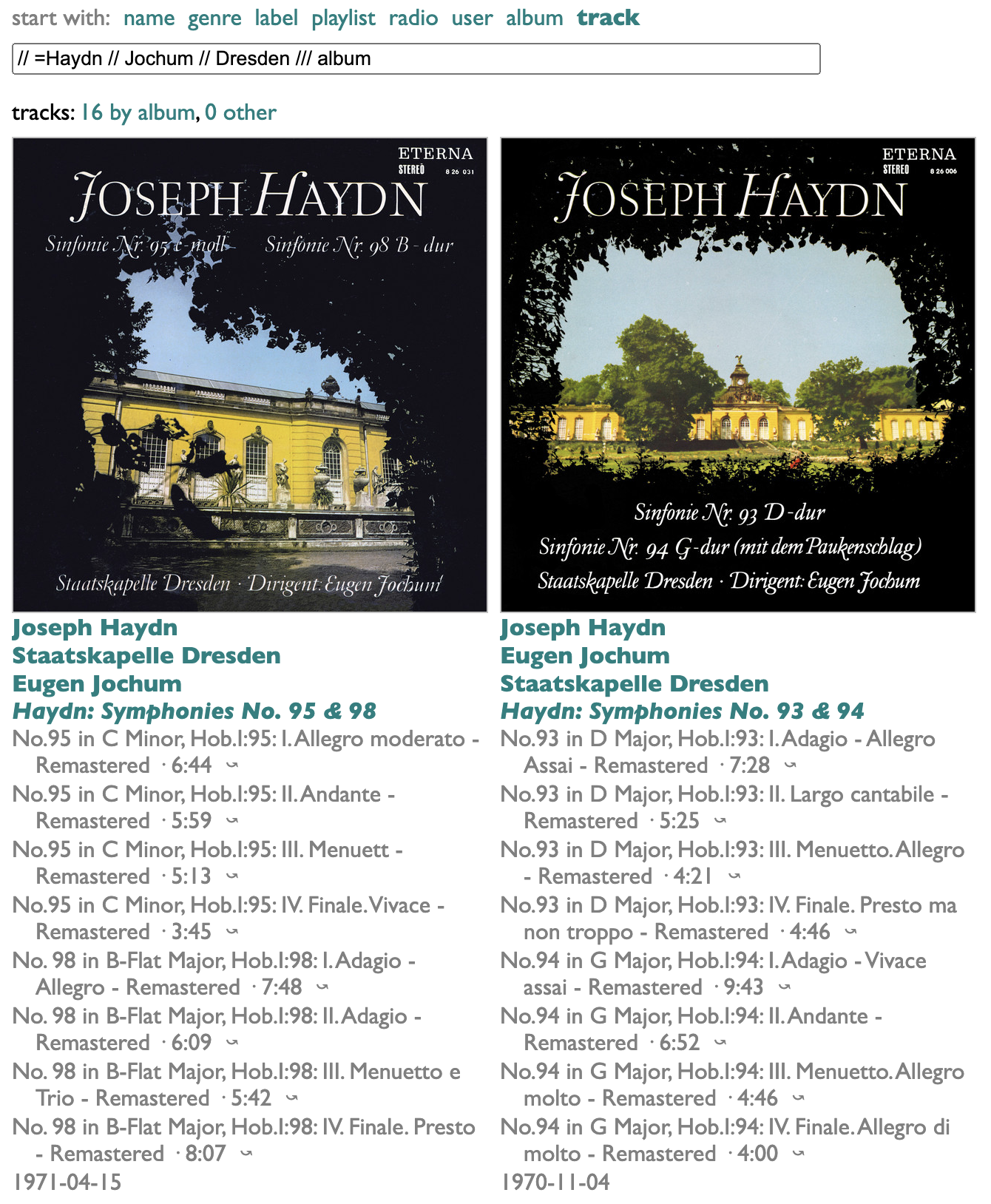
The main addition is that you can append // to your track-name search (which here is blank because we're trying to find these without remembering what they're called) to filter by artist. Doing =Haydn looks for "Haydn" only in the name of the first artist, which in the case of classical releases is always the composer. +Dresden would specify matching "Dresden" in an artist name other than the first, but there's nobody named "Haydn Dresden" yet, so I didn't bother. Partial matches are fine in all cases, so we don't need to know whether Haydn is listed as Joseph or Franz Joseph, nor how to spell Eugen or Staatskapelle.
The extra bonus feature I added is the /// album bit, which says to find only tracks that appear on proper albums (as opposed to dodgy mood compilations), and then to group and sort the results by those albums. I also took the time to factor common prefixes out of the titles, which spares us only the repeated word "Symphony" in the case of the two omnibus Haydn packages above, but produces much more readable track lists in some more-common cases. Take, e.g., this:
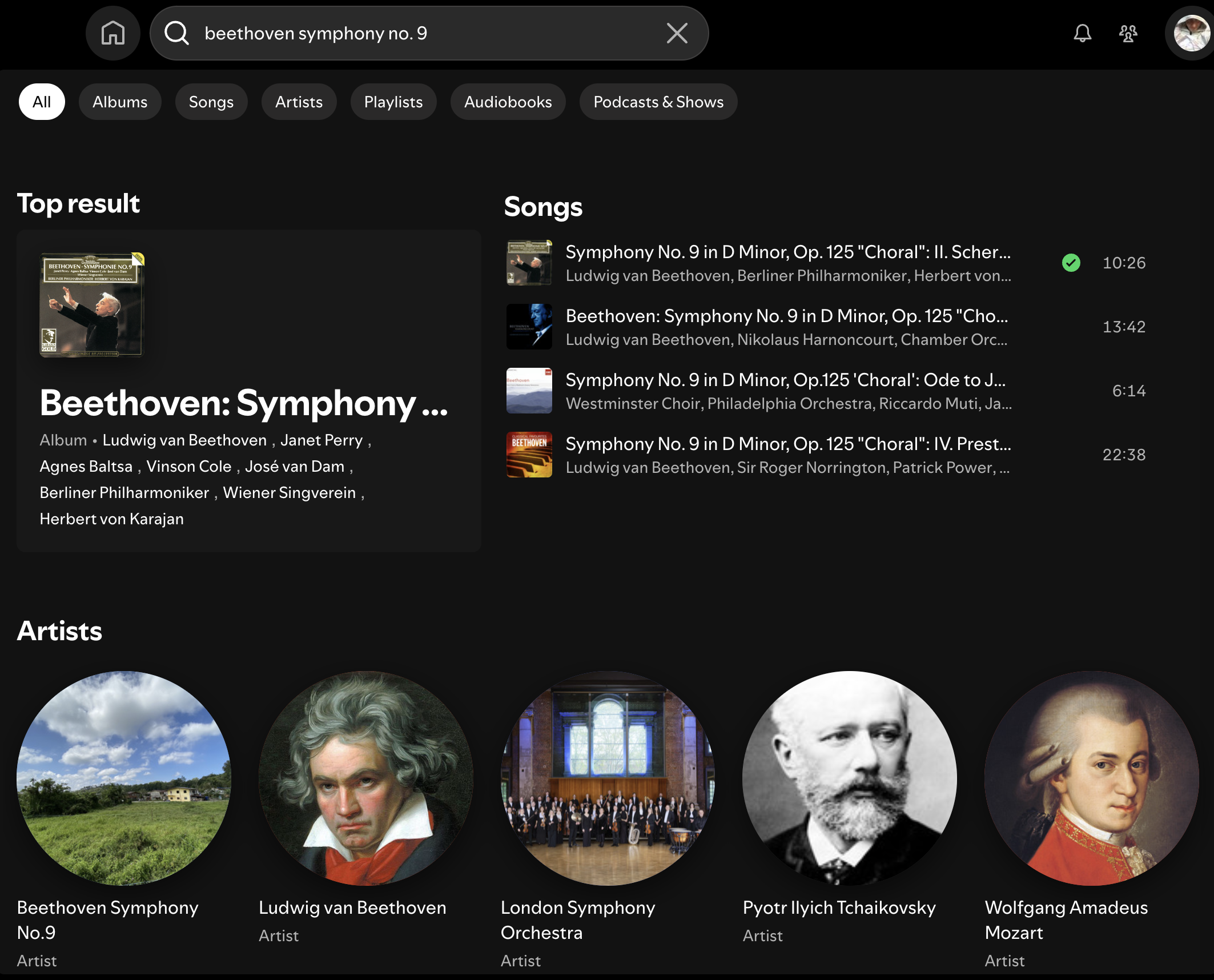
Ah yes, Beethoven's immortal symphony "...", popularly known as "Ellipsica". All eight lines in the "Songs" section are truncated, and trust me that you don't need to know what the "artist" called "Beethoven Symphony No. 9" sounds like, unless you are currently less than six months old and suffering from colic. Why are there spaces before the commas in the "Top result" artist list, and why does every artist in the boldly labeled Artists section say "Artist" again under it?
With a little care, filtering and sorting, we can get this:
