15 July 2014 to 29 April 2014
¶ empath playlists · 15 July 2014 listen/tech
The empath charts for best albums by year, style and country now have linked Spotify playlists to go with each year/style/country.
Which means, among other things, that there's now this playlist of songs from the current best metal albums of 2014, as rated by Encyclopaedia Metallum reviewers via my peculiar math:
Or, at the less-dynamic end of the spectrum, songs from the best metal albums from the Faroe Islands, a list I monitor assiduously in case it ever gets a band other than Tyr.
Which means, among other things, that there's now this playlist of songs from the current best metal albums of 2014, as rated by Encyclopaedia Metallum reviewers via my peculiar math:
Or, at the less-dynamic end of the spectrum, songs from the best metal albums from the Faroe Islands, a list I monitor assiduously in case it ever gets a band other than Tyr.
I really like the new album by this Dutch band BLØF, and the Dutch are in the World Cup, so naturally I made a playlist with one song from each of the 32 countries in the finals. The songs were chosen capriciously by me, and span a variety of styles.
I would love to say that I just happened to know good recent songs I liked from all 32 of these countries, but that was not true. There were 15 of those, and 4 more where I knew the artist already but not the song. The other 13 I have dug up specifically for this occasion.
If you want a puzzle, in addition to some songs, you can try to figure out which song goes with which country, and then see if you can discern why they're in this particular order. I'm not saying you should do this. Probably better to just listen to the songs.
[Update: to keep this fun going, I am adding another song from each country that advances to another round, so with the round of 16 complete this is up to 56 songs, all of which are interesting and most of which I bet you have not heard.]
I would love to say that I just happened to know good recent songs I liked from all 32 of these countries, but that was not true. There were 15 of those, and 4 more where I knew the artist already but not the song. The other 13 I have dug up specifically for this occasion.
If you want a puzzle, in addition to some songs, you can try to figure out which song goes with which country, and then see if you can discern why they're in this particular order. I'm not saying you should do this. Probably better to just listen to the songs.
[Update: to keep this fun going, I am adding another song from each country that advances to another round, so with the round of 16 complete this is up to 56 songs, all of which are interesting and most of which I bet you have not heard.]
¶ Every Noise at Once on Spotify · 20 June 2014 listen/tech
To celebrate the release of the slick new Spotify Web API, I've converted Every Noise at Once to use the :30 preview clips provided by the new API.
Examples ought to now start playing quite a bit more promptly when you click them, there are examples available for more artists in the individual genre maps, and the whole thing ought to work in some parts of the world where it previously didn't.
The wistful flipside of this transition is that the map no longer uses or links to Rdio for anything. I remain very fond of Rdio, and they were great Echo Nest partners and enthusiastic supporters of this whole genre project from the beginning. But given the Echo Nest's acquisition by Spotify, Rdio's decision to stop using our services is, at least in business terms, unsurprising. And thus, conversely, I won't be producing or updating genre playlists on Rdio any more. New things (and there will be lots of new things) will now all happen on Spotify. Come on over.
Examples ought to now start playing quite a bit more promptly when you click them, there are examples available for more artists in the individual genre maps, and the whole thing ought to work in some parts of the world where it previously didn't.
The wistful flipside of this transition is that the map no longer uses or links to Rdio for anything. I remain very fond of Rdio, and they were great Echo Nest partners and enthusiastic supporters of this whole genre project from the beginning. But given the Echo Nest's acquisition by Spotify, Rdio's decision to stop using our services is, at least in business terms, unsurprising. And thus, conversely, I won't be producing or updating genre playlists on Rdio any more. New things (and there will be lots of new things) will now all happen on Spotify. Come on over.
I really can't sleep sitting up. But I can write songs on planes. It's a trade-off.
So here. This is my new song, composed and played on the flight from Boston to Keflavik, and sung in my Stockholm hotel room a few hours later as I fought to stay awake until a reasonable bedtime.
Methods Out of Favor (4:10)
All my songs kind of sound a little bit the same, which I'm OK with, but this one is at least in a slightly different tempo.