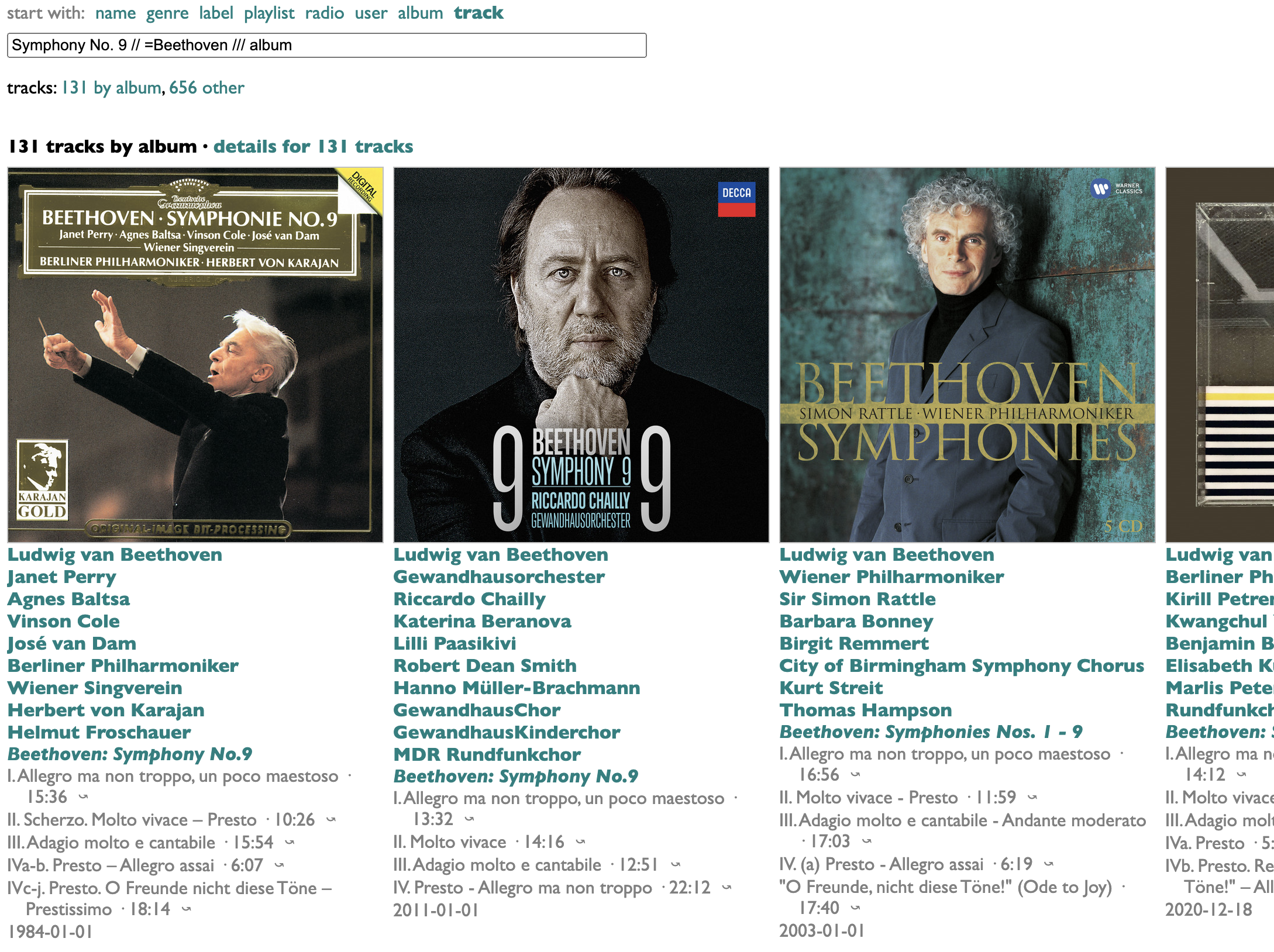
Real albums. Readable movements. No recommendations, no moods except for your own.
And none of this was even specific to classical music. You can use these same tools to find that Bowie/Jagger song about dancing:
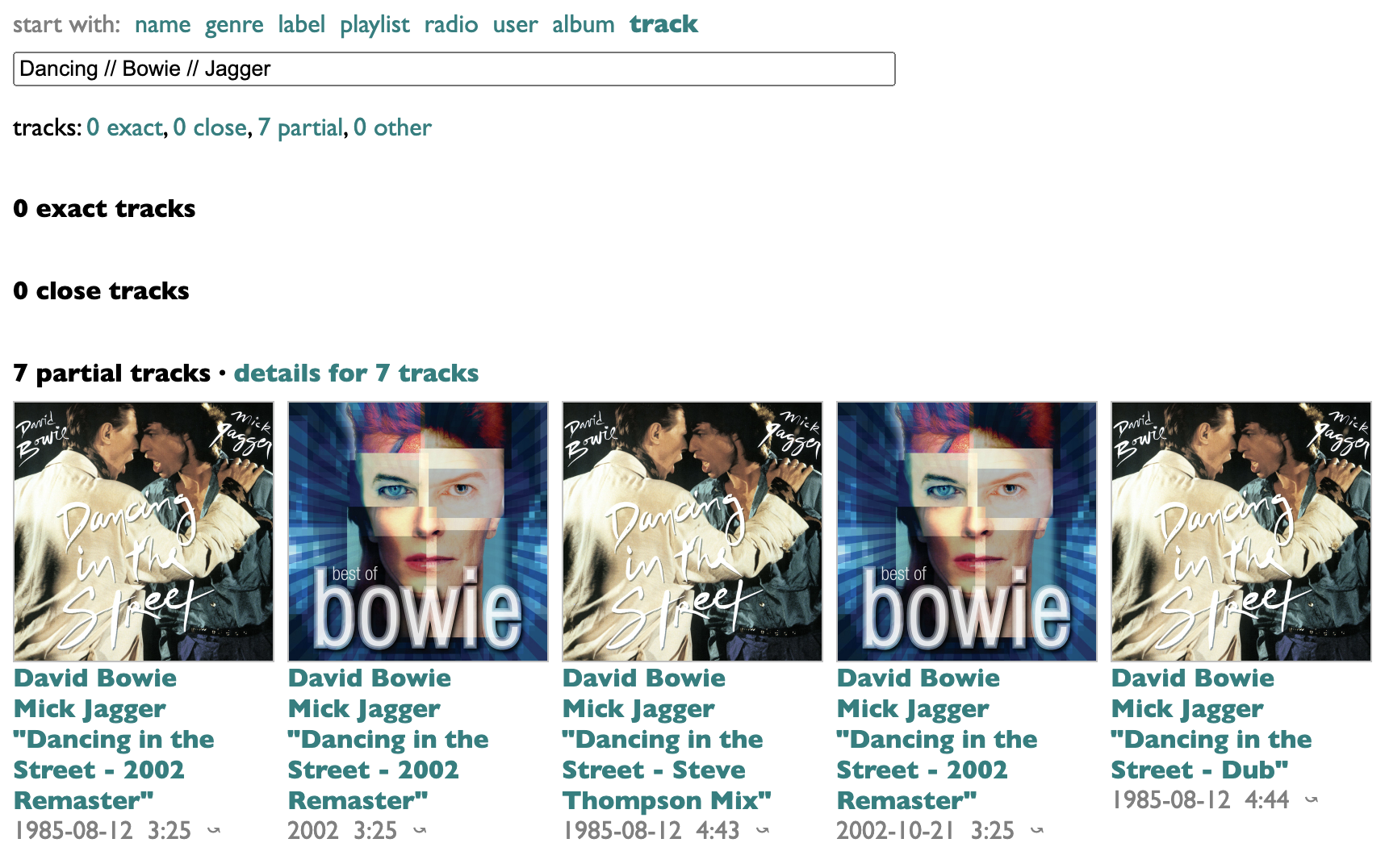
To be fair, you could find that, plus some bonus Björk, with normal searching:
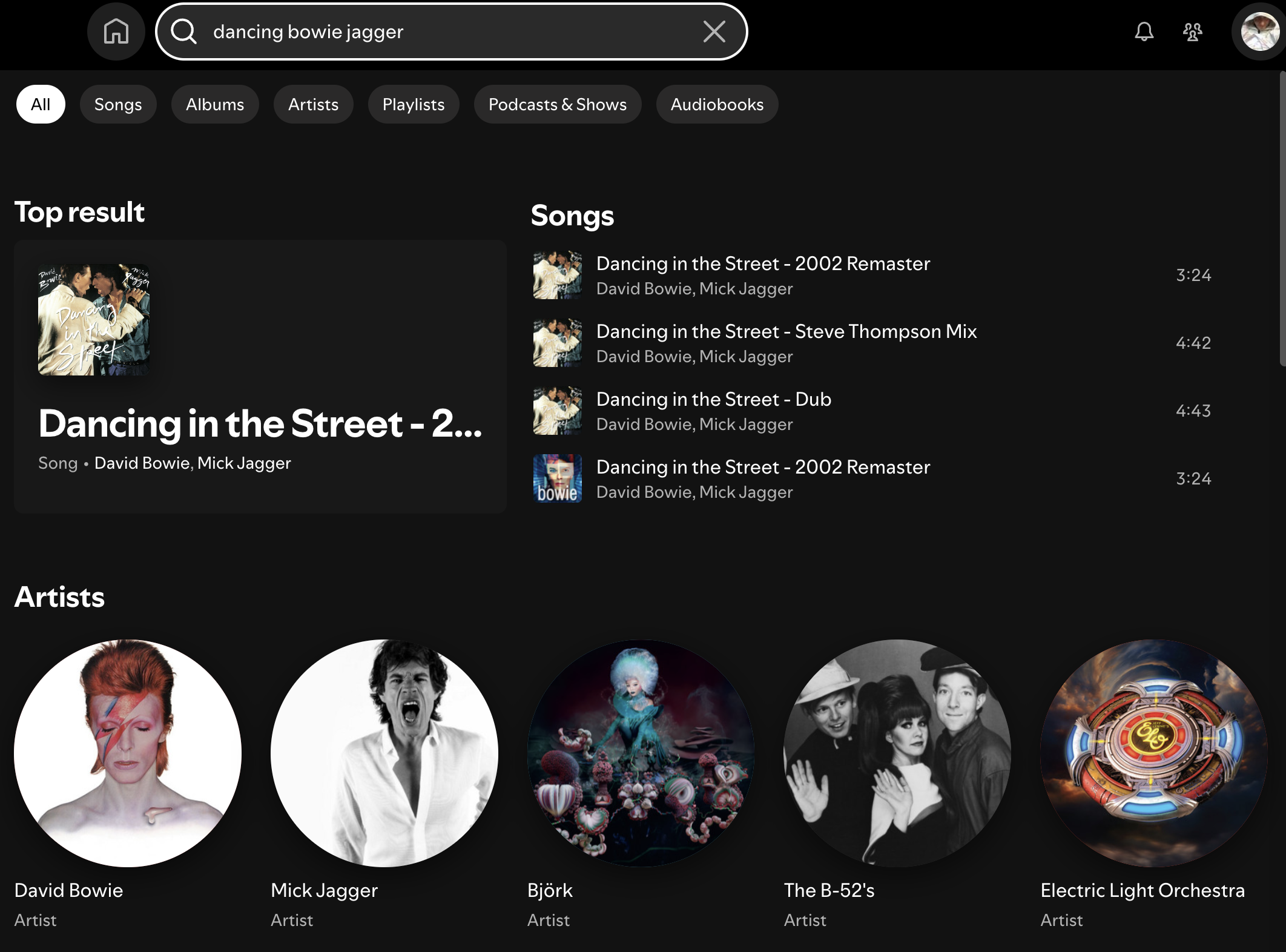
But my version has a wildcard filter so we can find all of Bowie's collaborations:
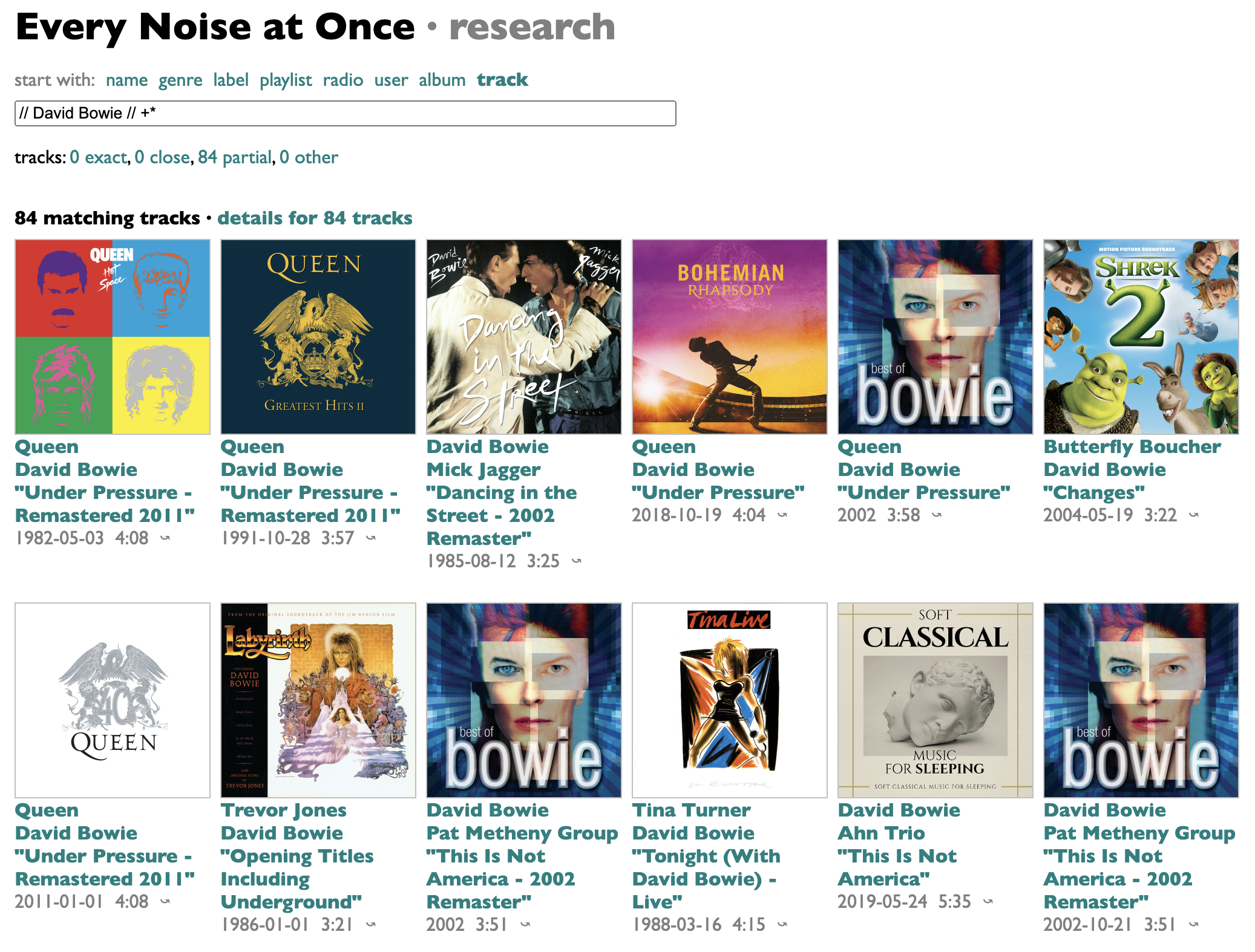
And even deduplicate them by song:
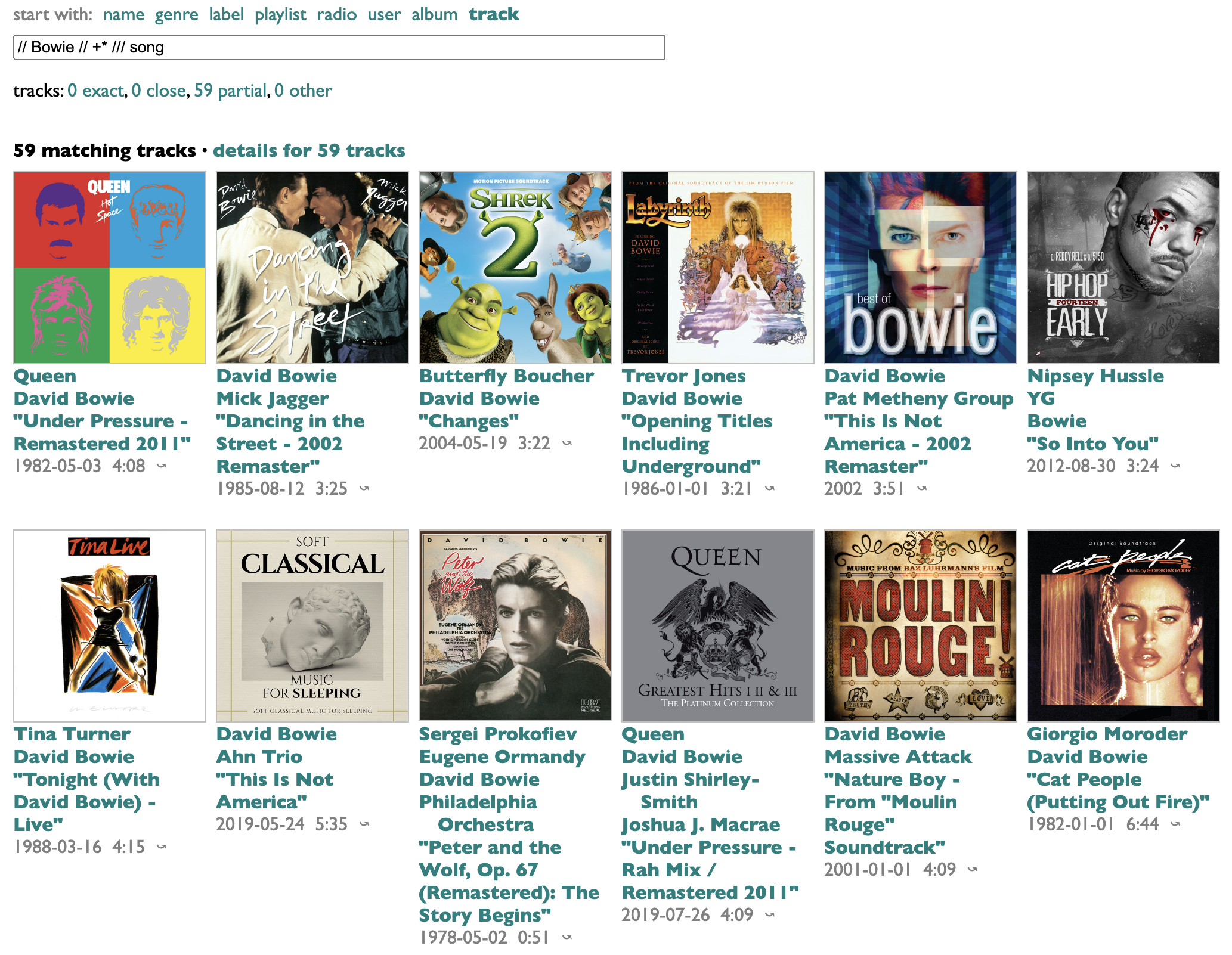
Or all the Ghetts tracks with no guests:
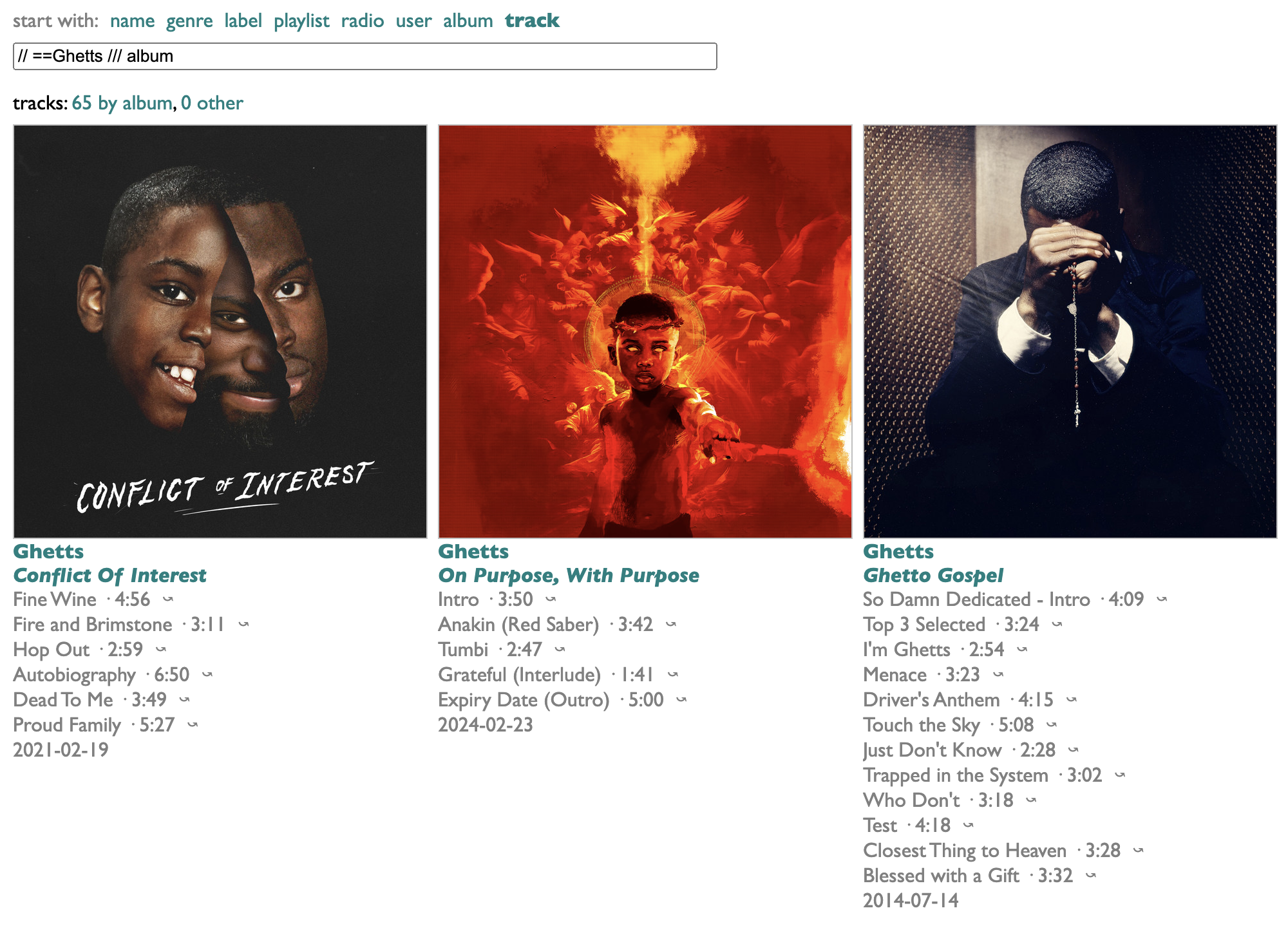
Or all the non-CHVRCHES songs featuring CHVRCHES:
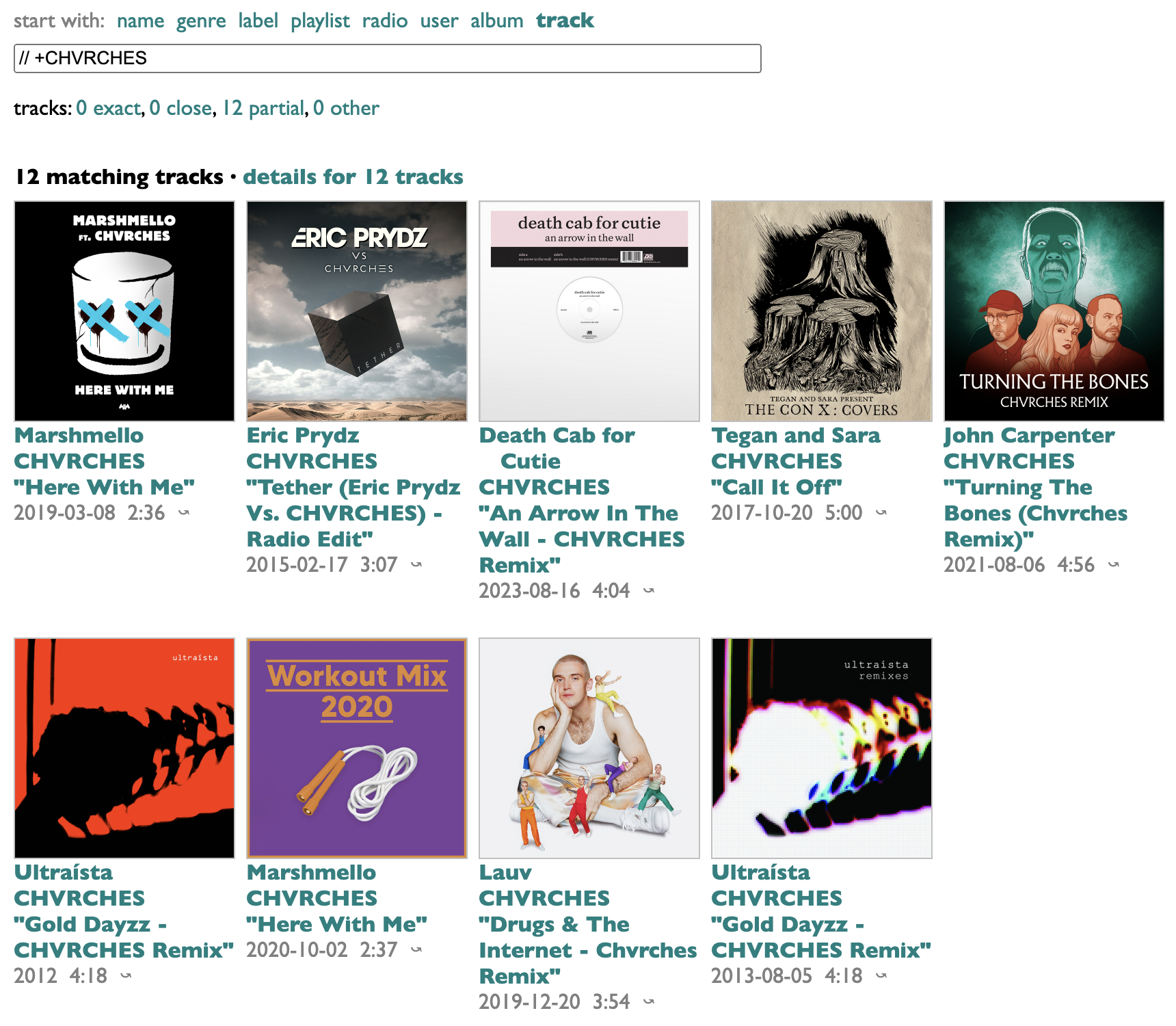
It's not complicated. We don't need AI for this.
(Which is good, because the AI is, maybe, not quite ready...)
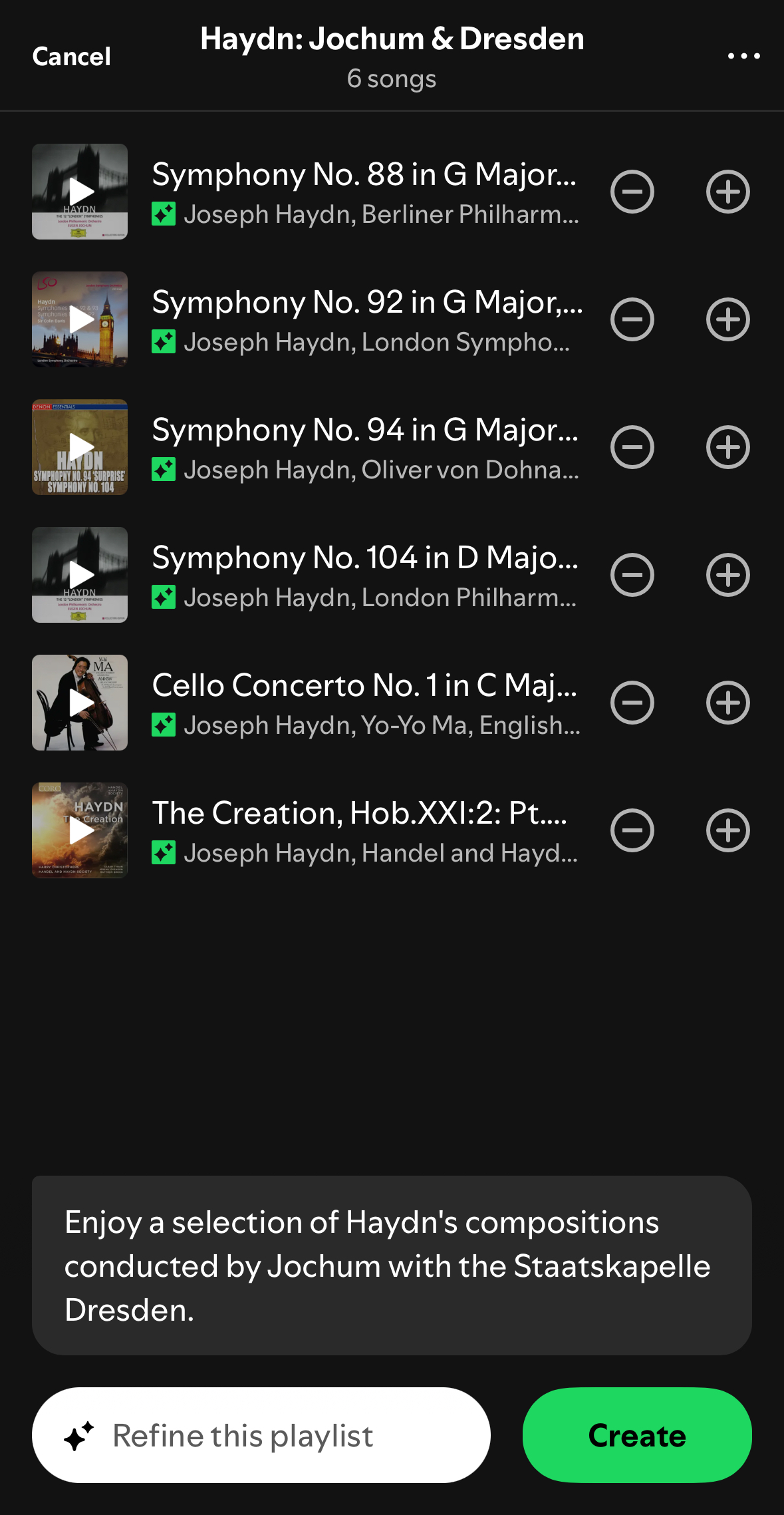
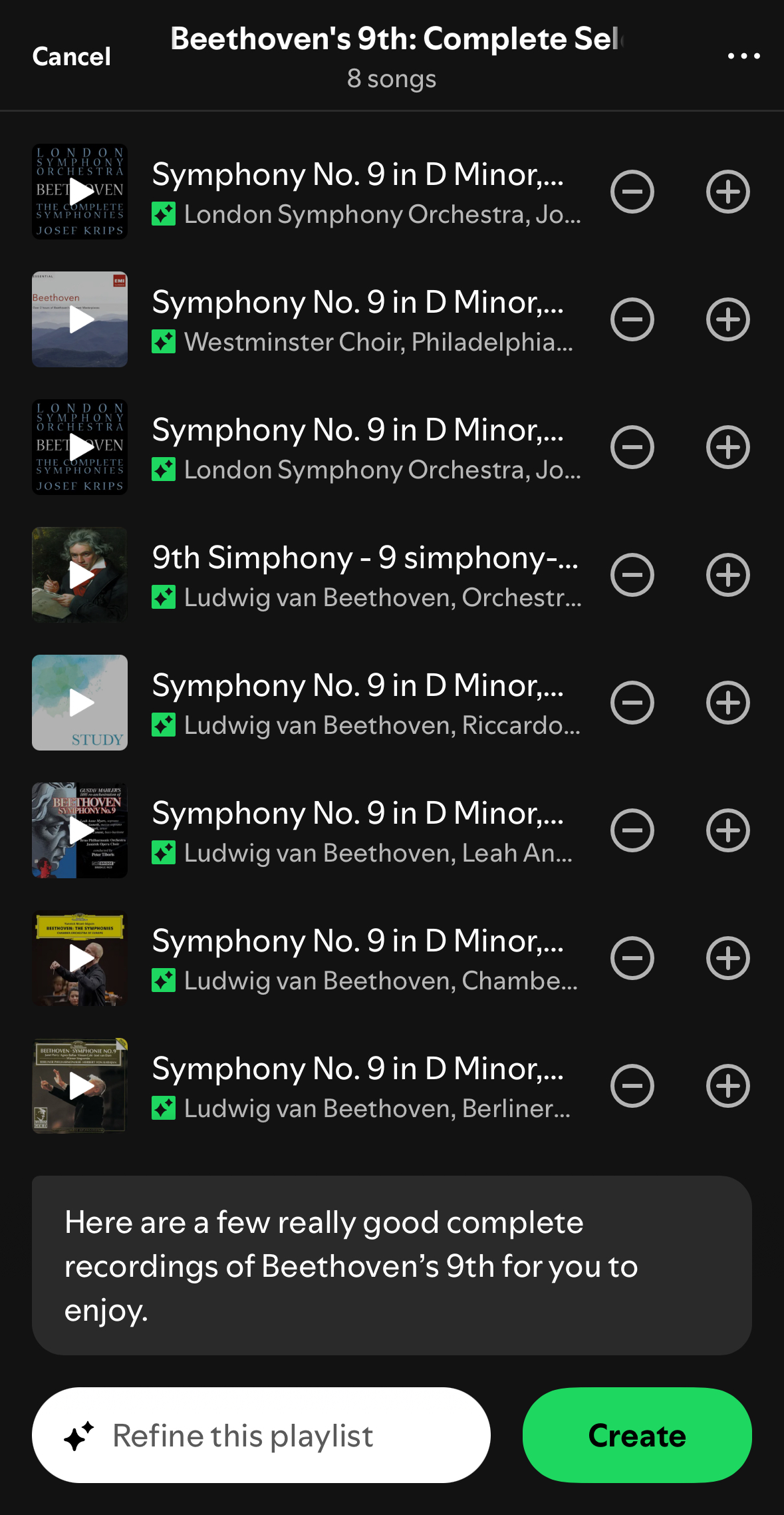
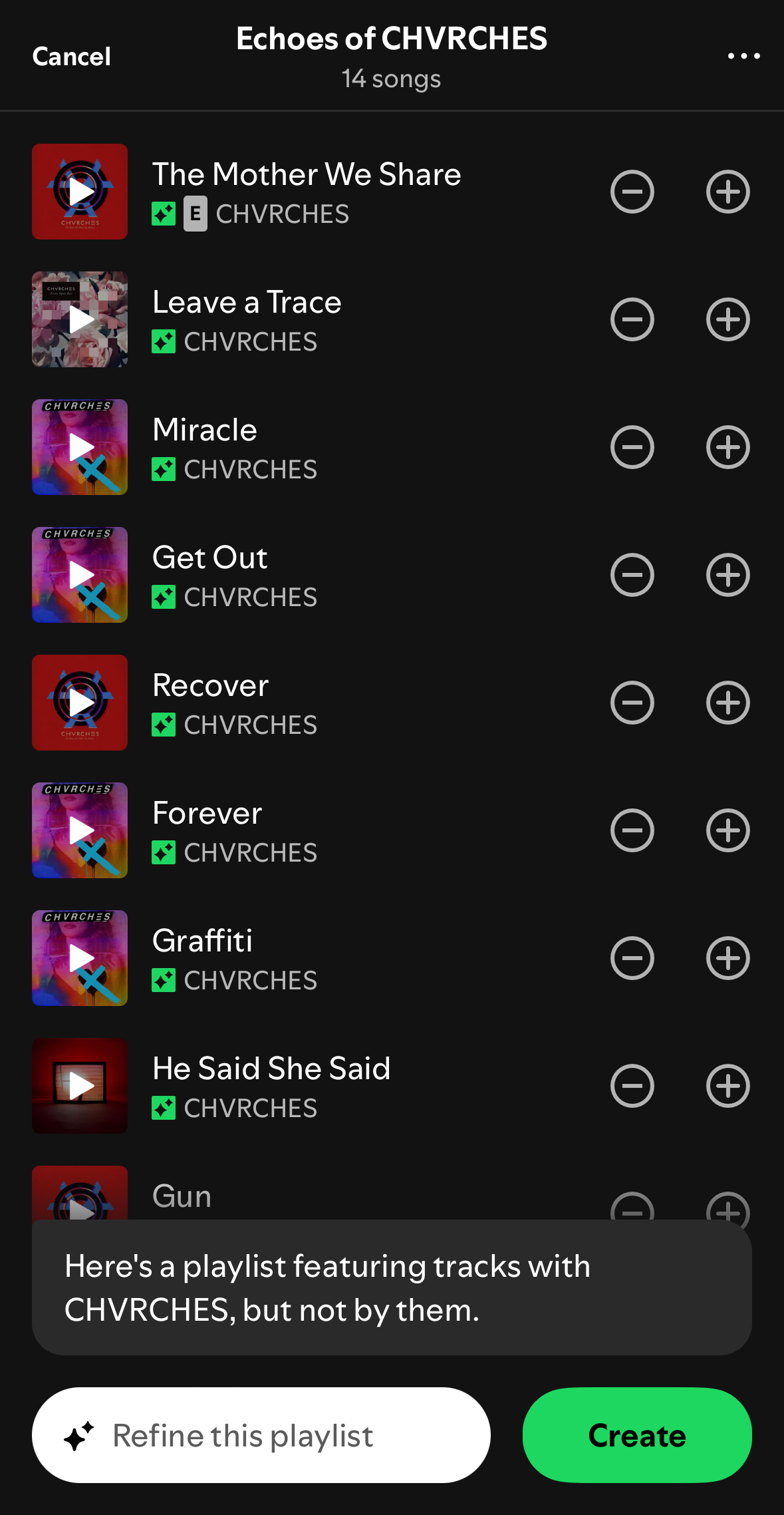
¶ New Releases by Genre: the comeback begins · 28 July 2024 listen/tech
Spotify killed the New Releases by Genre function of Every Noise at Once when they laid me off and cut off my website from its internal data sources. As I've described previously, the fact that a functional new-release tool required internal data-access, to begin with, was a result of minor structural contingencies, not conceptual or business objections, but in 10 years of working at Spotify I do not remember ever successfully persuading the API team to change a feature. If we're going to get NRbG back, we're going to have to figure out how to rebuild it with the tools we are allowed.
But since I need NRbG myself, emotionally not just logistically, I've kept experimenting with ways of recreating it. It didn't actually take me very long to build a personal version of it. Spotify still does have the best music-service API, by far, and the brute-force approach of searching for artists by genre, and then checking the catalogs of each of those artists one-by-one for new releases every week, does basically work. It just doesn't scale. I'm willing to wait a few minutes for the things I care about the most; it doesn't work to make everybody wait for everything anybody cares about. When I worked at Spotify, I could try to solve some problems for everybody at once; from outside, I am too constrained by API rate limits.
The code I wrote, however, would work for you as readily as for me. Even my "personal" tools are general-purpose, because I assume I'll be curious tomorrow about something I didn't care about today. Maybe it's more accurate to say that I tend to build tools to extend my curiosity as much as to satisfy it, or that extending and satisfying describe a propulsive cycle of curiosity more than alternative goals. I would love to inspire this same kind of curiosity in you, but I would settle for giving you some power and letting you discover what you do with it.
And a few days ago it occurred to me that I can. Or, rather, I can give you the power of my knowledge and experience embodied in code, and you can get the power of running it for yourself by signing up for your own API keys. Which is easy and free.
Here's how:
- go to developer.spotify.com
- click "Log in", and log into your regular Spotify account
- click your name in the top right, and pick Dashboard
- read and accept the developer terms of service
- on the Dashboard page, click "Create app" in the top right
-- App name: NRbG
-- App description: New Releases by Genre
-- Website: (leave blank)
-- Redirect URIs: localhost (NRbG doesn't actually use this)
-- [x] Web API (leave the others unchecked)
-- [x] I understand and agree etc.
- click Save
- on your new NRbG app page, click Settings in the top right
- click "View client secret"
- copy your "Client ID" and "Client secret"
- go to NRbG (DIY version)
- paste your client ID and secret into the boxes
- hit Enter
Now you have power.
The new version of NRbG is a little different from the old one. Instead of a list of all the genres in the world, it has a text box. Type a genre name there and hit Enter, and it will start looking for new releases by artists in or around that genre that came out in the last release week (from Saturday through Friday, because Friday is the traditional music-industry release day).
After a while it might start finding some.
The orange letters are the first letters of each song-title, and you can click on them to hear samples. If a new release has songs that already came out some other way, they will (usually) be grayed out here, like with the gray S above for the advance single "Sekuyiso Isikhathi" from THANDAZANI's album Sasibaningi.
If you click "show track URIs", at the bottom, you'll get a list of the URIs for all the new tracks from the releases you have checked in the list, which you can copy and paste into a blank (or existing) Spotify playlist (using command-C, command-V in the Spotify desktop app). There's also a "save playlist" option, which create a new playlist for you directly if you want.
Because I built this for myself, there are a few non-obvious features.
The text box actually takes a list of things, separated by + signs, and the things can each be any of these:
- a genre (e.g. maskandi or gothic symphonic metal)
- a Spotify artist link/URI
- the name of an individual artist, in quotes, like "Nightwish", although this will find the most popular with that name, so URIs are always safer
- a Spotify playlist link/URI, to be interpreted as a list of artists
- @ and then the name of a record label (e.g. @Profound Lore; the spelling has to be exactly right, but see the note later about playlists)
If your list starts with a +, the results will be added to the bottom of the current list; otherwise the current results will be replaced.
The ">>" link encodes your current parameters, so if you click that, you can then bookmark the resulting URL for reuse.
New releases for selected labels, because labels are the only thing that works properly in new-release API searching, are each shown individually, in labeled groups. Everything else in a given list is combined to make a consolidated set of artists, those artists are then checked for their closest related artists (via Fans Also Like), and the whole thus-expanded list of artists is reordered by collective relevance and then checked individually in order for new releases.
If you don't know the exact genre names you want, offhand, you can also just type a partial name and an asterisk, like metal*, and it will give you a list of all the genre names that include that word. Or you could go to everynoise.com and type an artist name into the search box in the top right to see what genres they belong to.
The words "new" and "releases", in "new releases by genre" at the top, are both actually mode controls. "new" switches back and forth between "new", for new-release mode, and "top", for one-top-release-per-artist sampler mode, not constrained by dates. "releases" cycles through "releases" (everything), "albums" (no singles or compilations) and "singles" (no albums or compilations).
If you want to get only 1 track per release, for sampler purposes, you can put "1/" before your list. Or indeed any number and then a "/". This will pick the most popular however-many tracks on each release, and gray out the rest (and exclude them from the URI list) like the non-new tracks on new releases.
You might notice that this app, although it requires your API keys, does not itself log into your Spotify account. This is intentional. Many Spotify features are personalized for you in complicated ways, if you are logged in, and for exploratory purposes we don't want that. This means, too, that this app cannot access or modify your personal information. But if you want to control its behavior by giving it more information, it can look up non-private playlists, so that's the mechanism.
If you use a playlist as input (yours or anybody else's), it will look for new releases by the primary artists of the tracks in that playlist and their related artists, but excluding the specific releases already in the playlist. So if you, like me, spend a lot of time using this tool every Friday to make a playlist of new releases you want to hear, you can put that playlist's URI back into the same tool and it will check to see if there's anything else related that you might have missed.
In addition, once you've set up your API keys and NRbG is working, the playlist-profile page in the everynoise research tools also gets a couple added features for finding new releases. Put a playlist link or URI into that view, and it already shows you genres and record labels for every track in the list. But scroll to the bottom of the page, and you'll also see something like this:
The "see new releases" line gives you three links to NRbG for different ways of expanding on this list, each with a set of parameters pre-filled from the data in this playlist.
And, for one last bonus feature, you can check an earlier week by putting that week's Friday date (in YYYY-MM-DD format) at the beginning of your input as an override, like this:
and hit Enter to get:
You can even check whole years by including just a year, although be warned, in both cases, that release-date data gets unreliable pretty quickly once you go back beyond the very recent past.
I don't know what else I'll do with this. Probably more, because it's fun. Feedback, error reports and ideas are all welcome, in the meantime.
See what you find.
But since I need NRbG myself, emotionally not just logistically, I've kept experimenting with ways of recreating it. It didn't actually take me very long to build a personal version of it. Spotify still does have the best music-service API, by far, and the brute-force approach of searching for artists by genre, and then checking the catalogs of each of those artists one-by-one for new releases every week, does basically work. It just doesn't scale. I'm willing to wait a few minutes for the things I care about the most; it doesn't work to make everybody wait for everything anybody cares about. When I worked at Spotify, I could try to solve some problems for everybody at once; from outside, I am too constrained by API rate limits.
The code I wrote, however, would work for you as readily as for me. Even my "personal" tools are general-purpose, because I assume I'll be curious tomorrow about something I didn't care about today. Maybe it's more accurate to say that I tend to build tools to extend my curiosity as much as to satisfy it, or that extending and satisfying describe a propulsive cycle of curiosity more than alternative goals. I would love to inspire this same kind of curiosity in you, but I would settle for giving you some power and letting you discover what you do with it.
And a few days ago it occurred to me that I can. Or, rather, I can give you the power of my knowledge and experience embodied in code, and you can get the power of running it for yourself by signing up for your own API keys. Which is easy and free.
Here's how:
- go to developer.spotify.com
- click "Log in", and log into your regular Spotify account
- click your name in the top right, and pick Dashboard
- read and accept the developer terms of service
- on the Dashboard page, click "Create app" in the top right
-- App name: NRbG
-- App description: New Releases by Genre
-- Website: (leave blank)
-- Redirect URIs: localhost (NRbG doesn't actually use this)
-- [x] Web API (leave the others unchecked)
-- [x] I understand and agree etc.
- click Save
- on your new NRbG app page, click Settings in the top right
- click "View client secret"
- copy your "Client ID" and "Client secret"
- go to NRbG (DIY version)
- paste your client ID and secret into the boxes
- hit Enter
Now you have power.
The new version of NRbG is a little different from the old one. Instead of a list of all the genres in the world, it has a text box. Type a genre name there and hit Enter, and it will start looking for new releases by artists in or around that genre that came out in the last release week (from Saturday through Friday, because Friday is the traditional music-industry release day).

After a while it might start finding some.
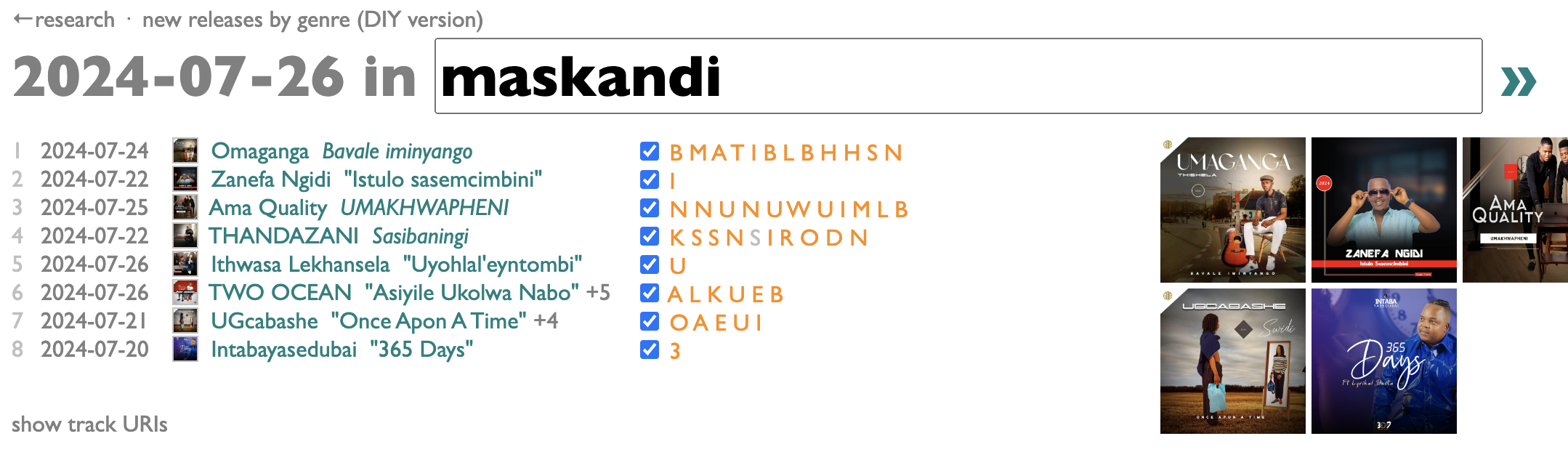
The orange letters are the first letters of each song-title, and you can click on them to hear samples. If a new release has songs that already came out some other way, they will (usually) be grayed out here, like with the gray S above for the advance single "Sekuyiso Isikhathi" from THANDAZANI's album Sasibaningi.
If you click "show track URIs", at the bottom, you'll get a list of the URIs for all the new tracks from the releases you have checked in the list, which you can copy and paste into a blank (or existing) Spotify playlist (using command-C, command-V in the Spotify desktop app). There's also a "save playlist" option, which create a new playlist for you directly if you want.
Because I built this for myself, there are a few non-obvious features.
The text box actually takes a list of things, separated by + signs, and the things can each be any of these:
- a genre (e.g. maskandi or gothic symphonic metal)
- a Spotify artist link/URI
- the name of an individual artist, in quotes, like "Nightwish", although this will find the most popular with that name, so URIs are always safer
- a Spotify playlist link/URI, to be interpreted as a list of artists
- @ and then the name of a record label (e.g. @Profound Lore; the spelling has to be exactly right, but see the note later about playlists)
If your list starts with a +, the results will be added to the bottom of the current list; otherwise the current results will be replaced.
The ">>" link encodes your current parameters, so if you click that, you can then bookmark the resulting URL for reuse.
New releases for selected labels, because labels are the only thing that works properly in new-release API searching, are each shown individually, in labeled groups. Everything else in a given list is combined to make a consolidated set of artists, those artists are then checked for their closest related artists (via Fans Also Like), and the whole thus-expanded list of artists is reordered by collective relevance and then checked individually in order for new releases.
If you don't know the exact genre names you want, offhand, you can also just type a partial name and an asterisk, like metal*, and it will give you a list of all the genre names that include that word. Or you could go to everynoise.com and type an artist name into the search box in the top right to see what genres they belong to.
The words "new" and "releases", in "new releases by genre" at the top, are both actually mode controls. "new" switches back and forth between "new", for new-release mode, and "top", for one-top-release-per-artist sampler mode, not constrained by dates. "releases" cycles through "releases" (everything), "albums" (no singles or compilations) and "singles" (no albums or compilations).
If you want to get only 1 track per release, for sampler purposes, you can put "1/" before your list. Or indeed any number and then a "/". This will pick the most popular however-many tracks on each release, and gray out the rest (and exclude them from the URI list) like the non-new tracks on new releases.
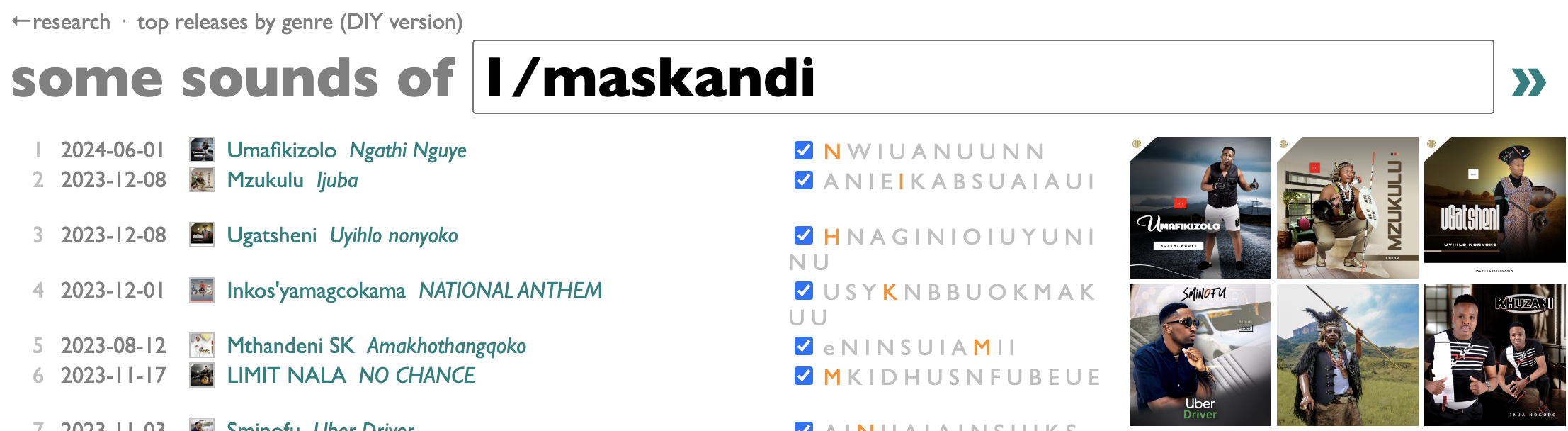
You might notice that this app, although it requires your API keys, does not itself log into your Spotify account. This is intentional. Many Spotify features are personalized for you in complicated ways, if you are logged in, and for exploratory purposes we don't want that. This means, too, that this app cannot access or modify your personal information. But if you want to control its behavior by giving it more information, it can look up non-private playlists, so that's the mechanism.
If you use a playlist as input (yours or anybody else's), it will look for new releases by the primary artists of the tracks in that playlist and their related artists, but excluding the specific releases already in the playlist. So if you, like me, spend a lot of time using this tool every Friday to make a playlist of new releases you want to hear, you can put that playlist's URI back into the same tool and it will check to see if there's anything else related that you might have missed.
In addition, once you've set up your API keys and NRbG is working, the playlist-profile page in the everynoise research tools also gets a couple added features for finding new releases. Put a playlist link or URI into that view, and it already shows you genres and record labels for every track in the list. But scroll to the bottom of the page, and you'll also see something like this:
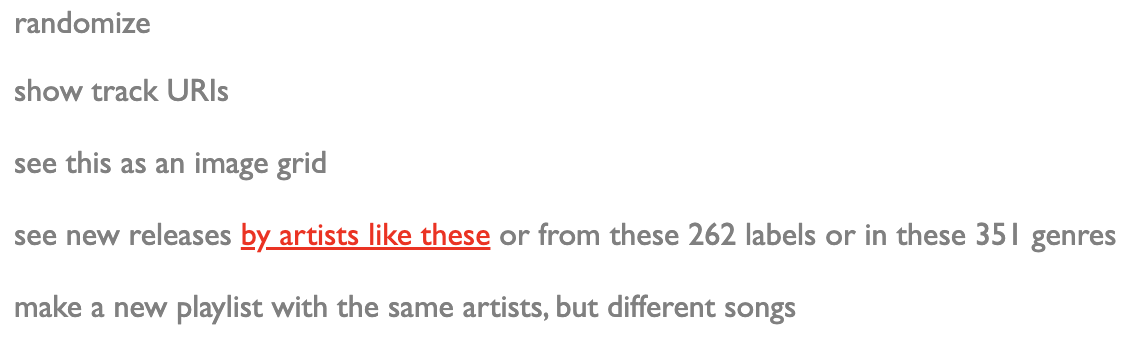
The "see new releases" line gives you three links to NRbG for different ways of expanding on this list, each with a set of parameters pre-filled from the data in this playlist.