So here. This is my new song, composed and played on the flight from Boston to Keflavik, and sung in my Stockholm hotel room a few hours later as I fought to stay awake until a reasonable bedtime.
Methods Out of Favor (4:10)
All my songs kind of sound a little bit the same, which I'm OK with, but this one is at least in a slightly different tempo.
¶ A little more press · 21 May 2014 listen/tech
A couple new bits of press about Every Noise at Once:
PolicyMic - Discover Incredible New Music You've Never Heard Using This Interactive Map
Daily Dot - Dive into Every Noise at Once, a musical map of genres you didn't know existed
The first one is particularly good in the sense of being written thoughtfully by somebody other than me. The second one is particularly good in the sense of consisting largely of quotes from my answers to their questions.
PolicyMic - Discover Incredible New Music You've Never Heard Using This Interactive Map
Daily Dot - Dive into Every Noise at Once, a musical map of genres you didn't know existed
The first one is particularly good in the sense of being written thoughtfully by somebody other than me. The second one is particularly good in the sense of consisting largely of quotes from my answers to their questions.
¶ Every Noise at Once, more oncely · 20 May 2014 listen/tech
The Every Noise at Once genre-map reduces 13 music-analytical dimensions to 2 visual dimensions.
But if that's still a little too much for you, I've now added a version that reduces the whole genre space to one dimension: a list. But a list that you can sort and filter several different ways!
If one dimension is still too profuse and rococo for you, the reduction of the analytical space into zero dimensions is kind of this:
But if that's still a little too much for you, I've now added a version that reduces the whole genre space to one dimension: a list. But a list that you can sort and filter several different ways!
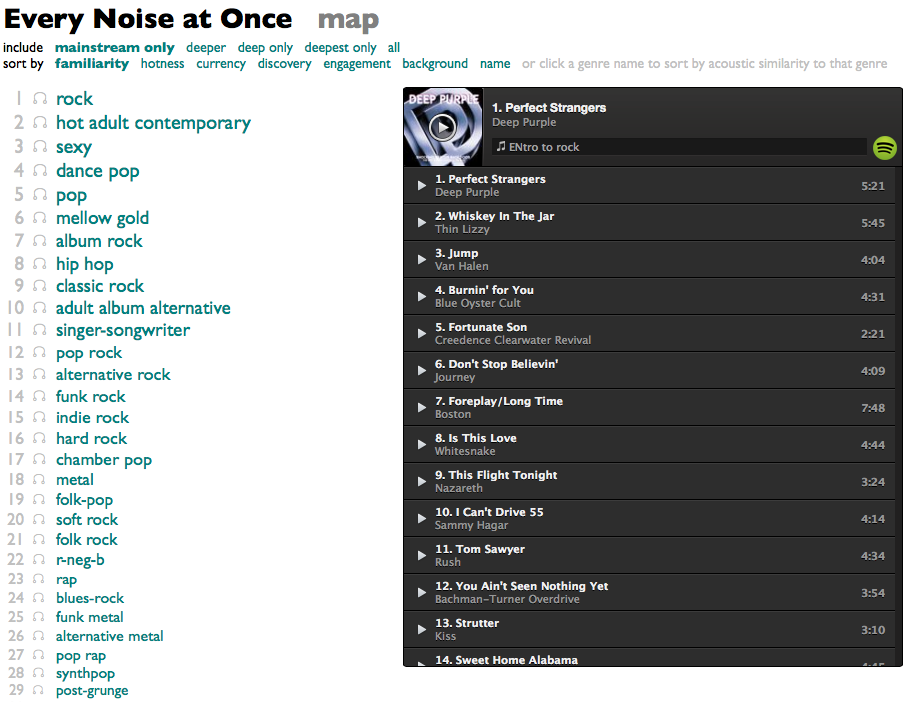
If one dimension is still too profuse and rococo for you, the reduction of the analytical space into zero dimensions is kind of this:
¶ Drunkard's Rock · 19 May 2014 listen/tech
Drunkard's Walk is a mathematical idea involving a random iterative traversal of a multidimensional space.
Drunkard's Rock is an experiment I did to pursue a random iterative traversal of the multidimensional musical artist-similarity space.