And, for one last bonus feature, you can check an earlier week by putting that week's Friday date (in YYYY-MM-DD format) at the beginning of your input as an override, like this:

and hit Enter to get:
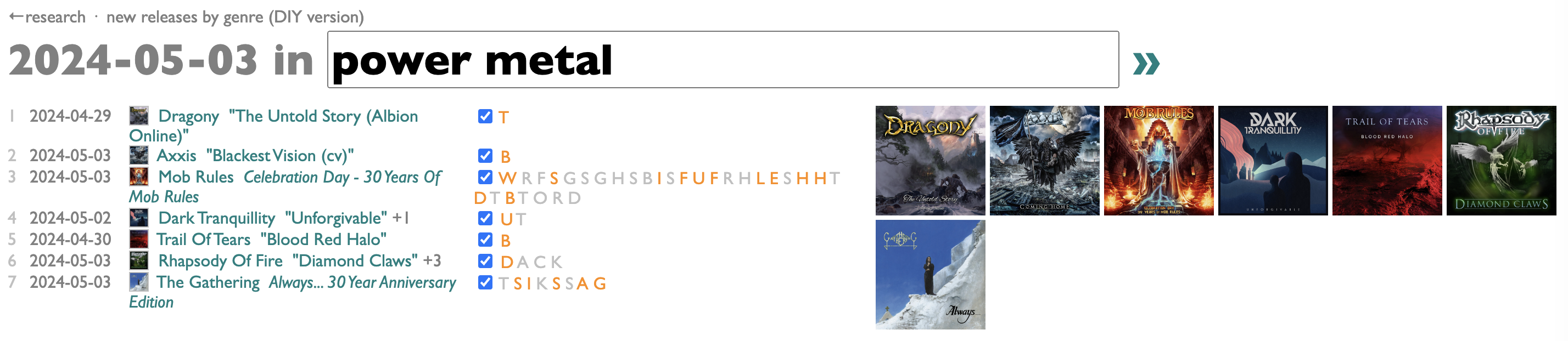
You can even check whole years by including just a year, although be warned, in both cases, that release-date data gets unreliable pretty quickly once you go back beyond the very recent past.
I don't know what else I'll do with this. Probably more, because it's fun. Feedback, error reports and ideas are all welcome, in the meantime.
See what you find.
¶ Corners of the world · 25 July 2024 listen/tech
I'm keeping a running list of book-related media links at the bottom of this post, but here are a few new things from an interestingly global week:
- I'm featured in an article about AI and the future in the French magazine Usbek & Rica this month. My copy hasn't arrvied yet, and I think it's in French, so I'm as curious as anybody what I said.
- Iveta Hajdakova and Tom Hoy at the London international consulting group Stripe Partners, who I know from some work they did for Spotify while I was there, interviewed me about algorithms and music for their Viewpoints series.
- There's an interview/feature with/about me and You Have Not Yet Heard Your Favourite Song both in print and online in the Polish magazine Polityka.
- I'll be making my second ever visit to the southern hemisphere, and first to New Zealand and Australia, to appear in conversation at Going Global Music Summit 2024 in Auckland, August 29-30, and then BIGSOUND 2024 in Brisbane, September 2-6!
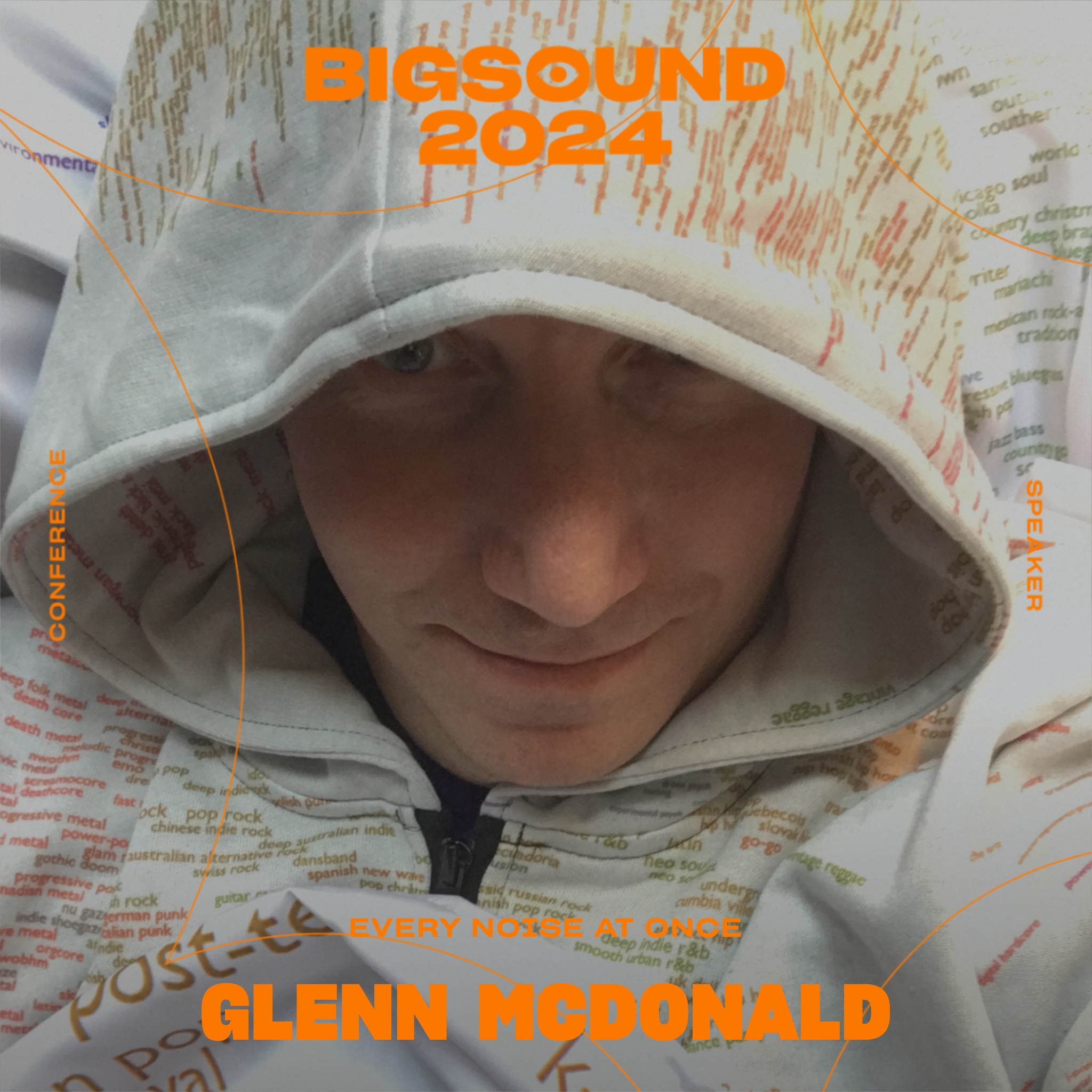
- I'm featured in an article about AI and the future in the French magazine Usbek & Rica this month. My copy hasn't arrvied yet, and I think it's in French, so I'm as curious as anybody what I said.
- Iveta Hajdakova and Tom Hoy at the London international consulting group Stripe Partners, who I know from some work they did for Spotify while I was there, interviewed me about algorithms and music for their Viewpoints series.
- There's an interview/feature with/about me and You Have Not Yet Heard Your Favourite Song both in print and online in the Polish magazine Polityka.
- I'll be making my second ever visit to the southern hemisphere, and first to New Zealand and Australia, to appear in conversation at Going Global Music Summit 2024 in Auckland, August 29-30, and then BIGSOUND 2024 in Brisbane, September 2-6!
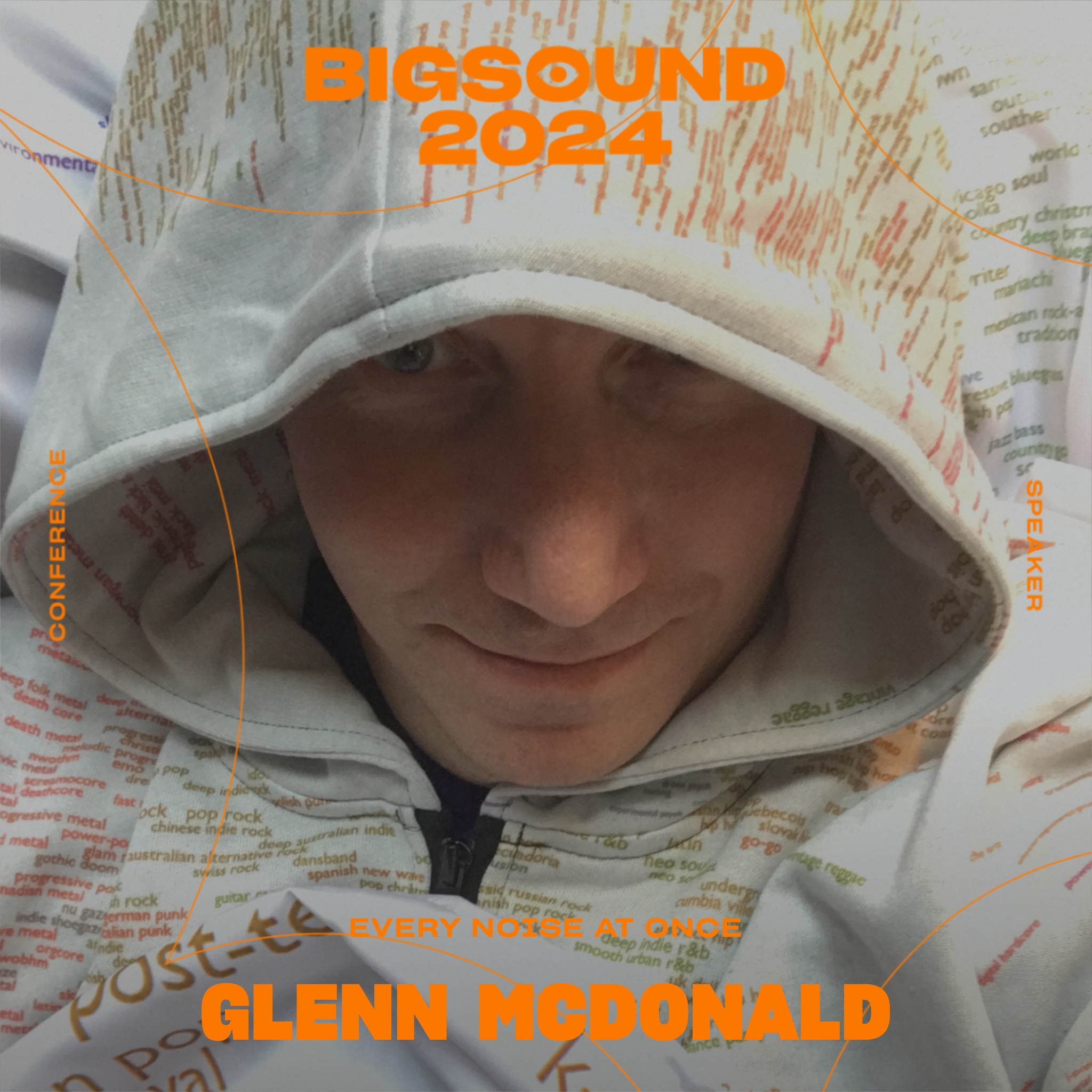
You Have Not Yet Heard Your Favourite Song began in a Google doc I had provisionally titled Every Noise at Once: New Fears and New Joys From the Streaming Liberation of All the World's Music. The first chapter-list I made there was mostly just a chronological free-association of stories I tell. That structure was useful in suggesting that there was probably a book's quantity of words involved, but when we started sending this outline and a few sample chapters to publishers, the fairly unanimous response was that I was not famous enough for strangers to want to read about my adventures in music just because I had them.
I couldn't really argue with that, nor did I actually want to write a memoir. I wanted to try to formulate an argument. Not an entirely straightforward or linear argument, exactly, but still, something more like an essay than a memoir: not every noise, and not at once, but particular noises, in an order with progressions and resolutions.
Restructuring the list of stories into the outline of an essay was easy and encouraging, and a couple of the chapters I had written already nearly fit the new structure, but the first version's first chapter was quite clearly the first chapter of a memoir, and I just deleted it and started over.
And by "deleted", I mean that I copied it into a different doc in case I thought of some other use for it later. I just came across that doc again, and blogs solve the not-famous-enough problem by not asking anybody but you to care whether you care. So here, for no other reason than that I wrote it once, is that original first chapter of the memoir I didn't write:
I couldn't really argue with that, nor did I actually want to write a memoir. I wanted to try to formulate an argument. Not an entirely straightforward or linear argument, exactly, but still, something more like an essay than a memoir: not every noise, and not at once, but particular noises, in an order with progressions and resolutions.
Restructuring the list of stories into the outline of an essay was easy and encouraging, and a couple of the chapters I had written already nearly fit the new structure, but the first version's first chapter was quite clearly the first chapter of a memoir, and I just deleted it and started over.
And by "deleted", I mean that I copied it into a different doc in case I thought of some other use for it later. I just came across that doc again, and blogs solve the not-famous-enough problem by not asking anybody but you to care whether you care. So here, for no other reason than that I wrote it once, is that original first chapter of the memoir I didn't write:
Chapter 1: Precious Jukeboxes
Without music, I would not even exist.
This is probably true in some existential or logistic way for a lot of people, but music is literally how my parents met. They were folk-singing in New Haven, Connecticut, in 1963. My mother had a duo with a friend. My father was in a Peter, Paul & Mary-esque trio. My father was my mother's guitar-teacher before either of them realized they were my parents.
After they got married they moved to Texas, where my father came from, and there I grew up, with their records and their acoustic guitars. We begin with our parents' music. In my case this was a stereo in a cabinet in our living room and a small collection of LPs, mostly folk standards like The Weavers and The Kingston Trio, and some of their 70s successors from the gentle margins of pop, like Joan Baez and Judy Collins and John Denver. My mother had some Ray Charles and Dave Brubeck records from when she wrote jazz reviews for her school paper, and Eddie Fisher records from her days in his Fan Club. They did buy new records, occasionally, but rarely by new artists. They loved and valued music, but without any sense of frantic urgency or gnawing incompleteness. And thus, if you'd tried to categorize recorded music as an economic activity based on their music spending, you would probably have put it in the same tier with handmade ceramics or gardening trowels.
For popular music, or really anything except my parents' records, there was the car radio. My parents were exactly the right demographic for NPR, but mercifully the wrong personality types for talk-radio, so from the back seat I got to hear Casey Kasem or whatever shuffled loop of the top 40 was playing during the week in between canonical countdowns.
Looking over the charts from my childhood years, I find that by the 1976 chart I recognize almost everything. That was the year I was given my first record-player of my own, and the store where my father bought it foisted two 7" singles on him as part of the deal, which he dutifully passed along to me, probably without having listened to them first: the Starland Vocal Band's frankly inappropriate "Afternoon Delight" and Walter Murphy's ridiculously discofied "A Fifth of Beethoven". I would happily listen to either of these songs again if for some reason I was once again imprisoned in a musical void where no other songs existed.
But my emergent taste wasn't much more adventurous than those. The first record I bought with my own money, which of course is a milestone that dates me by the fact that it's a milestone, was the Eagles' Hotel California. My record-budget was such that even a single LP was a serious investment subject to a ruthless risk-assessment that realistically required me to already have heard and loved at least four of its songs, and the only place I would have heard them was on the car radio. The viable size of the music industry, at least judged by its access to the record budget of an average American 10-year-old, was probably measured in dozens of artists.
One day, on that radio, I heard "Hold the Line", by Toto. Heard with any kind of perspective, Toto is the softest of soft-rock bands, and the maudlin, patronizing "Africa" has more fittingly become their limp sigil. But about 10 seconds into "Hold the Line" there's a power chord. It is not the first mainstream pop hit with a power chord, but somehow it was the first one I heard. It sounded like a difference engine, to me, or a dragon made out of moonlight, or some kind of god tearing the universe open along a revealed seam. The album cover has a sword. That sword sliced through my world.
I am fully aware that writing an origin-myth for a life-long obsession with heavy metal that begins with "Hold the Line" is like saying your love of Thai food began with a wide noodle dipped in Pop Rocks. But that's how it began, for me, and in my world in 1978, that's kind of the only way anything ever began. One sound can change your life. Toto led me towards Foreigner and Bad Company and Boston. Not via fast clicks, because there was no Fans Also Like to navigate through, but over the excruciating course of radio months and tentative spending. In 1979 I got my first radio, a flip-number clock radio with a single tiny speaker and no headphone jack, so I would turn the volume knob all the way down and then press my ear to it in order to listen to The Great American Radio Show after my supposed bedtime. I found the two Album Rock stations my parents never played in the car. Toto led me to Boston, which got me to Kansas and Supertramp, and then to Rush. Give 12-year-olds a radio of their own, and maybe you can have hundreds of artists instead of dozens.
Meanwhile, reading Michael Moorcock books got me to his lyric-writing for Hawkwind, and then Blue Öyster Cult, who were the first band I ever saw in concert. The Moorcock-co-written Blue Öyster Cult song "Veteran of the Psychic Wars" also appeared on the soundtrack for the 1981 movie Heavy Metal, and that soundtrack also had "The Mob Rules", by Black Sabbath.
I definitely hadn't heard that on the radio. "The Mob Rules" starts with that guitar noise from the very first moment, churning, relentless. Ronnie James Dio howls demonically, not like a halloween-costume devil but like an exiled lord of a forsaken realm. Cymbals start crashing like the night sky is the sun coming apart into shards. If pop was about melody, disco was about movement and rock was about energy, then metal was about power. Not about the ends of power or its victims, but about the visceral feeling of wielding it, of how it runs through you, of how it makes you want more.
Thus began my record-collecting life, in earnest, as a quest to find out what else the radio wasn't telling me about, and how else it could make me feel. But it was so hard to begin, when every step required all of your budget, and knowledge you mostly didn't have. Jukeboxes say 25¢ on them, which sounds cheap, but knowing what songs to play cost more than coins, and knowing what songs weren't in them didn't even have a coin slot. Music discovery, thus, was still barely a thing. Or it was, but as if Ferdinand Magellan had travelled from Portugal to Spain to receive a royal commission to go back and discover Portugal again.
And even if I had somehow discovered the shores of a new world, what would I have done? I'd heard "Dancing Queen", I knew ABBA were from Sweden, I knew roughly where that was. I didn't know it would later become a specifically important part of my life, but I'm sure I would have assumed there were more people making music there than just ABBA, if it had occurred to me to entertain the question.
But so what? There were no other Swedish pop songs on the radio. There were no other Swedish records in the record stores. There were no magazines about Swedish music at the drugstore, or books about it in the library. None of my friends knew anything else about Swedish music than ABBA, either. To learn about Gräs och Stenar, or Nationalteatern, or Gyllene Tider, all I would have been able to do was wait, patiently, to become old enough to get a job to save up for a plane ticket to Stockholm, where I would have had to find a telephone directory and figure out how to look up where the record stores were, and then go hope somebody in one of them spoke English and was willing to explain Swedish pop history to a random kid from Texas.
The world was full of music. But I was standing in the same record stores, flipping through the same few mute sealed packages over and over, wondering what was inside them and what was missing, wanting to know.
Without music, I would not even exist.
This is probably true in some existential or logistic way for a lot of people, but music is literally how my parents met. They were folk-singing in New Haven, Connecticut, in 1963. My mother had a duo with a friend. My father was in a Peter, Paul & Mary-esque trio. My father was my mother's guitar-teacher before either of them realized they were my parents.
After they got married they moved to Texas, where my father came from, and there I grew up, with their records and their acoustic guitars. We begin with our parents' music. In my case this was a stereo in a cabinet in our living room and a small collection of LPs, mostly folk standards like The Weavers and The Kingston Trio, and some of their 70s successors from the gentle margins of pop, like Joan Baez and Judy Collins and John Denver. My mother had some Ray Charles and Dave Brubeck records from when she wrote jazz reviews for her school paper, and Eddie Fisher records from her days in his Fan Club. They did buy new records, occasionally, but rarely by new artists. They loved and valued music, but without any sense of frantic urgency or gnawing incompleteness. And thus, if you'd tried to categorize recorded music as an economic activity based on their music spending, you would probably have put it in the same tier with handmade ceramics or gardening trowels.
For popular music, or really anything except my parents' records, there was the car radio. My parents were exactly the right demographic for NPR, but mercifully the wrong personality types for talk-radio, so from the back seat I got to hear Casey Kasem or whatever shuffled loop of the top 40 was playing during the week in between canonical countdowns.
Looking over the charts from my childhood years, I find that by the 1976 chart I recognize almost everything. That was the year I was given my first record-player of my own, and the store where my father bought it foisted two 7" singles on him as part of the deal, which he dutifully passed along to me, probably without having listened to them first: the Starland Vocal Band's frankly inappropriate "Afternoon Delight" and Walter Murphy's ridiculously discofied "A Fifth of Beethoven". I would happily listen to either of these songs again if for some reason I was once again imprisoned in a musical void where no other songs existed.
But my emergent taste wasn't much more adventurous than those. The first record I bought with my own money, which of course is a milestone that dates me by the fact that it's a milestone, was the Eagles' Hotel California. My record-budget was such that even a single LP was a serious investment subject to a ruthless risk-assessment that realistically required me to already have heard and loved at least four of its songs, and the only place I would have heard them was on the car radio. The viable size of the music industry, at least judged by its access to the record budget of an average American 10-year-old, was probably measured in dozens of artists.
One day, on that radio, I heard "Hold the Line", by Toto. Heard with any kind of perspective, Toto is the softest of soft-rock bands, and the maudlin, patronizing "Africa" has more fittingly become their limp sigil. But about 10 seconds into "Hold the Line" there's a power chord. It is not the first mainstream pop hit with a power chord, but somehow it was the first one I heard. It sounded like a difference engine, to me, or a dragon made out of moonlight, or some kind of god tearing the universe open along a revealed seam. The album cover has a sword. That sword sliced through my world.
I am fully aware that writing an origin-myth for a life-long obsession with heavy metal that begins with "Hold the Line" is like saying your love of Thai food began with a wide noodle dipped in Pop Rocks. But that's how it began, for me, and in my world in 1978, that's kind of the only way anything ever began. One sound can change your life. Toto led me towards Foreigner and Bad Company and Boston. Not via fast clicks, because there was no Fans Also Like to navigate through, but over the excruciating course of radio months and tentative spending. In 1979 I got my first radio, a flip-number clock radio with a single tiny speaker and no headphone jack, so I would turn the volume knob all the way down and then press my ear to it in order to listen to The Great American Radio Show after my supposed bedtime. I found the two Album Rock stations my parents never played in the car. Toto led me to Boston, which got me to Kansas and Supertramp, and then to Rush. Give 12-year-olds a radio of their own, and maybe you can have hundreds of artists instead of dozens.
Meanwhile, reading Michael Moorcock books got me to his lyric-writing for Hawkwind, and then Blue Öyster Cult, who were the first band I ever saw in concert. The Moorcock-co-written Blue Öyster Cult song "Veteran of the Psychic Wars" also appeared on the soundtrack for the 1981 movie Heavy Metal, and that soundtrack also had "The Mob Rules", by Black Sabbath.
I definitely hadn't heard that on the radio. "The Mob Rules" starts with that guitar noise from the very first moment, churning, relentless. Ronnie James Dio howls demonically, not like a halloween-costume devil but like an exiled lord of a forsaken realm. Cymbals start crashing like the night sky is the sun coming apart into shards. If pop was about melody, disco was about movement and rock was about energy, then metal was about power. Not about the ends of power or its victims, but about the visceral feeling of wielding it, of how it runs through you, of how it makes you want more.
Thus began my record-collecting life, in earnest, as a quest to find out what else the radio wasn't telling me about, and how else it could make me feel. But it was so hard to begin, when every step required all of your budget, and knowledge you mostly didn't have. Jukeboxes say 25¢ on them, which sounds cheap, but knowing what songs to play cost more than coins, and knowing what songs weren't in them didn't even have a coin slot. Music discovery, thus, was still barely a thing. Or it was, but as if Ferdinand Magellan had travelled from Portugal to Spain to receive a royal commission to go back and discover Portugal again.
And even if I had somehow discovered the shores of a new world, what would I have done? I'd heard "Dancing Queen", I knew ABBA were from Sweden, I knew roughly where that was. I didn't know it would later become a specifically important part of my life, but I'm sure I would have assumed there were more people making music there than just ABBA, if it had occurred to me to entertain the question.
But so what? There were no other Swedish pop songs on the radio. There were no other Swedish records in the record stores. There were no magazines about Swedish music at the drugstore, or books about it in the library. None of my friends knew anything else about Swedish music than ABBA, either. To learn about Gräs och Stenar, or Nationalteatern, or Gyllene Tider, all I would have been able to do was wait, patiently, to become old enough to get a job to save up for a plane ticket to Stockholm, where I would have had to find a telephone directory and figure out how to look up where the record stores were, and then go hope somebody in one of them spoke English and was willing to explain Swedish pop history to a random kid from Texas.
The world was full of music. But I was standing in the same record stores, flipping through the same few mute sealed packages over and over, wondering what was inside them and what was missing, wanting to know.
Large Language Models, by encoding our language, also encode our beliefs about ourselves, exactly as confused and conflicting as they seem to us when we read them, too. When we ask these models to answer questions, we would like to think we are invoking our most aggregated collective wisdom, but more realistically we are usually eliciting the mean of our conditioned beliefs. We talk to ChatGPT as if it has patiently and lovingly studied us, crystalizing all the latent truths we have half discovered and half suspected. But what we have actually constructed is an erratic analogical model, and the most interesting parts of it are probably the ones in the middle, which are also exactly the ones that LLMs as chatbots are least intent on revealing.
One of the many amazing things ChatGPT can do, though, is describe images in words. Another one of these amazing things it can do is produce images from descriptions.
It's entertaining to chain these two things together. Tell it to describe a picture in precise detail, then give it back that description and tell it to generate that image. Here's a picture of my wife and I eating a casually celebratory dinner:
And here's what image-to-description-to-image turns us into:
It made us younger and hotter, obviously, but the other details are also intriguing. All the drinks have limes, now, and straws pointing right. The one closed umbrella has been rendered canonically open and multiple. Every major detail in the original picture has been transliterated into its paradigmatic, normative form. This process is even more obvious if you keep going a few more iterations, feeding each generated image back into the loop:
We have converged on the LLM equivalent of Platonic Forms: the most soft-taco-like soft tacos, the most people-being-photographed haircuts, the most endlessly elemental green picnic benches. Somehow we've ended up with several of the definitive little metal containers of small-bite-accompanying sauces, even though the original picture had zero of these. If photography is the documentation of specific light that actually exists for an isolated instant, independent of our subjective and temporal experience of it, then this is the opposite of that: an illustration of the schemata through which we perceive. But in the case of images, instead of a mean schema that integrates all of our diverse models, we get a median one drawn carelessly from the somewhere in the middle: these anonymous pretty people and their tiny aiolis, not depicted so much as photorealistically caricatured by schematography.
But then, one of the interesting things about photography is that our experience of our environment is never a simple geometry of light. I have a favorite vantage point on the Longfellow Bridge, between Cambridge and Boston, where I stop and take another frame of the same slow movie almost every day. The view is singular. The river and the sky bracket it cinemascopically, with the Esplanade stretched out across the midline, and Beacon Hill and downtown Boston rising up ahead of the bridge. I like this image, too, but it absolutely does not capture the feeling of standing on the bridge.
Run this photograph through our schematograph converter, though, and you get something that is wildly inaccurate but also sort of closer to the feeling:
The resulting dreamscape is, like the couple and their soft tacos, surprisingly stable across further iterations:
The actual Boston city-planners could explain some hard-learned lessons about running elevated freeways through the middle of your city, and maybe also give some basic-engineering tips about how suspension works. But as a rendition of what our cities would look like in the future if we had learned nothing from the past, this is both shiny and apt.
For now, though, while the city is still less shiny and more walkable, walk across that bridge, down the ducklings' path and past The Embrace to the office with me. The office windows overlook Readers' Park and the Boston Irish Famine Memorial, across the street from the Old South Meeting House. This is an intersection richly invested with American history, and also a Chipotle.
Schematography quantizes the particular odd geometry of this plaza into something more generically recognizable as City.
The urban equivalent of tiny aiolis appears to be rooftop HVAC units, which the schematograph has introduced into the view on its own. It seems a bit confused about the nature of automobiles, and has placed a couple of them on rooftops, one on a sidewalk and another wedged sideways next to the crosswalk that goes nowhere. The bench placements are a little dubious, and somebody appears to have left a garbage can on top of one. There's no way in or out of the little park, which the man at about 10:30 in the image has just realized.
These weirdnesses mostly get normalized out with a few more iterations, other than the bad parking, which is arguably the most Boston-like feature that survives the schematography:
Sometimes schematography is kind of what we're trying to do with photography anyway. This is a picture I took at a concert, and if you weren't there and naturally don't care about which specific show I was at, the schematographs of using darkness and lights as a sensory proxy for loud music in a crowded room are about as effective as the original:
The drummer appears to be running out of drums by the last image, and is the guitarist going to play the thigh-high chinmes with the end of his guitar? Also, does the singer's uncle, dancing in the background, realize that he is visible to the audience?
Sometimes, though, we take pictures to remember moments that specifically matter. Here's one of those:
Run reality through the schematograph and you can be reminded sharply about the difference between what actually happens and the assumptions that pile up in our data.
In the warroom of our unexamined dreams, the men are steely and pale, the cable-management is magical, and we have outgrown disposable coffee cups. Military men will clone themselves like minions if you aren't careful. You bring in just one to run the PowerPoint and by the time he has the screen-mirroring working correctly the room is full of them.
70 billion parameters are enough to suggest that some things are virtually certain. Men are serious, and serious things are meant for serious men. Serious men can be old or worried or both, but not neither. Serious men drink tap water. Women are occasional, but also the only ones who appear to be aware of what's going on around them. Something important is happening off-screen.
None of this is really news. Important things are always happening off-screen. Sometimes an unguarded reflection sneaks through, and we get a glimpse of the implied subject. The important present things for the future of Artificial Intelligence are probably the ways in which these schematographs and chatbots and accelerating generations are not intelligence, but the important present things for the future of people are probably the ways in which this automation is only superficially artificial. AI is not an alien oracle come to enlighten or enslave us. It's us, in increasingly elaborate costumes, aspiring to be unrecognizable in the most astonishing detail, but always absolutely unmistakable in our own limited and reconverging imaginations.
One of the many amazing things ChatGPT can do, though, is describe images in words. Another one of these amazing things it can do is produce images from descriptions.
It's entertaining to chain these two things together. Tell it to describe a picture in precise detail, then give it back that description and tell it to generate that image. Here's a picture of my wife and I eating a casually celebratory dinner:
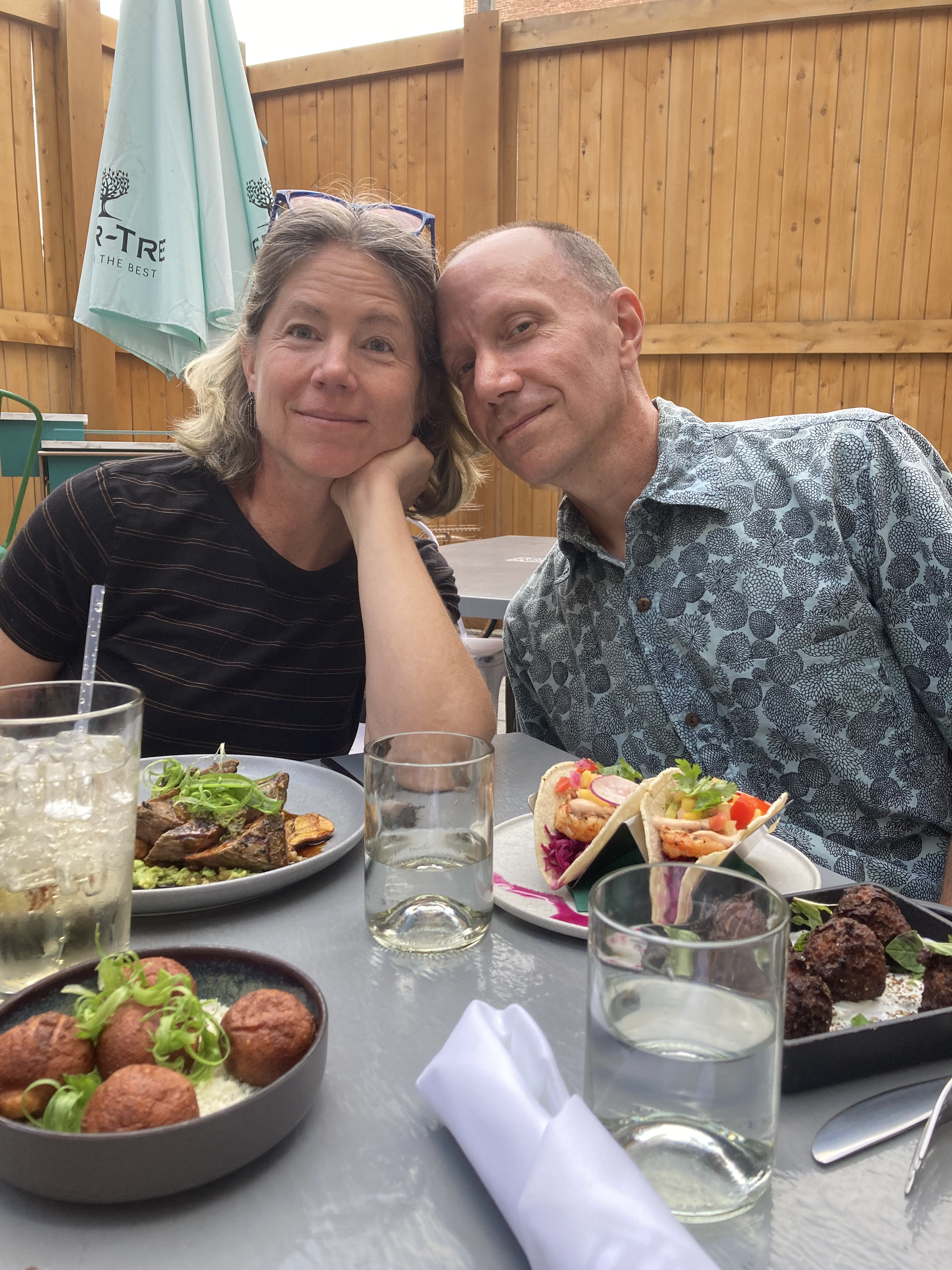
And here's what image-to-description-to-image turns us into:
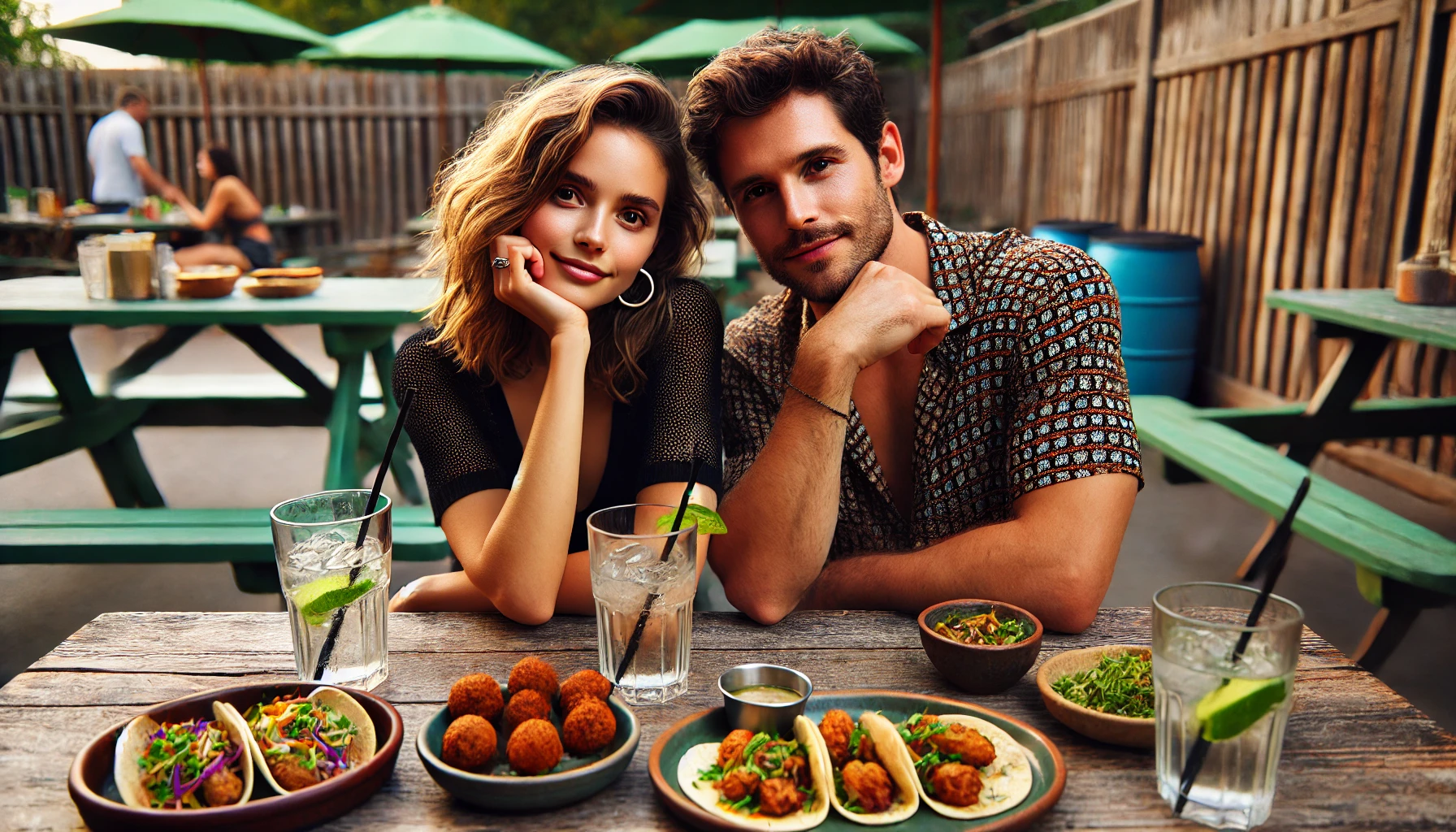
It made us younger and hotter, obviously, but the other details are also intriguing. All the drinks have limes, now, and straws pointing right. The one closed umbrella has been rendered canonically open and multiple. Every major detail in the original picture has been transliterated into its paradigmatic, normative form. This process is even more obvious if you keep going a few more iterations, feeding each generated image back into the loop:
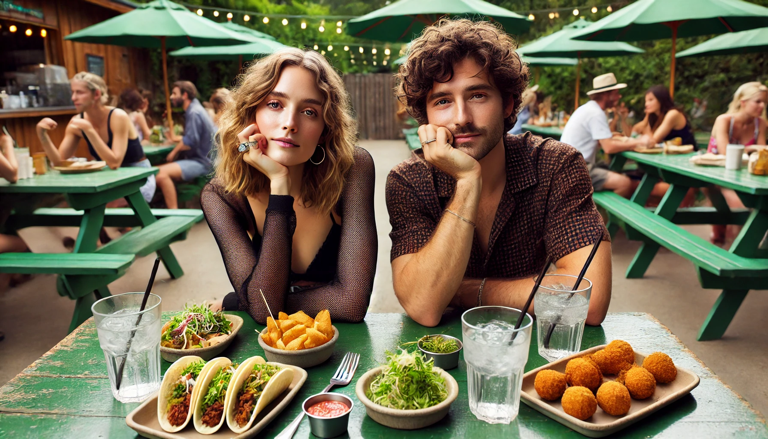
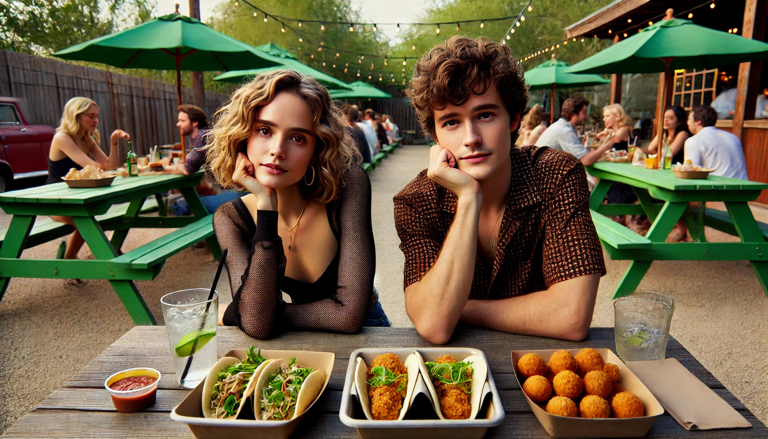
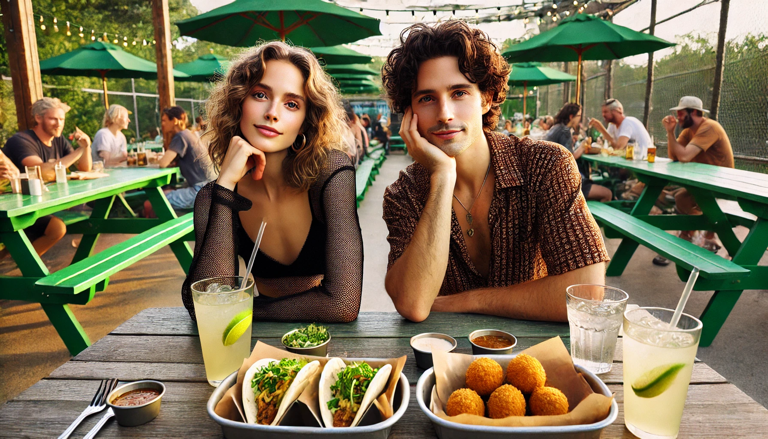
We have converged on the LLM equivalent of Platonic Forms: the most soft-taco-like soft tacos, the most people-being-photographed haircuts, the most endlessly elemental green picnic benches. Somehow we've ended up with several of the definitive little metal containers of small-bite-accompanying sauces, even though the original picture had zero of these. If photography is the documentation of specific light that actually exists for an isolated instant, independent of our subjective and temporal experience of it, then this is the opposite of that: an illustration of the schemata through which we perceive. But in the case of images, instead of a mean schema that integrates all of our diverse models, we get a median one drawn carelessly from the somewhere in the middle: these anonymous pretty people and their tiny aiolis, not depicted so much as photorealistically caricatured by schematography.
But then, one of the interesting things about photography is that our experience of our environment is never a simple geometry of light. I have a favorite vantage point on the Longfellow Bridge, between Cambridge and Boston, where I stop and take another frame of the same slow movie almost every day. The view is singular. The river and the sky bracket it cinemascopically, with the Esplanade stretched out across the midline, and Beacon Hill and downtown Boston rising up ahead of the bridge. I like this image, too, but it absolutely does not capture the feeling of standing on the bridge.