In the mathematical version, the drunkard is allowed to retrace their steps, and in fact the point of the problem is to determine the chance of the drunkard randomly arriving home again.
In my version, retracing steps is explicitly disallowed, and thus the drunkard is doomed to wander until the universe expires. Probably it says something about my personality that this seems like the preferable curse to me.
Anyway, I started the calculation with Black Sabbath, both because my own musical evolution sort of started in earnest with Black Sabbath, and because Paul Lamere used Black Sabbath as the reference point in his inversely minded Six Degrees of Black Sabbath, which attempts to find the shortest path between two bands.
My version, to reiterate, just keeps wandering. I guess it is searching for the longest path between Black Sabbath and whatever it finds last. Except I stopped it at 100k steps, because the resulting web page is enormous enough. It will annoy you least if you just leave it alone for a couple minutes while it loads, and then you should be able to scroll around.
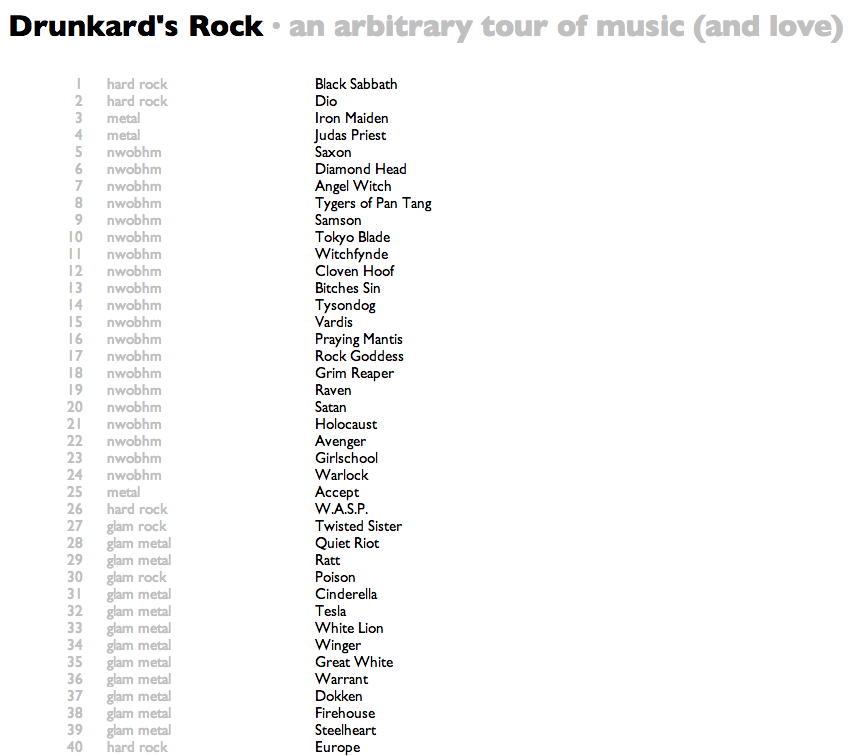
Drunkard's Rock is an experiment I did to pursue a random iterative traversal of the multidimensional musical artist-similarity space.
In the mathematical version, the drunkard is allowed to retrace their steps, and in fact the point of the problem is to determine the chance of the drunkard randomly arriving home again.
In my version, retracing steps is explicitly disallowed, and thus the drunkard is doomed to wander until the universe expires. Probably it says something about my personality that this seems like the preferable curse to me.
Anyway, I started the calculation with Black Sabbath, both because my own musical evolution sort of started in earnest with Black Sabbath, and because Paul Lamere used Black Sabbath as the reference point in his inversely minded Six Degrees of Black Sabbath, which attempts to find the shortest path between two bands.
My version, to reiterate, just keeps wandering. I guess it is searching for the longest path between Black Sabbath and whatever it finds last. Except I stopped it at 100k steps, because the resulting web page is enormous enough. It will annoy you least if you just leave it alone for a couple minutes while it loads, and then you should be able to scroll around.