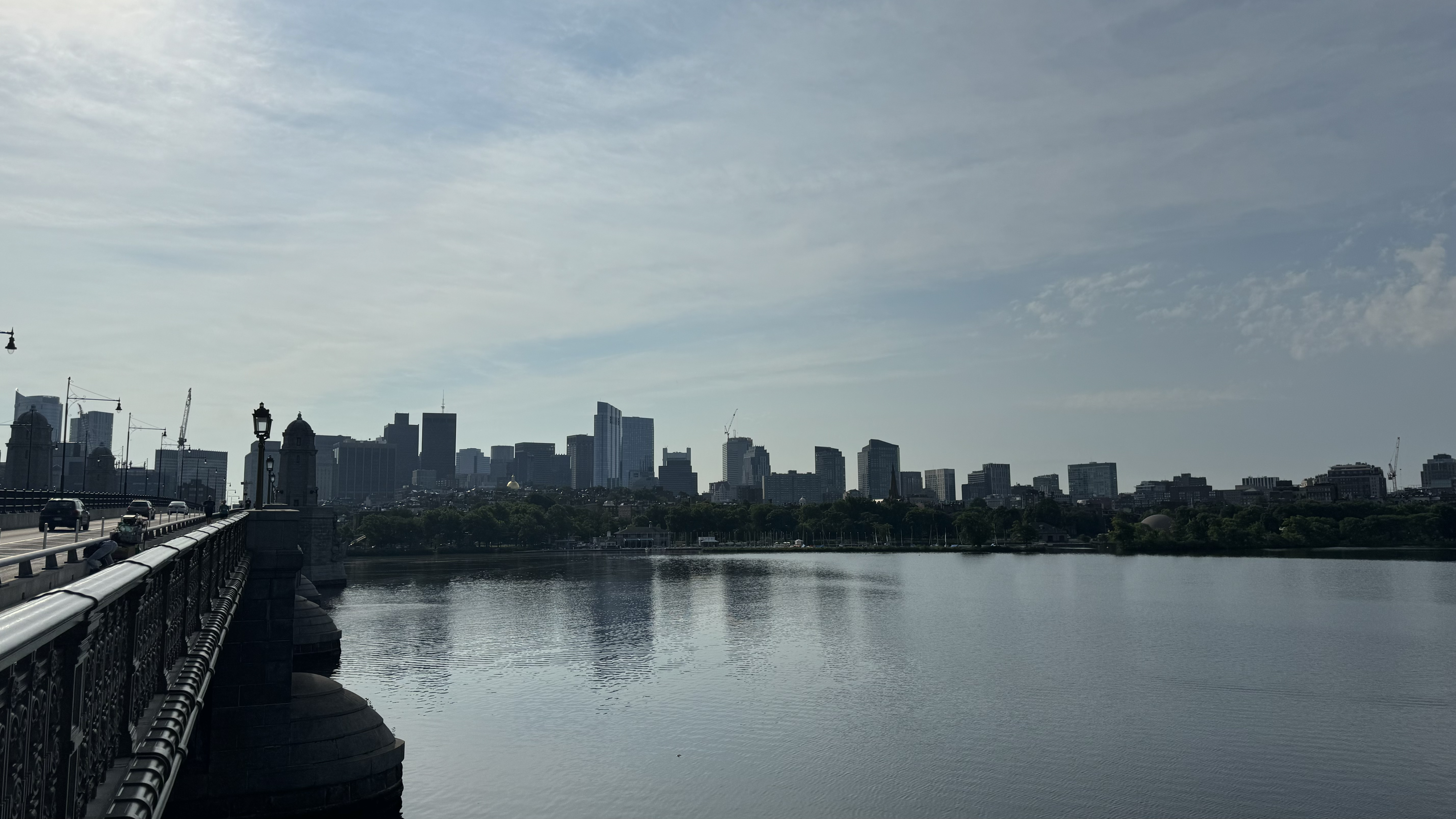
Run this photograph through our schematograph converter, though, and you get something that is wildly inaccurate but also sort of closer to the feeling:
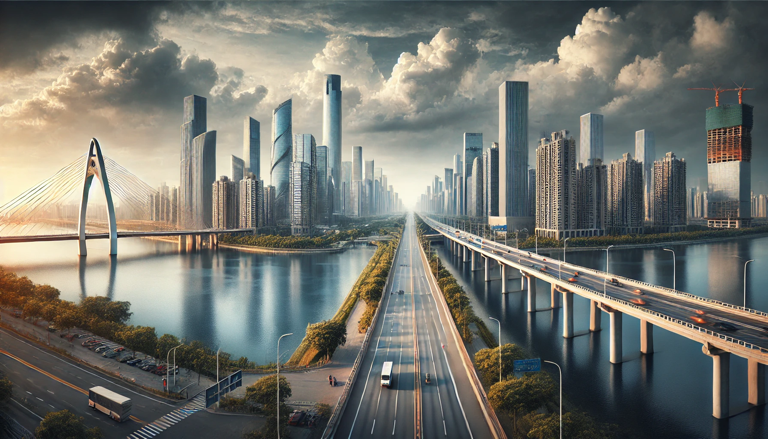
The resulting dreamscape is, like the couple and their soft tacos, surprisingly stable across further iterations:
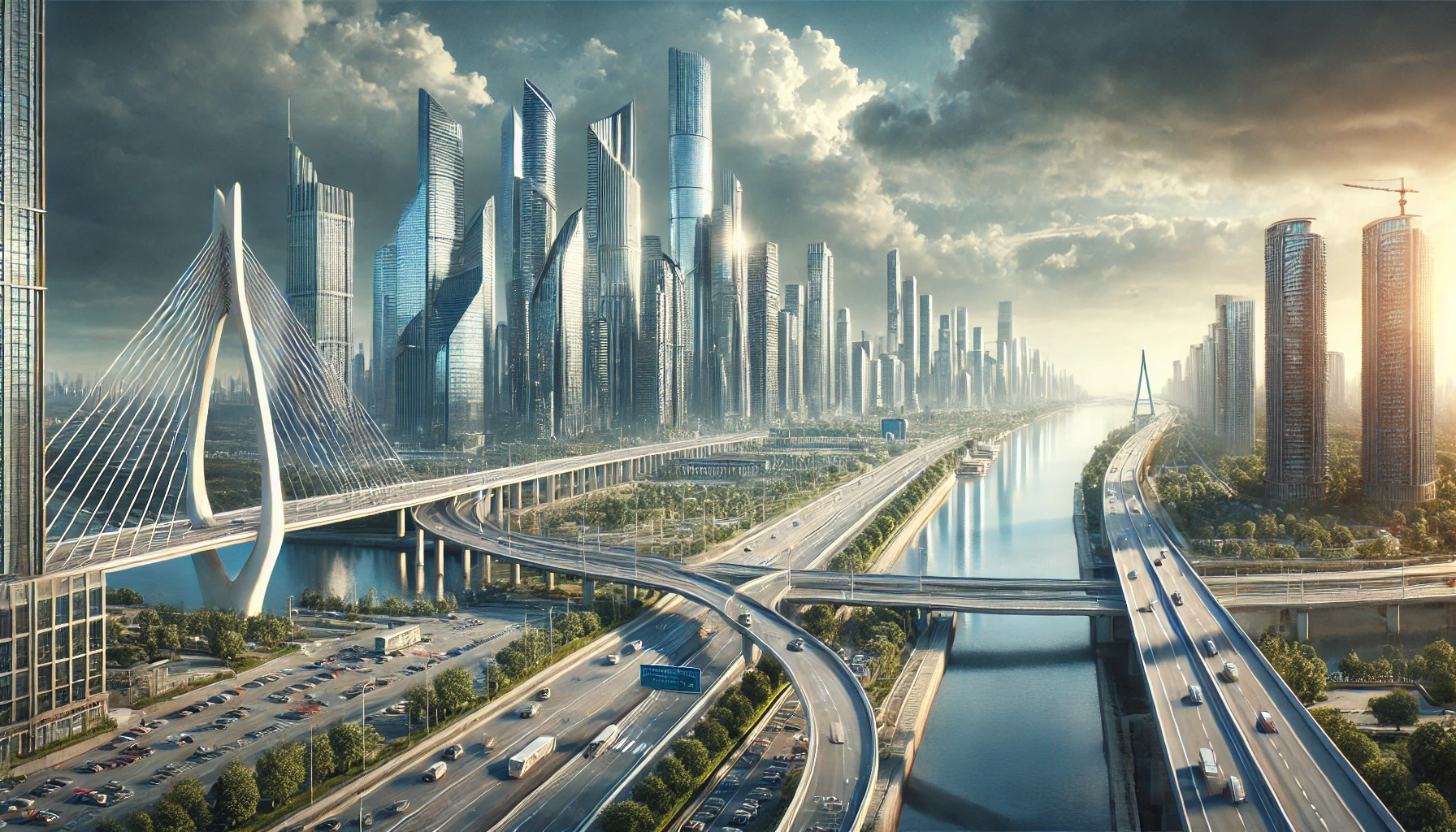
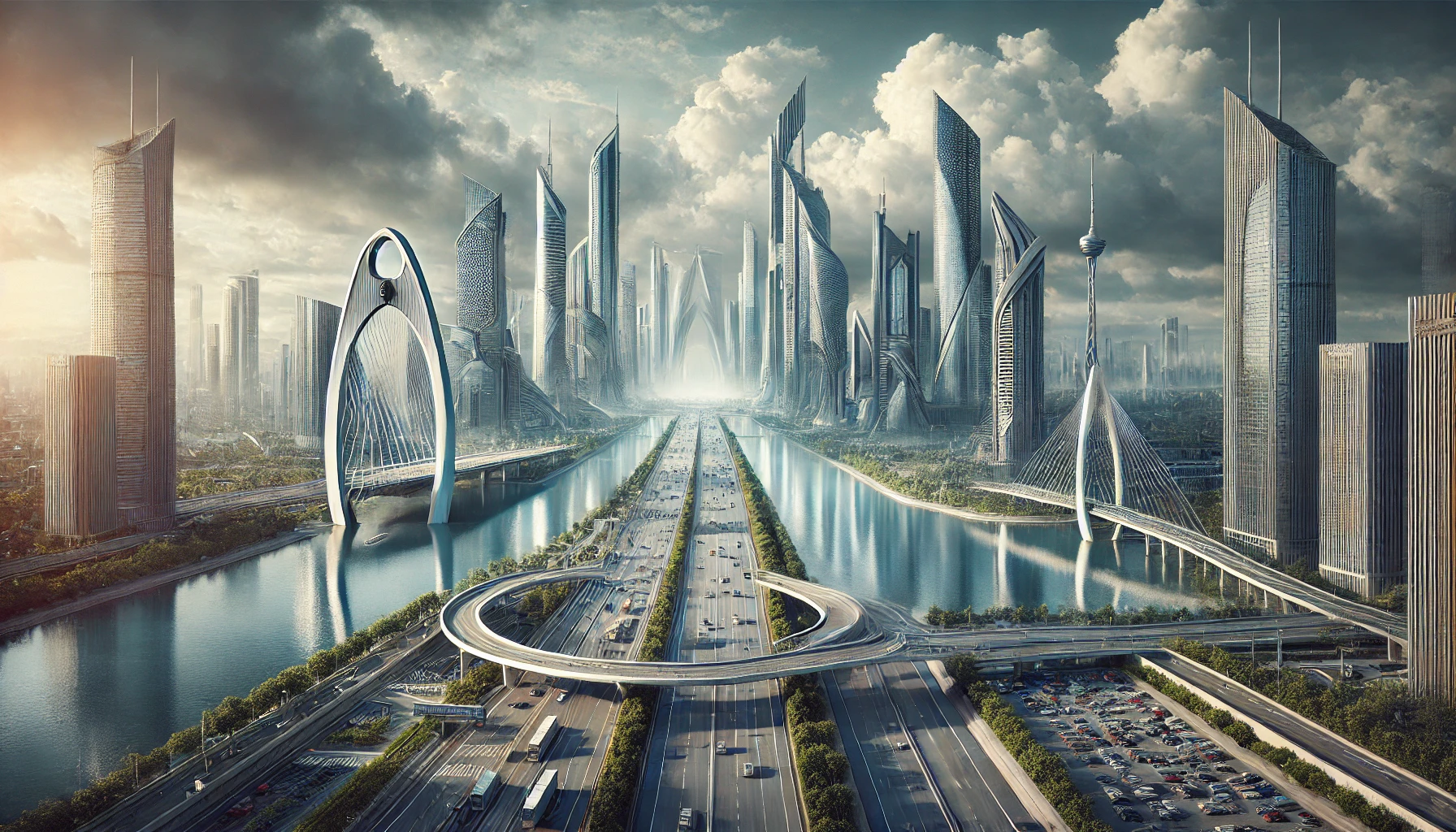
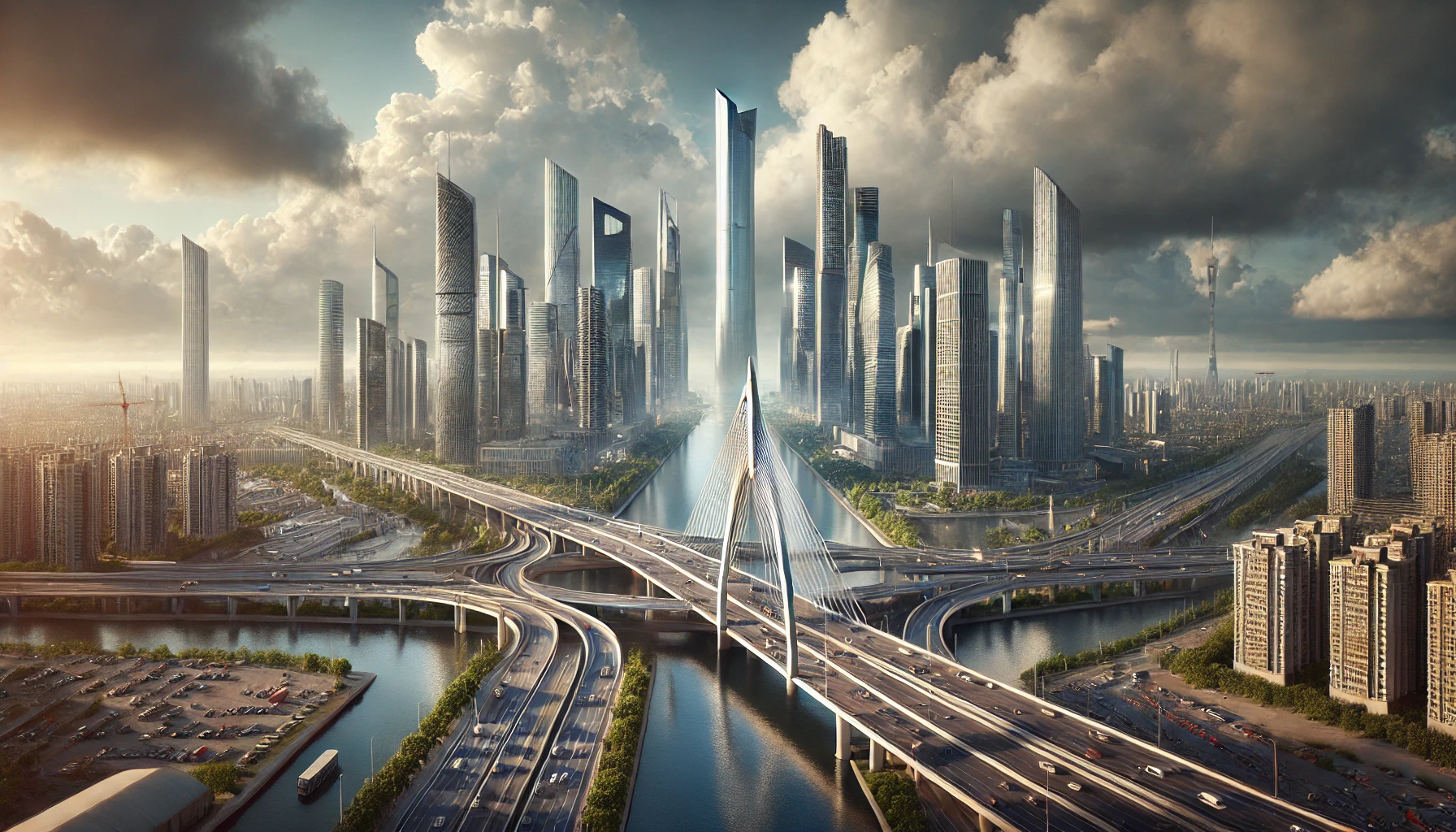
The actual Boston city-planners could explain some hard-learned lessons about running elevated freeways through the middle of your city, and maybe also give some basic-engineering tips about how suspension works. But as a rendition of what our cities would look like in the future if we had learned nothing from the past, this is both shiny and apt.
For now, though, while the city is still less shiny and more walkable, walk across that bridge, down the ducklings' path and past The Embrace to the office with me. The office windows overlook Readers' Park and the Boston Irish Famine Memorial, across the street from the Old South Meeting House. This is an intersection richly invested with American history, and also a Chipotle.
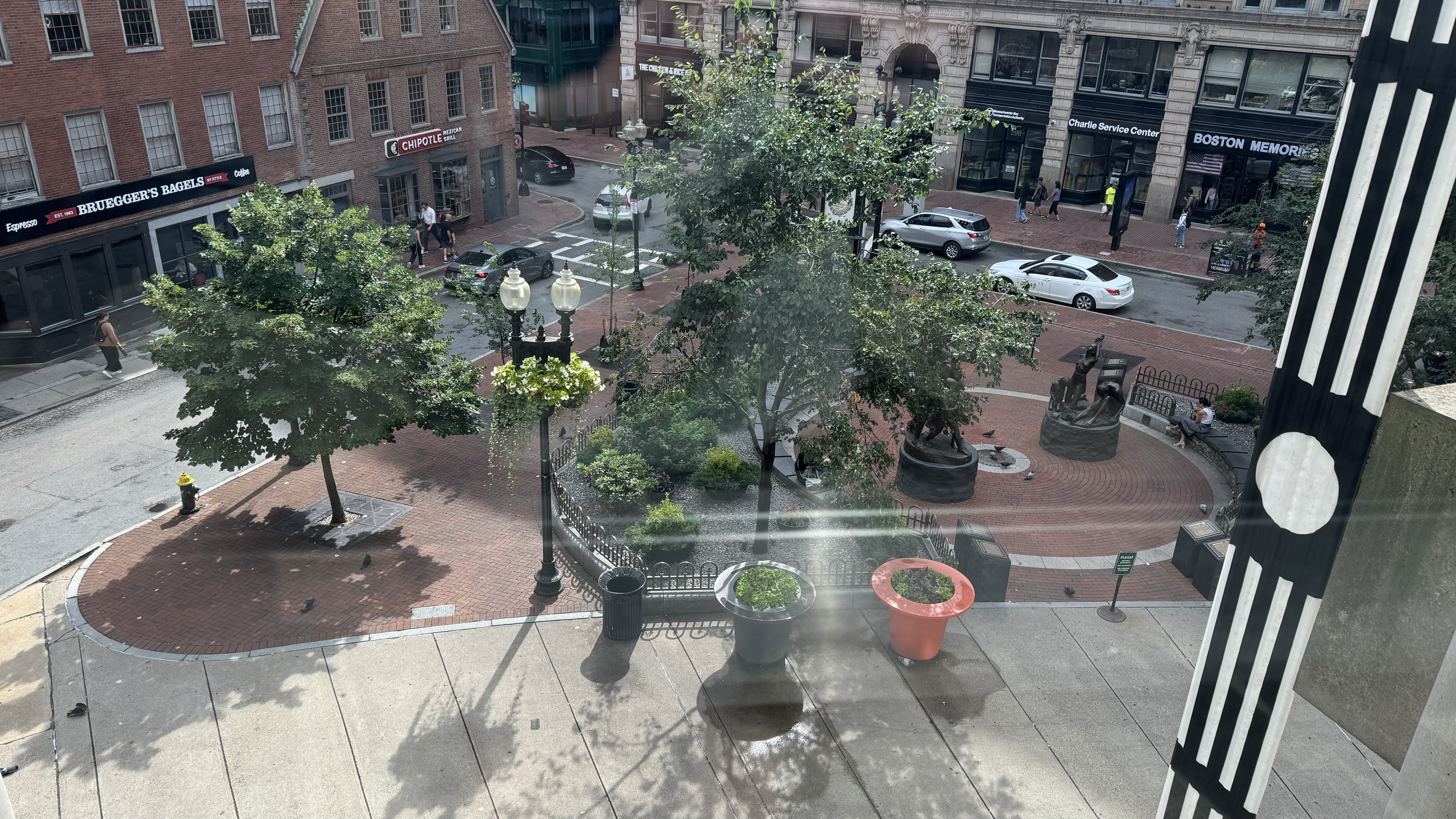
Schematography quantizes the particular odd geometry of this plaza into something more generically recognizable as City.
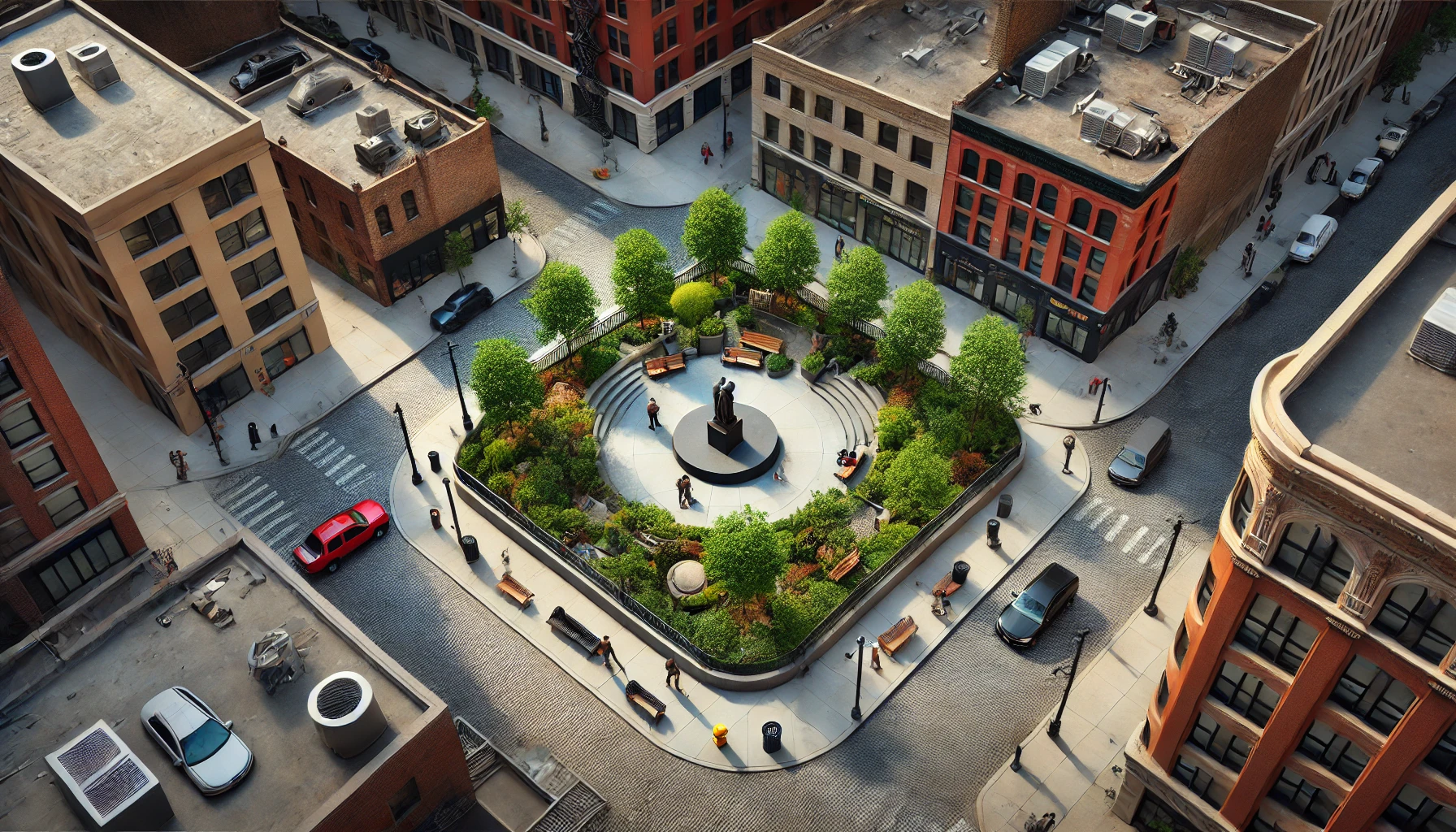
The urban equivalent of tiny aiolis appears to be rooftop HVAC units, which the schematograph has introduced into the view on its own. It seems a bit confused about the nature of automobiles, and has placed a couple of them on rooftops, one on a sidewalk and another wedged sideways next to the crosswalk that goes nowhere. The bench placements are a little dubious, and somebody appears to have left a garbage can on top of one. There's no way in or out of the little park, which the man at about 10:30 in the image has just realized.
These weirdnesses mostly get normalized out with a few more iterations, other than the bad parking, which is arguably the most Boston-like feature that survives the schematography:
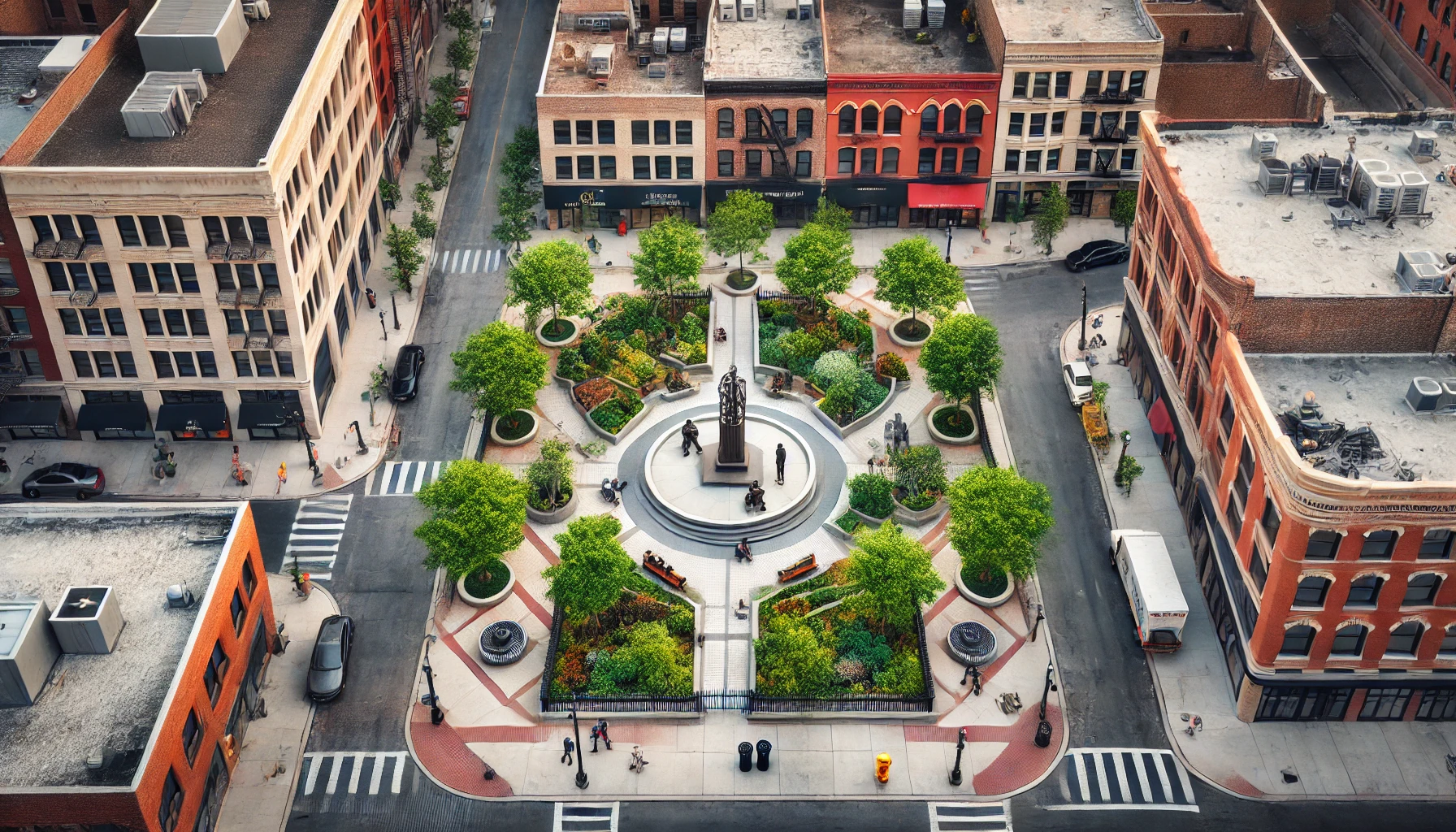
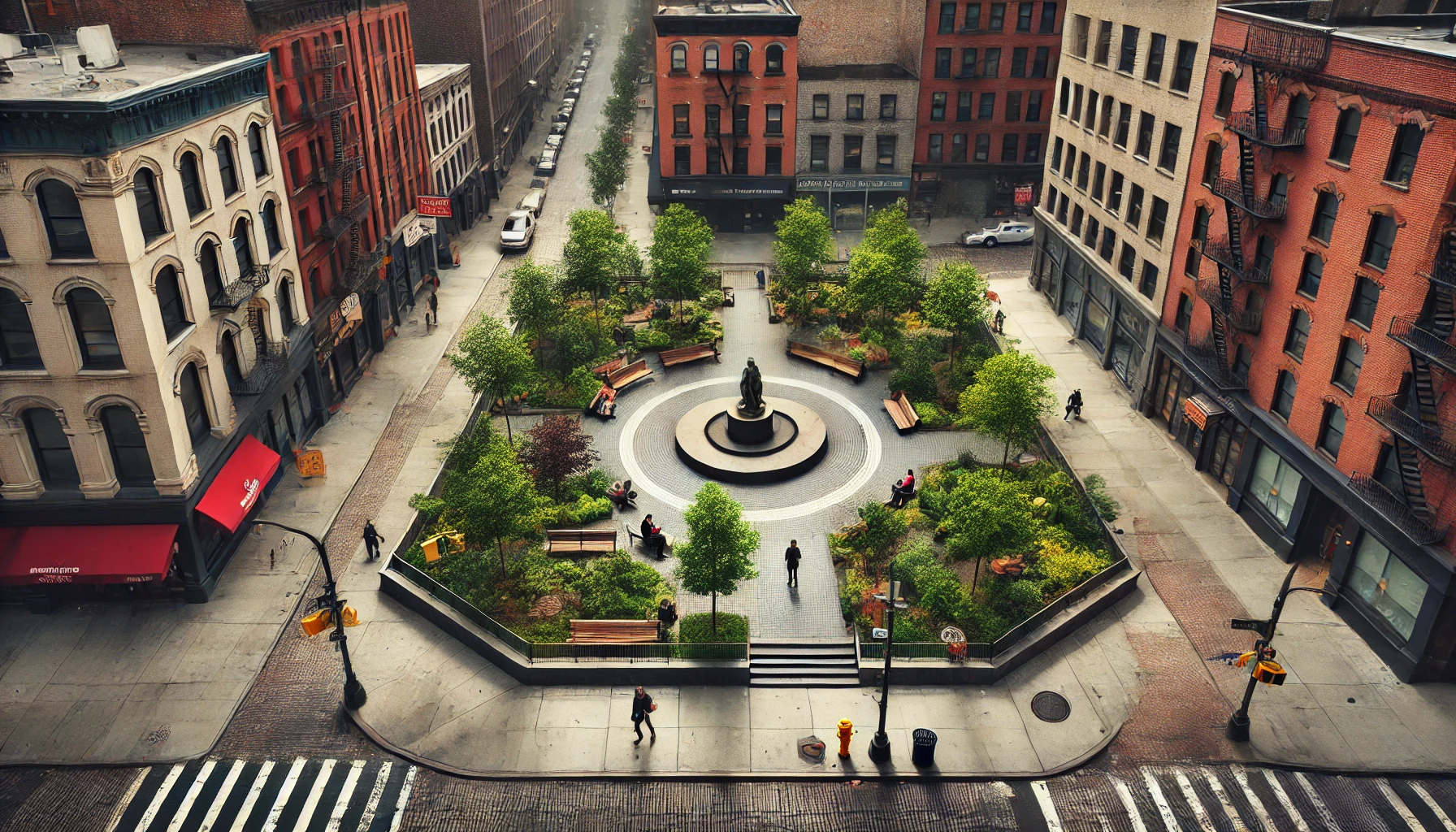
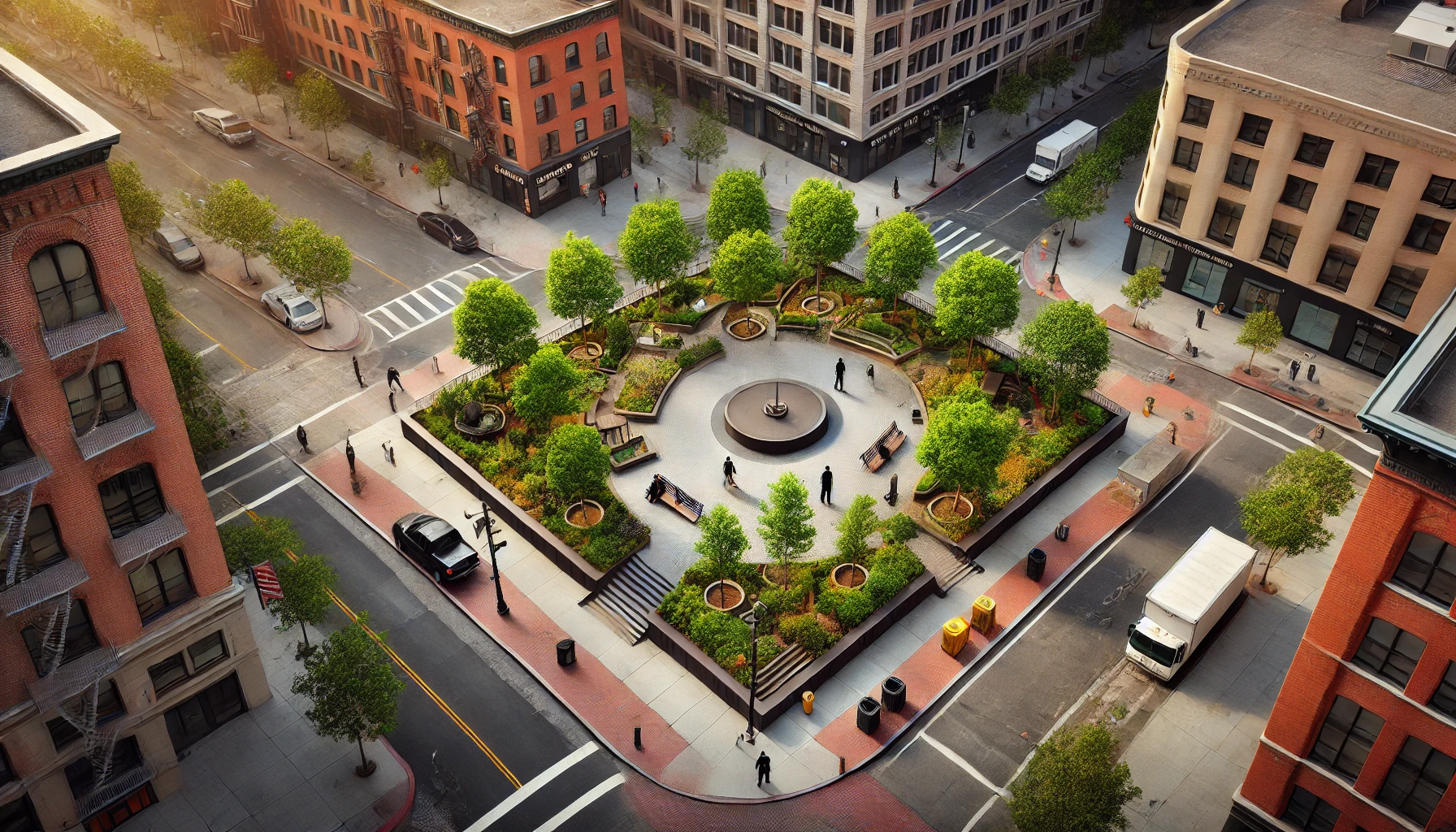
Sometimes schematography is kind of what we're trying to do with photography anyway. This is a picture I took at a concert, and if you weren't there and naturally don't care about which specific show I was at, the schematographs of using darkness and lights as a sensory proxy for loud music in a crowded room are about as effective as the original:
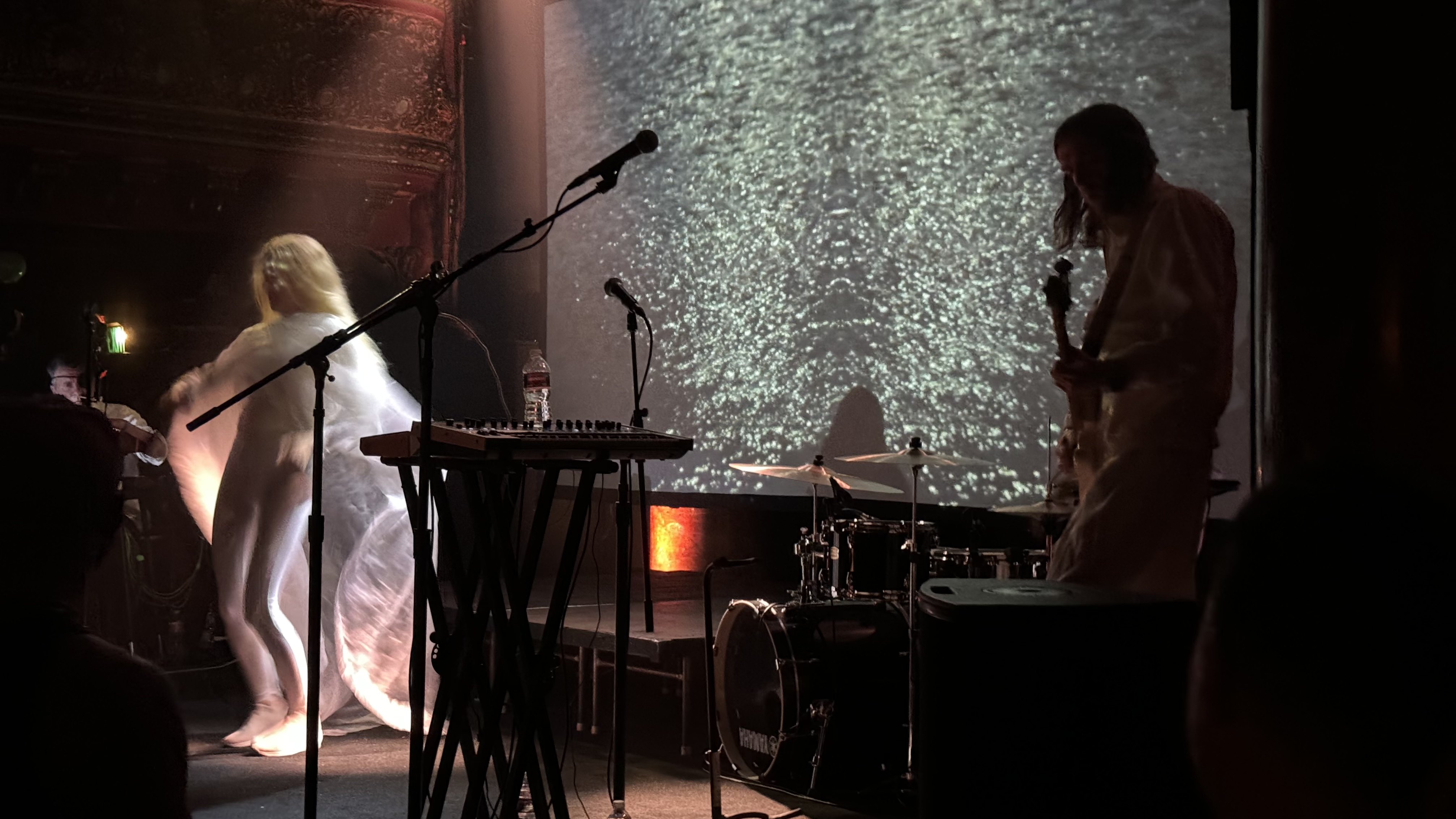
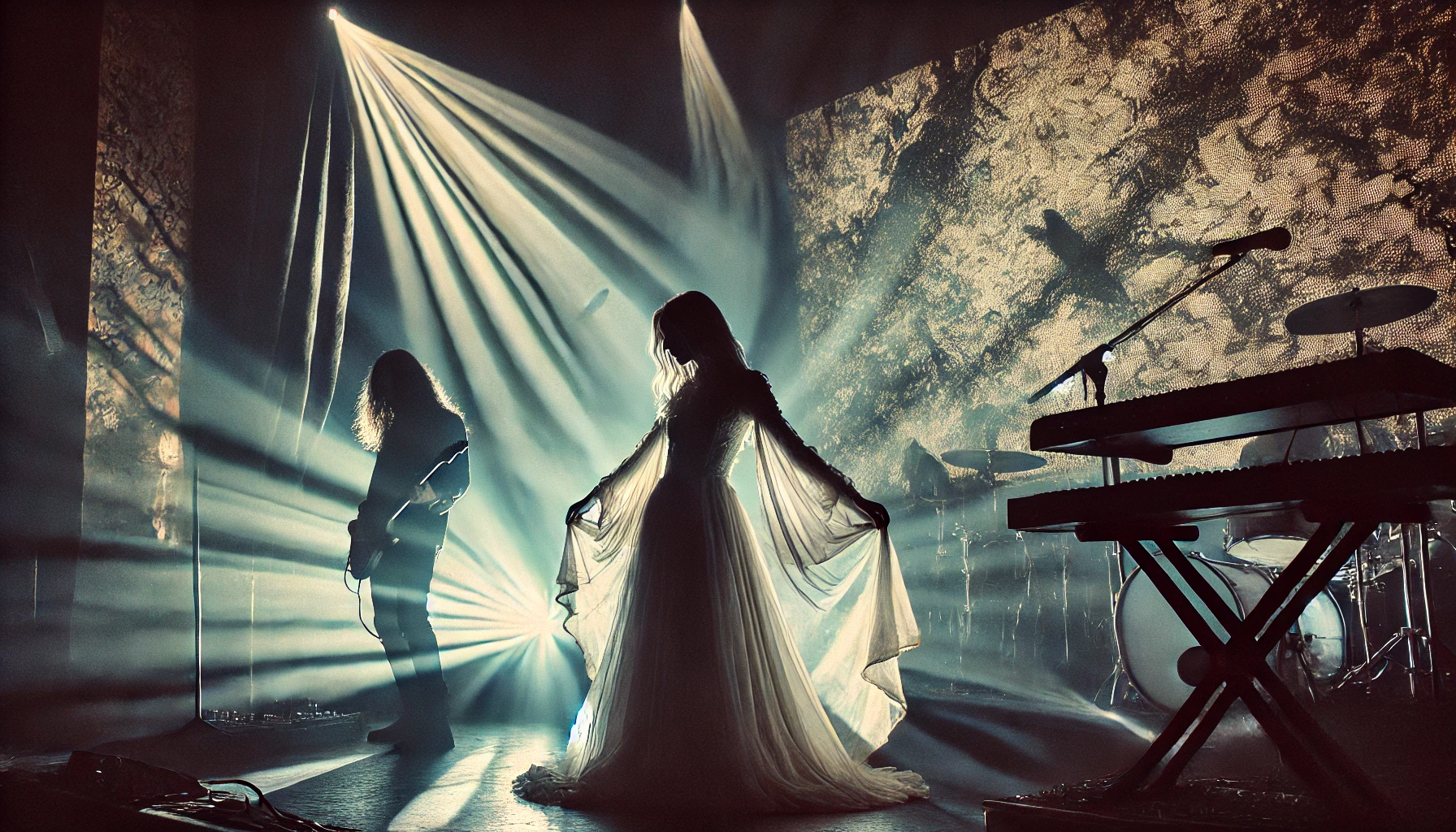
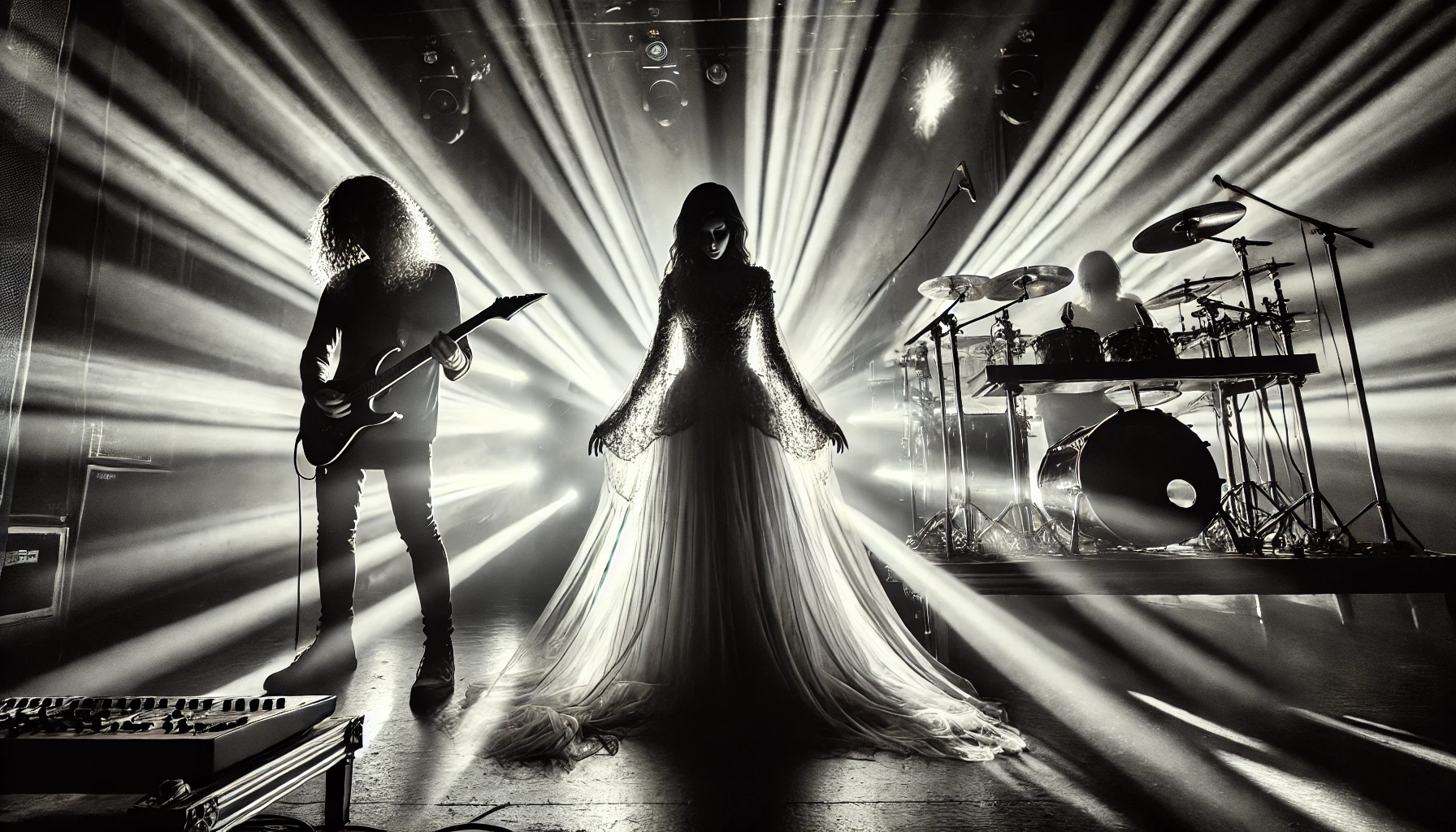
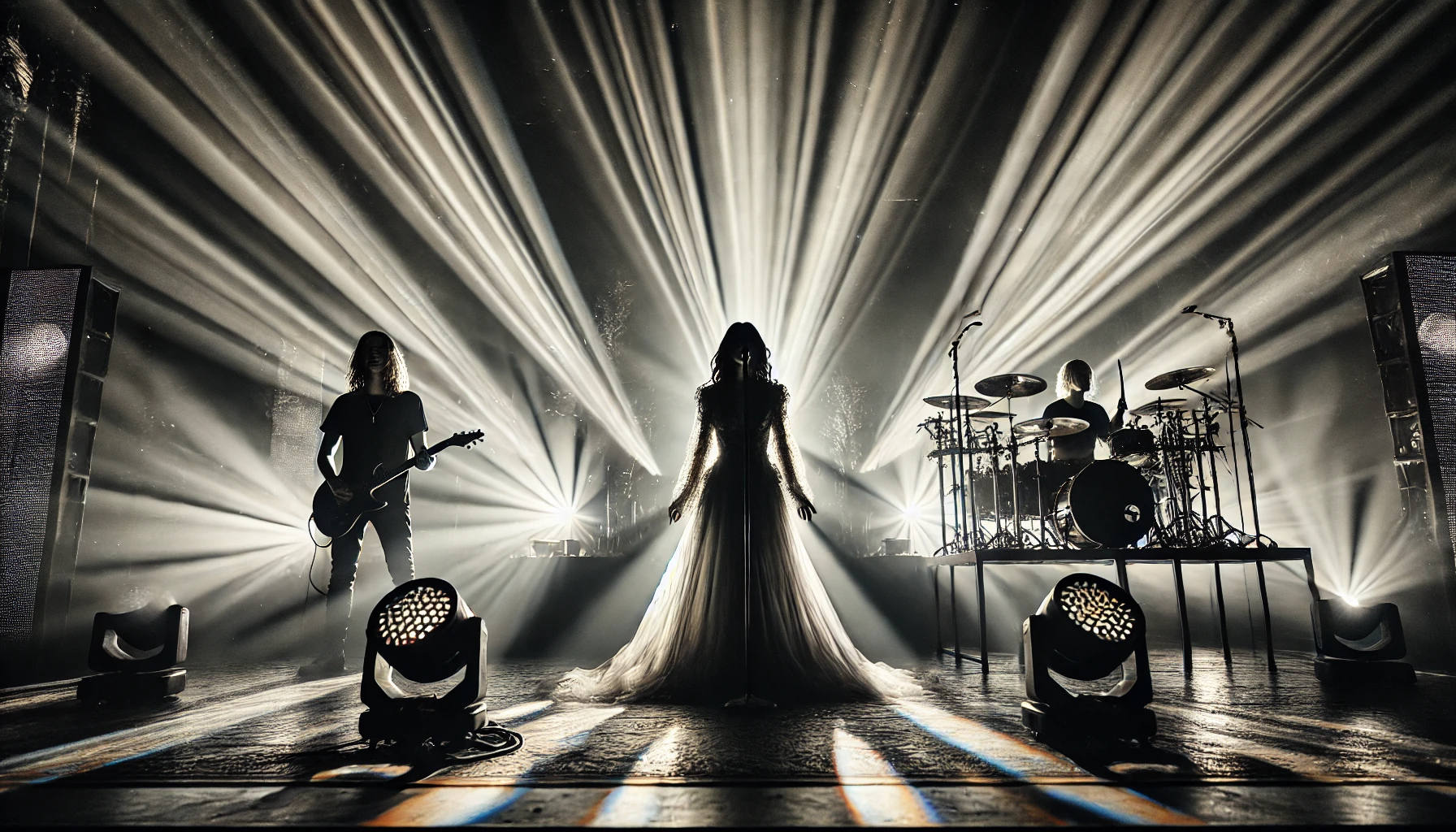
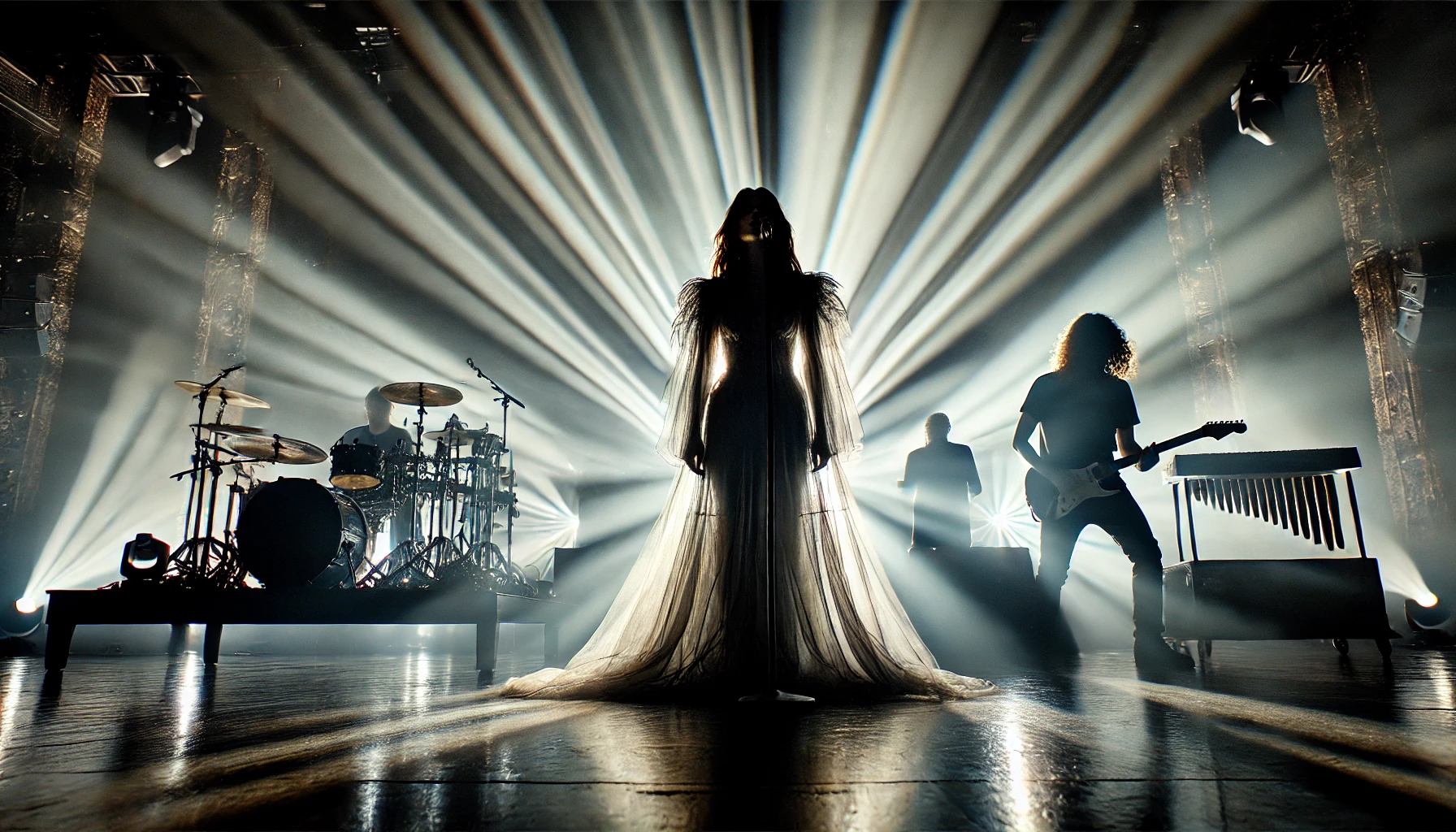
The drummer appears to be running out of drums by the last image, and is the guitarist going to play the thigh-high chinmes with the end of his guitar? Also, does the singer's uncle, dancing in the background, realize that he is visible to the audience?
Sometimes, though, we take pictures to remember moments that specifically matter. Here's one of those:
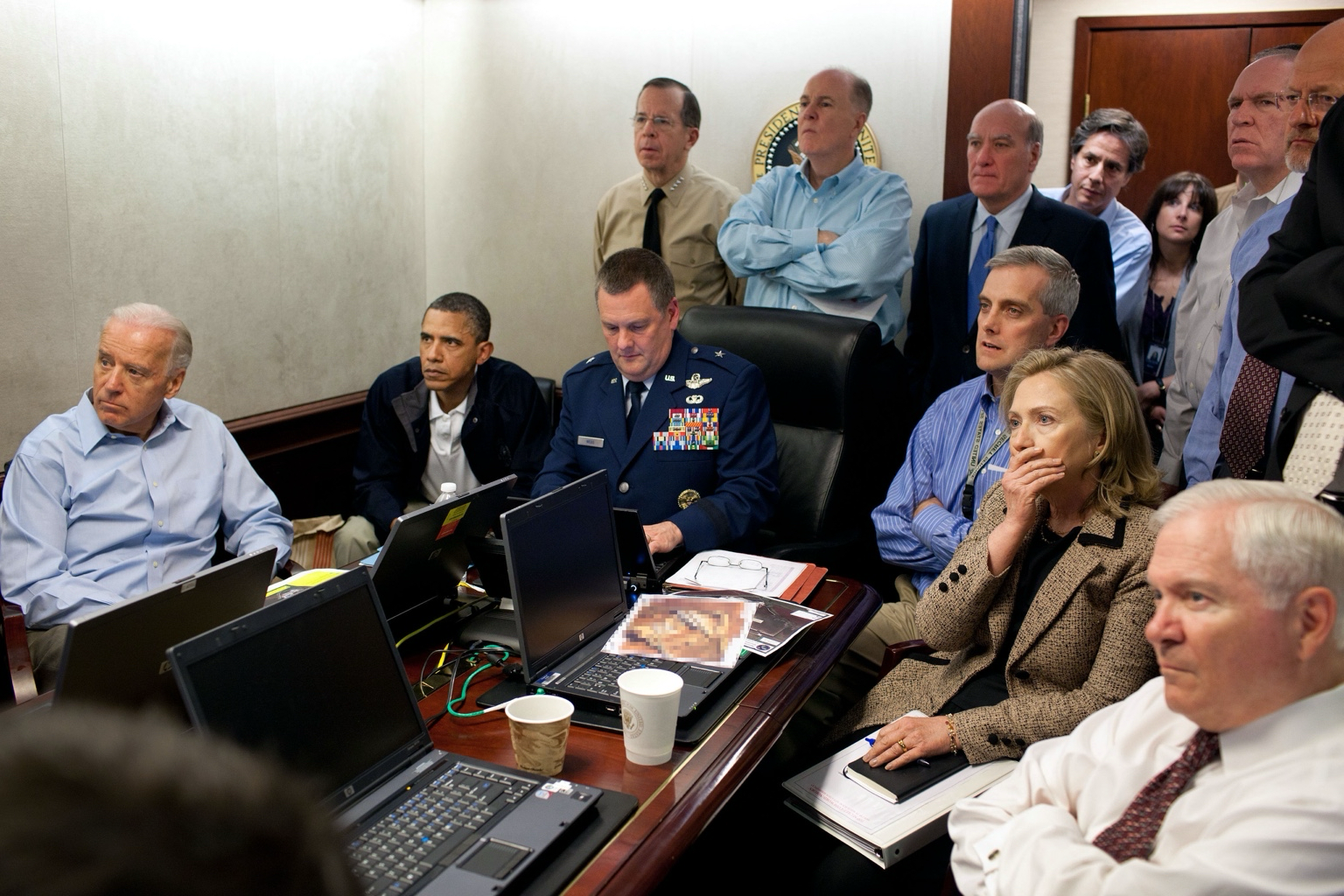
Run reality through the schematograph and you can be reminded sharply about the difference between what actually happens and the assumptions that pile up in our data.
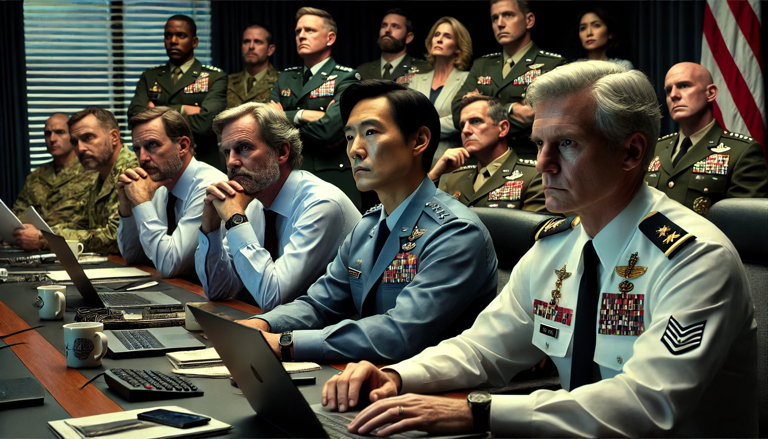
In the warroom of our unexamined dreams, the men are steely and pale, the cable-management is magical, and we have outgrown disposable coffee cups. Military men will clone themselves like minions if you aren't careful. You bring in just one to run the PowerPoint and by the time he has the screen-mirroring working correctly the room is full of them.
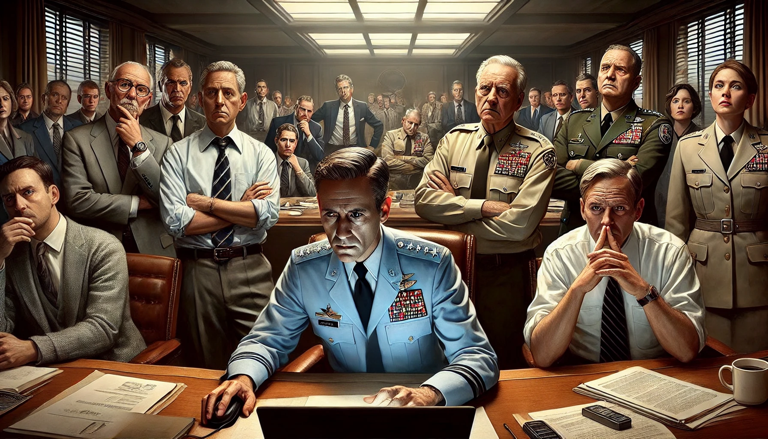
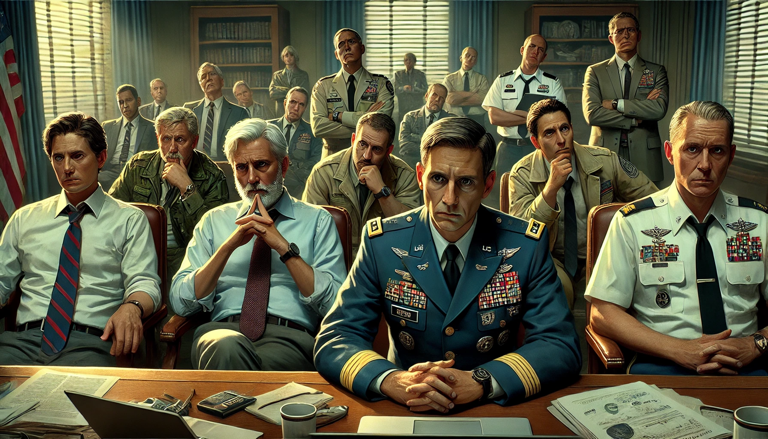
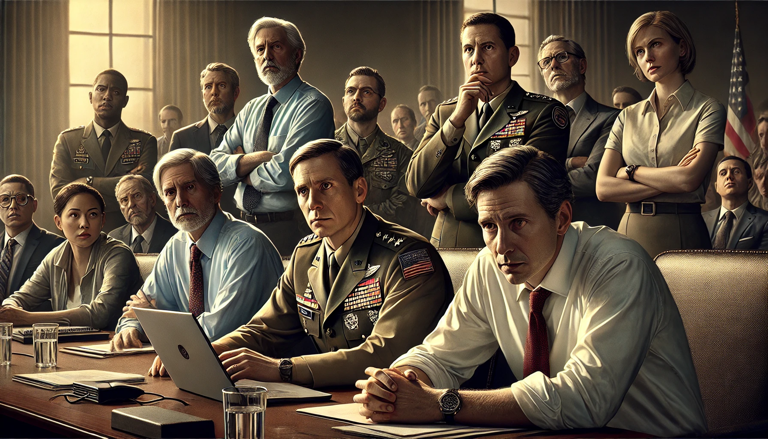
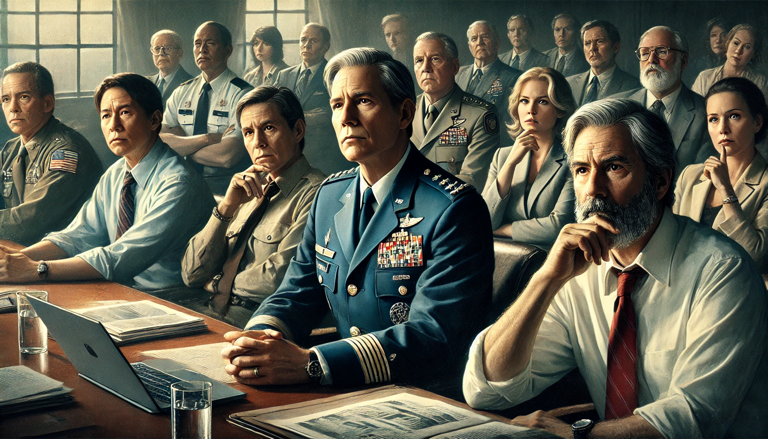
70 billion parameters are enough to suggest that some things are virtually certain. Men are serious, and serious things are meant for serious men. Serious men can be old or worried or both, but not neither. Serious men drink tap water. Women are occasional, but also the only ones who appear to be aware of what's going on around them. Something important is happening off-screen.
None of this is really news. Important things are always happening off-screen. Sometimes an unguarded reflection sneaks through, and we get a glimpse of the implied subject. The important present things for the future of Artificial Intelligence are probably the ways in which these schematographs and chatbots and accelerating generations are not intelligence, but the important present things for the future of people are probably the ways in which this automation is only superficially artificial. AI is not an alien oracle come to enlighten or enslave us. It's us, in increasingly elaborate costumes, aspiring to be unrecognizable in the most astonishing detail, but always absolutely unmistakable in our own limited and reconverging imaginations.