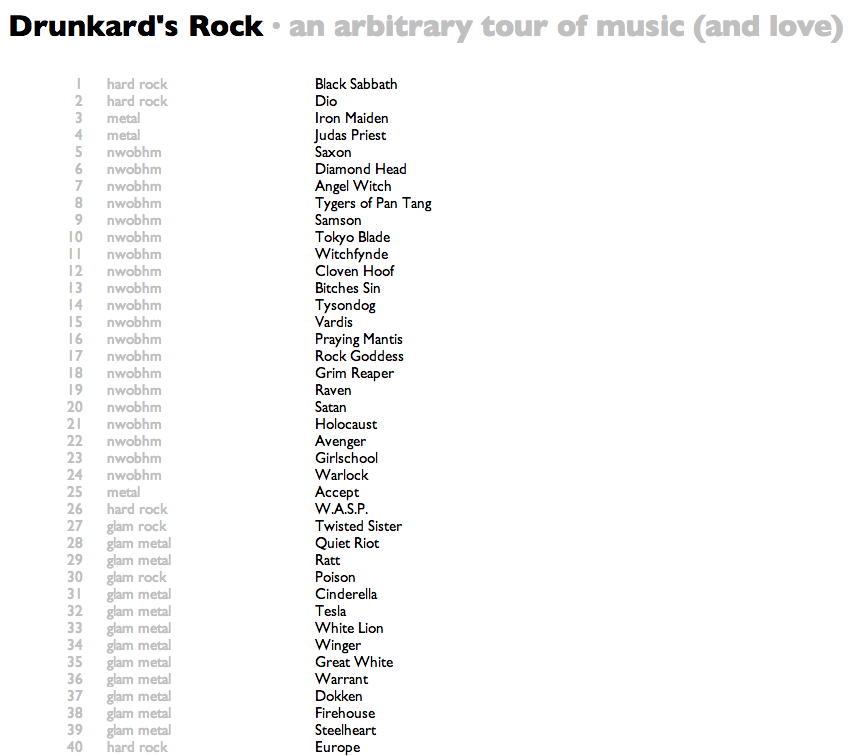
¶ 4 Entries for an Audible Glossary · 2 May 2014 listen/tech
Every Noise at Once is a readability-adjusted scatter-plot of musical genres. The music moves from high density on the left to high bounciness on the right, and from high mechanism at the top to high organism at the bottom. Although I do have more words to explain what each of these ideas means, it's maybe better to just hear how the qualities manifest themselves in actual music.
So here are four data-generated sampler playlists of songs that demonstrate the extreme values of these two analytical dimensions:
So here are four data-generated sampler playlists of songs that demonstrate the extreme values of these two analytical dimensions:
Density | Bounciness |
Mechanism | Organism |
¶ Voices on the Radio, Whispers in Your Ear · 1 May 2014 listen/tech
WBUR ran a story today about The Echo Nest, including such important topics as Massive Amounts of Data and Viking Metal.
Music Freaks At Somervilles The Echo Nest Fuel The Engine Under Spotifys Hood
Meanwhile, one thing I did with our Massive Amounts of Data today is make some playlists of quiet, calm music from unlikely sources. Here, for example, are three sets from artists normally known for metal, electronica or hip hop.
Music Freaks At Somervilles The Echo Nest Fuel The Engine Under Spotifys Hood
Meanwhile, one thing I did with our Massive Amounts of Data today is make some playlists of quiet, calm music from unlikely sources. Here, for example, are three sets from artists normally known for metal, electronica or hip hop.
I gave a talk on music discovery and genre-mapping at the 2014 EMP Pop Conference in Seattle last weekend. My co-panelist Michaelangelo Matos had the presence of mind to record the audio from this, and mostly the talk was just audio. Actually, I was wearing some really excellent silver pants, but while talking I was behind a podium, so you aren't missing that much. The map I describe at the end is Every Noise at Once.
So if you're curious, the talk is 16:32 long, and you can listen here:
Every Noise at Once @ EMP Pop 2014
And, although this will not make a lot of sense until after you listen to the talk, the playlist I narrate is also available here:
So if you're curious, the talk is 16:32 long, and you can listen here:
Every Noise at Once @ EMP Pop 2014
And, although this will not make a lot of sense until after you listen to the talk, the playlist I narrate is also available here: